Deep learning for electromagnetically induced transparency (EIT) metasurface optimization design
JOURNAL OF PHYSICS D-APPLIED PHYSICS(2022)
摘要
In order to accelerate the design process of electromagnetically induced transparency (EIT) metasurface, a deep learning-based EIT metasurface design method is proposed, where the spectral profile of EIT metasurface can be predicted by the forward prediction process, and the EIT metasurface geometry parameters based on the target spectral profile can be obtained by the inverse design process. In the inverse design process, a cascaded convolutional neural network (CNN) consisting of one-dimensional convolutional layer, a batch normalization layer, a pooling layer and an exponential linear unit (ELU) activation function is employed. Each CNN is designed to achieve feature extraction for spectra. The inverse network achieves low mean square errors (MSE), with MSE of 0.011 on the validation sets. After training, the model can more accurately predict the parameters with error of 0.3 mu m. This method is more efficient and saves computing resources, allowing designers to focus on the target spectra. More importantly, it can be extended to the design of arbitrary metasurface.
更多查看译文
关键词
metasurface, electromagnetically induced transparency effect (EIT), deep learning, inverse design
AI 理解论文
溯源树
样例
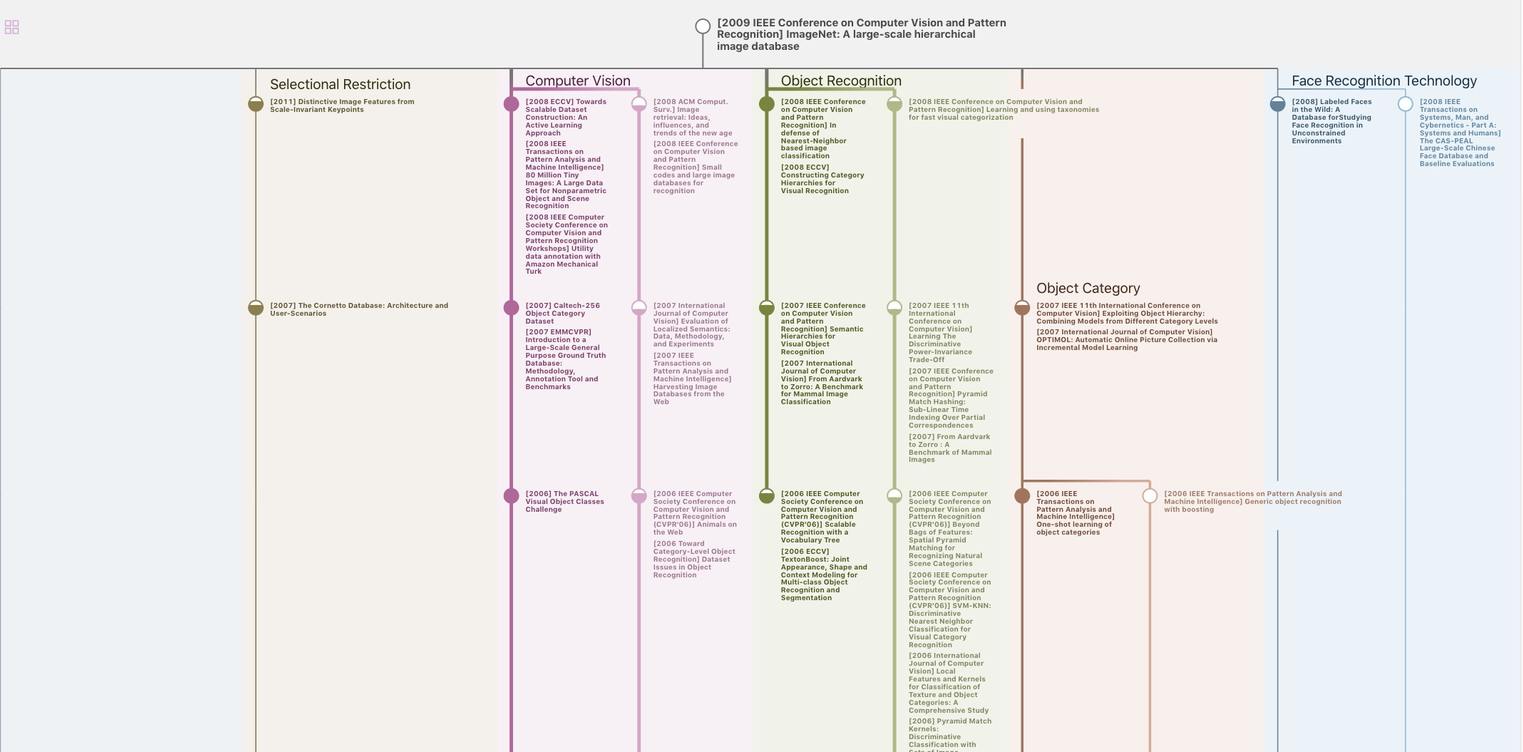
生成溯源树,研究论文发展脉络
Chat Paper
正在生成论文摘要