A Unified Strategy for Multilingual Grammatical Error Correction with Pre-trained Cross-Lingual Language Model.
European Conference on Artificial Intelligence(2022)
摘要
Synthetic data construction of Grammatical Error Correction (GEC) for non-English languages relies heavily on human-designed and language-specific rules, which produce limited error-corrected patterns. In this paper, we propose a generic and language-independent strategy for multilingual GEC, which can train a GEC system effectively for a new non-English language with only two easy-to-access resources: 1) a pretrained cross-lingual language model (PXLM) and 2) parallel translation data between English and the language. Our approach creates diverse parallel GEC data without any language-specific operations by taking the non-autoregressive translation generated by PXLM and the gold translation as error-corrected sentence pairs. Then, we reuse PXLM to initialize the GEC model and pretrain it with the synthetic data generated by itself, which yields further improvement. We evaluate our approach on three public benchmarks of GEC in different languages. It achieves the state-of-the-art results on the NLPCC 2018 Task 2 dataset (Chinese) and obtains competitive performance on Falko-Merlin (German) and RULEC-GEC (Russian). Further analysis demonstrates that our data construction method is complementary to rule-based approaches.
更多查看译文
关键词
Natural Language Processing: Applications,Natural Language Processing: Language Generation
AI 理解论文
溯源树
样例
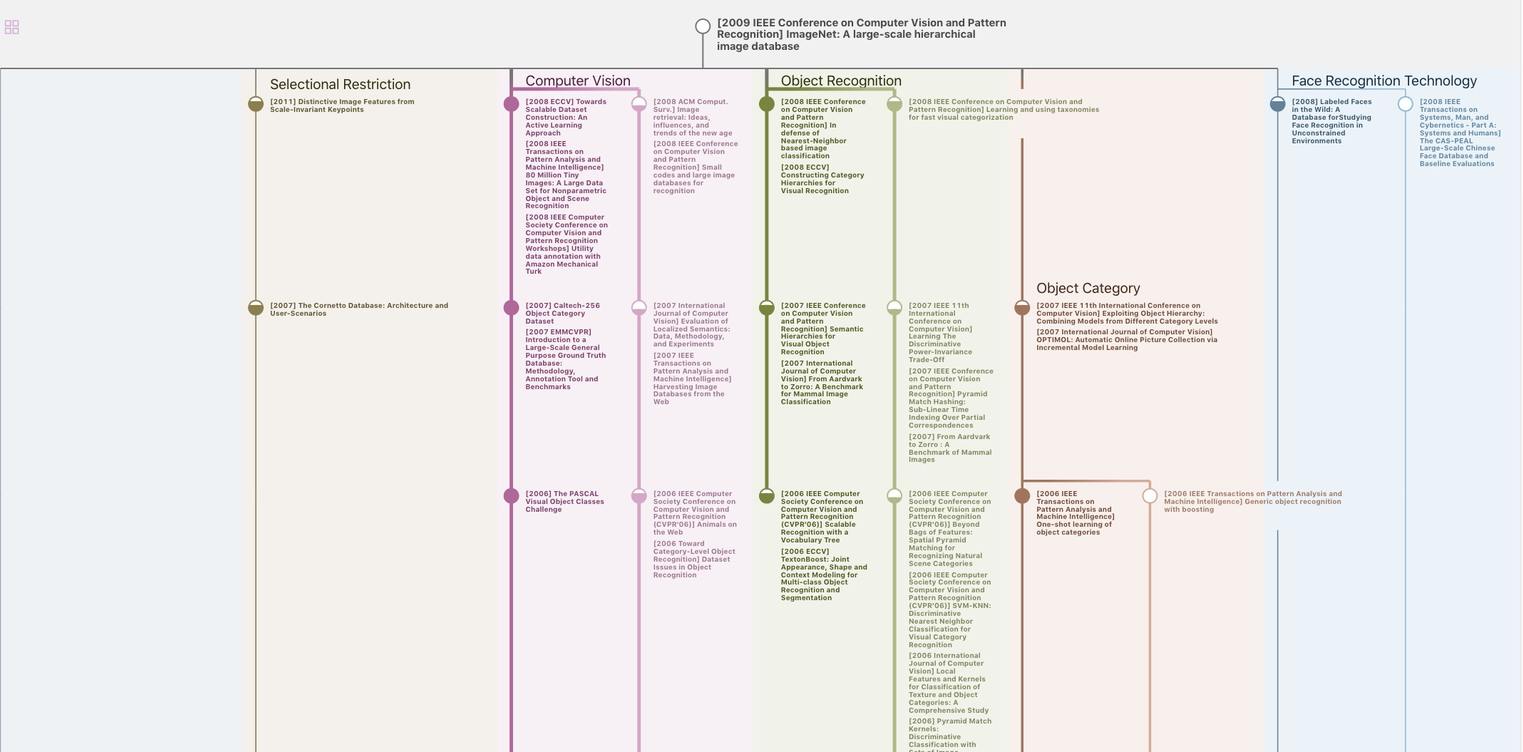
生成溯源树,研究论文发展脉络
Chat Paper
正在生成论文摘要