Improved Trend-Aware Postprocessing of GCM Seasonal Precipitation Forecasts
JOURNAL OF HYDROMETEOROLOGY(2022)
摘要
Climate trends have been observed over the recent decades in many parts of the world, but current global climate models (GCMs) for seasonal climate forecasting often fail to capture these trends. As a result, model forecasts may be biased above or below the trendline. In our previous research, we developed a trend-aware forecast postprocessing method to overcome this problem. The method was demonstrated to be effective for embedding observed trends into seasonal temperature forecasts. In this study, we further develop the method for postprocessing GCM seasonal precipitation forecasts. We introduce new formulation and evaluation features to cater for special characteristics of precipitation amounts, such as having a zero lower bound and highly positive skewness. We apply the improved method to calibrate ECMWF SEAS5 forecasts of seasonal precipitation for Australia. Our evaluation shows that the calibrated forecasts reproduce observed trends over the hindcast period of 36 years. In some regions where observed trends are statistically significant, forecast skill is greatly improved by embedding trends into the forecasts. In most regions, the calibrated forecasts outperform the raw forecasts in terms of bias, skill, and reliability. Wider applications of the new trend-aware postprocessing method are expected to boost user confidence in seasonal precipitation forecasts.
更多查看译文
关键词
Bayesian methods, Forecast verification/skill, Hindcasts, Seasonal forecasting, Postprocessing, Trends
AI 理解论文
溯源树
样例
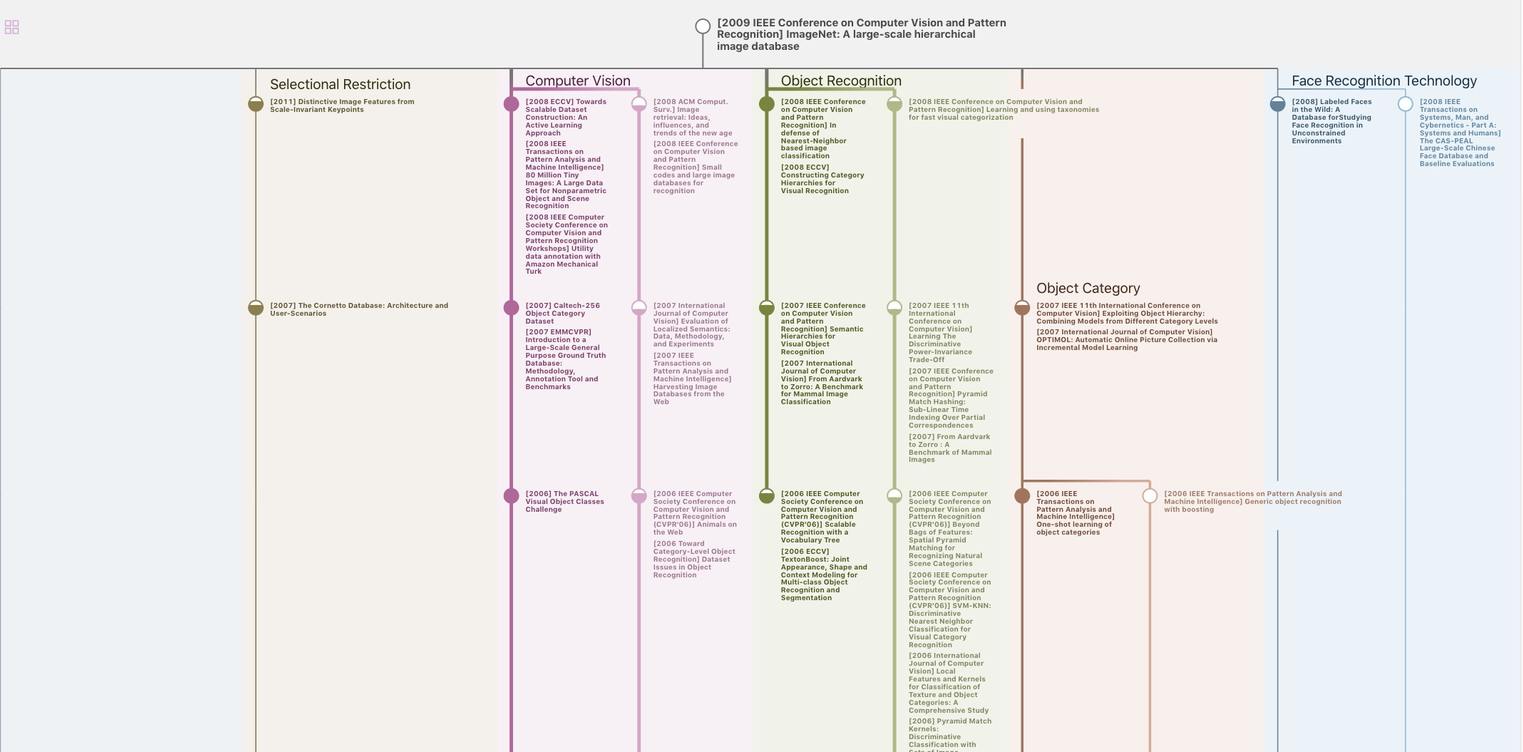
生成溯源树,研究论文发展脉络
Chat Paper
正在生成论文摘要