Machine learning for effective spacecraft operation: Operating INTEGRAL through dynamic radiation environments
Advances in Space Research(2022)
摘要
INTEGRAL (INTErnational Gamma-Ray Astrophysics Laboratory) is an astronomical observatory of the European Space Agency, responsible for many significant scientific discoveries in the last few decades. It orbits Earth since 2002 in a highly elliptical orbit, passing through the Van Allen belts – areas with high-energy ionized particles that can damage the spacecraft’s on-board equipment. An essential part of mission planning and operation of INTEGRAL is thus the prediction of its radiation belts entry and exit times. We propose a novel compact representation of the data and evaluate its potential using several machine learning methods. The experimental validation identifies gradient boosted trees with quantile loss as the best performing method. By using our approach, INTEGRAL can perform 2 additional hours (on average) of scientific measurements per orbit (with adjustment for uncertainty at the 95th percentile). This approach protects INTEGRAL from damages and improves its scientific return at the same time. It can be easily extended and applied to other spacecraft with similar orbits.
更多查看译文
关键词
Spacecraft operation,INTEGRAL,Mission planning,Van Allen belts,Machine learning,Ensemble methods,Gradient boosting,Random forests,Neural networks
AI 理解论文
溯源树
样例
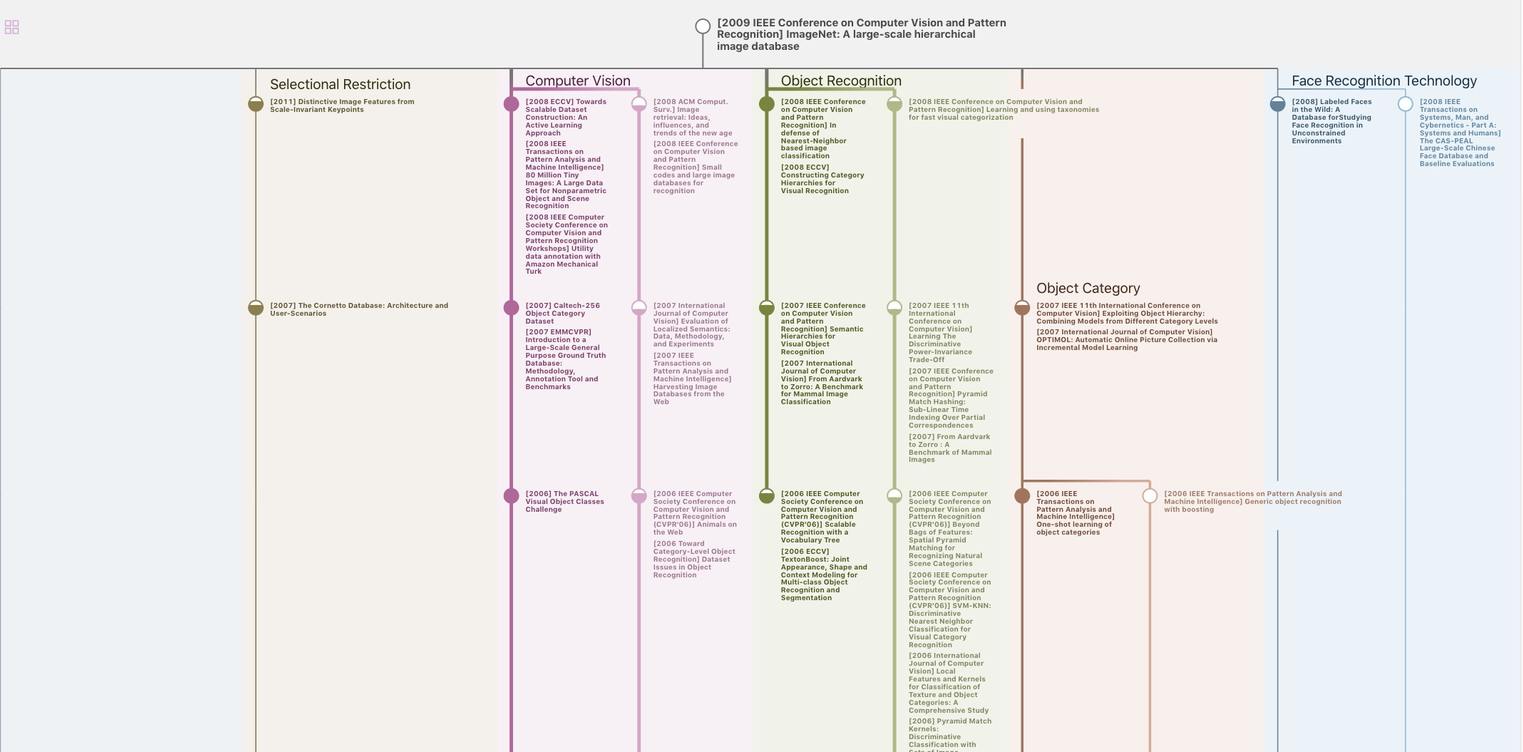
生成溯源树,研究论文发展脉络
Chat Paper
正在生成论文摘要