Hyperspectral-Based Classification of Managed Permanent Grassland with Multilayer Perceptrons: Influence of Spectral Band Count and Spectral Regions on Model Performance
AGRICULTURE-BASEL(2022)
摘要
Detailed knowledge of botanical composition is a key factor for the sustainable and site-specific management of permanent grassland and facilitates an improvement in the performance and efficiency of livestock feeding. Spectral-based data acquisition combined with machine learning has the potential to classify species groups and plant parts in permanent grassland with high accuracy. However, a disadvantage of this method is the fact that hyperspectral sensors with a wide spectral range and fine spectral and high spatial resolution are costly and create large amounts of data. Therefore, the question arises as to whether these parameters are necessary for accurate grassland classification. Thus, the use of sensors with lower spectral and spatial resolution and correspondingly lower data processing requirements could be a conceivable approach. Therefore, we investigated the classification performance with reduced predictor sets formed by different approaches in permanent grassland. For pixel-based classification, a cross-validated mean accuracy of 86.1% was reached using a multilayer perceptron (MLP) including all 191 available predictors, i.e., spectral bands. Using only 48 high-performing predictors, an accuracy of 80% could still be achieved. In particular, the spectral regions of 954 nm to 956 nm, 684 nm to 744 nm and 442 nm to 444 nm contributed most to the classification performance. These results provide a promising basis for future data acquisition and the analysis of grassland vegetation.
更多查看译文
关键词
grass, herb, legume, machine learning, hyperspectral imaging, data reduction
AI 理解论文
溯源树
样例
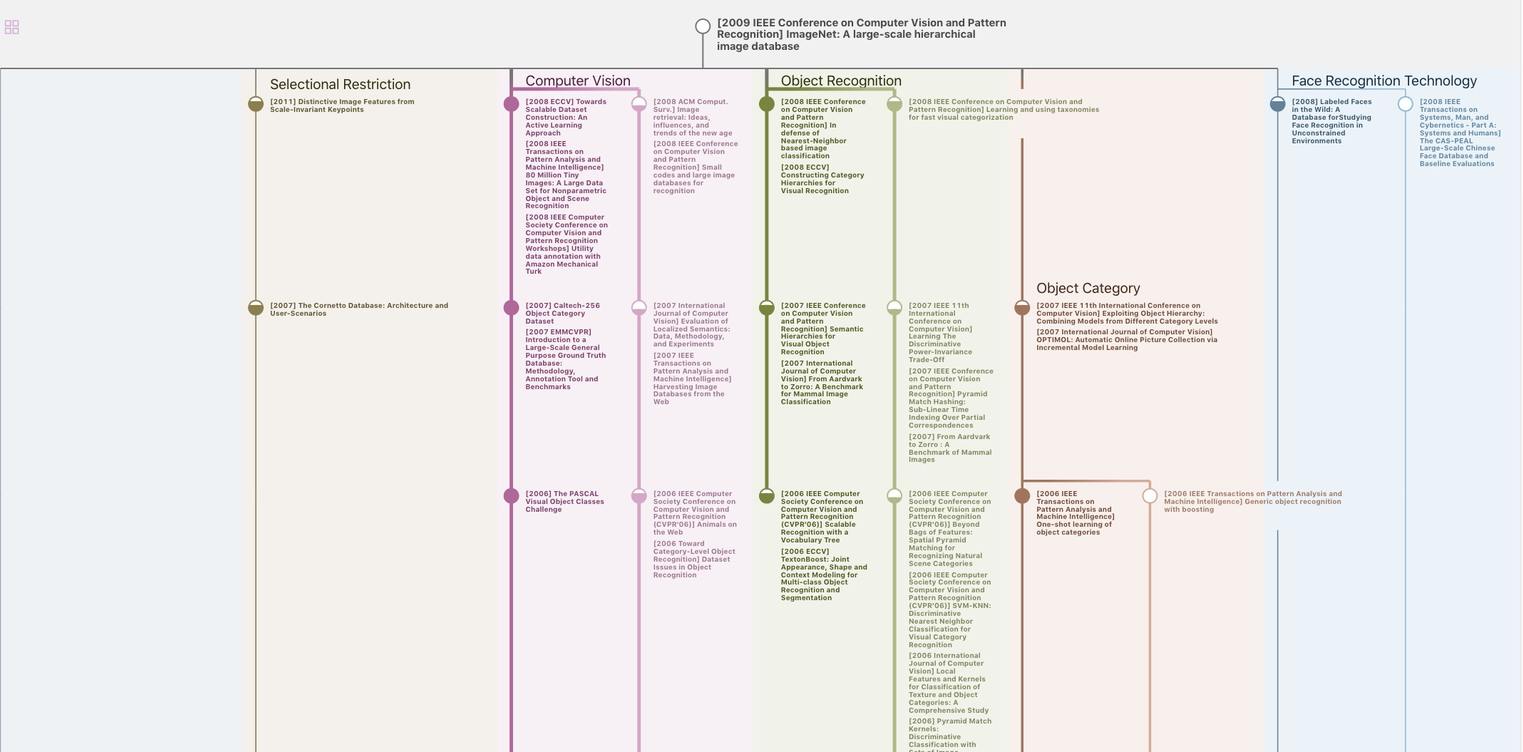
生成溯源树,研究论文发展脉络
Chat Paper
正在生成论文摘要