Classification of Low-Grade and High-Grade Glioma from MR Brain Images Using Multiple-Instance Learning with Combined Feature Set
VIETNAM JOURNAL OF COMPUTER SCIENCE(2022)
摘要
Fully automatic brain image classification of MR brain images is of great importance for research and clinical studies, since the precise detection may lead to a better treatment. In this work, an efficient method based on Multiple-Instance Learning (MIL) is proposed for the automatic classification of low-grade and high-grade MR brain tumor images. The main advantage of MIL-based approach over other classification methods is that MIL considers an image as a group of instances rather than a single instance, thus facilitating an effective learning process. The mi-Graph-based MIL approach is proposed for this classification. Two different implementations of MIL-based classification, viz. Patch-based MIL (PBMIL) and Superpixel-based MIL (SPBMIL), are made in this study. The combined feature set of LBP, SIFT and FD is used for the classification. The accuracies of low-grade-high-grade tumor image classification algorithm using SPBMIL method performed on T-1, T-2 and FLAIR images read 99.2765%, 99.4195% and 99.2265%, respectively. The error rate of the proposed classification system was noted to be insignificant and hence this automated classification system could be used for the classification of images with different pathological conditions, types and disease statuses.
更多查看译文
关键词
Low-grade-high-grade tumor classification, Multiple-Instance Learning (MIL), mi-Graph, Patch-based MIL (PBMIL), Superpixel-based MIL (SPBMIL)
AI 理解论文
溯源树
样例
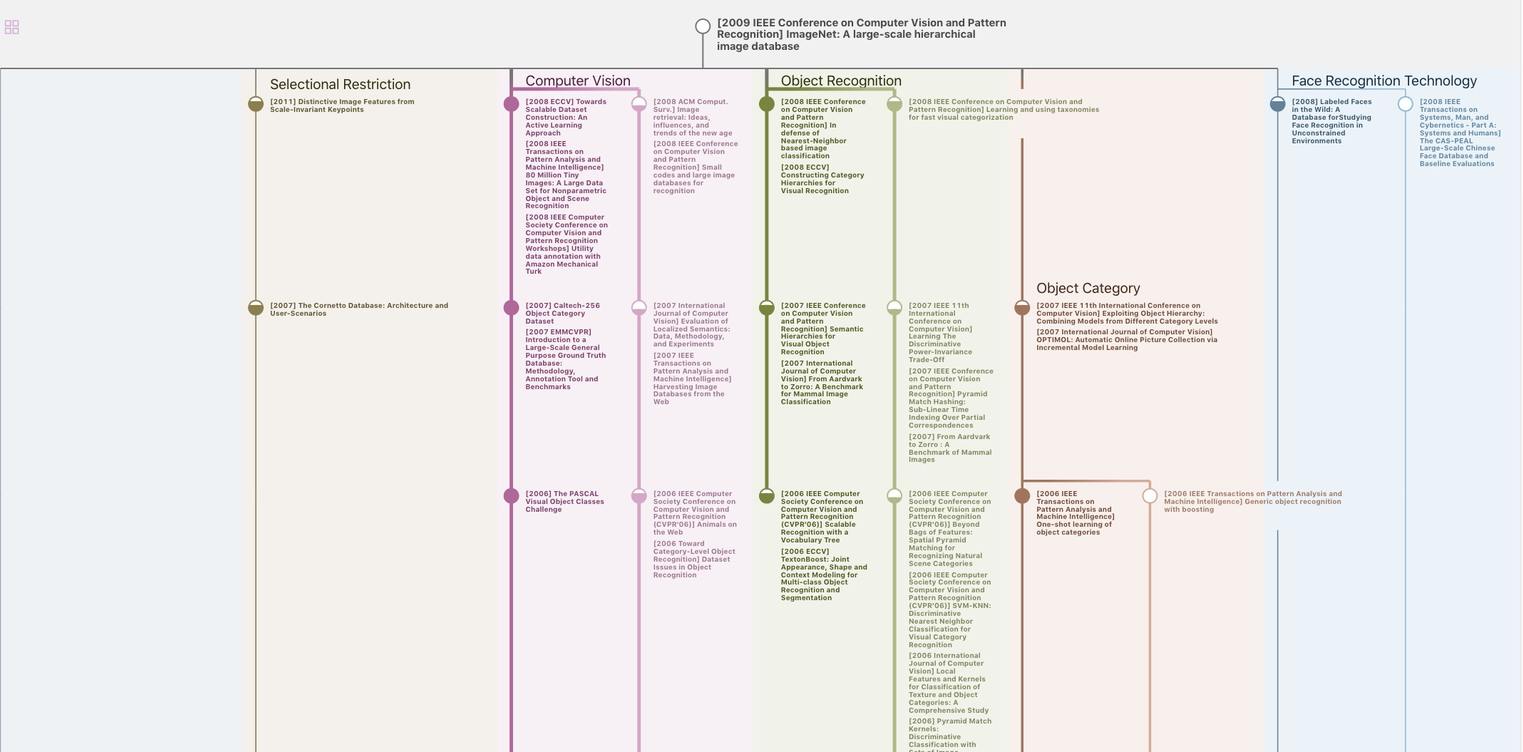
生成溯源树,研究论文发展脉络
Chat Paper
正在生成论文摘要