Optimized Deep Learning Model for Fire Semantic Segmentation
CMC-COMPUTERS MATERIALS & CONTINUA(2022)
摘要
Recent convolutional neural networks (CNNs) based deep learning has significantly promoted fire detection. Existing fire detection methods can efficiently recognize and locate the fire. However, the accurate flame boundary and shape information is hard to obtain by them, which makes it difficult to conduct automated fire region analysis, prediction, and early warning. To this end, we propose a fire semantic segmentation method based on Global Position Guidance (GPG) and Multi-path explicit Edge information Interaction (MEI). Specifically, to solve the problem of local segmentation errors in low-level feature space, a top-down global position guidance module is used to restrain the offset of low-level features. Besides, an MEI module is proposed to explicitly extract and utilize the edge information to refine the coarse fire segmentation results. We compare the proposed method with existing advanced semantic segmentation and salient object detection methods. Experimental results demonstrate that the proposed method achieves 94.1%, 93.6%, 94.6%, 95.3%, and 95.9% Intersection over Union (IoU) on five test sets respectively which outperforms the suboptimal method by a large margin. In addition, in terms of accuracy, our approach also achieves the best score.
更多查看译文
关键词
Fire semantic segmentation, local segmentation errors, global posi-tion guidance, multi-path explicit edge information interaction, feature fusion
AI 理解论文
溯源树
样例
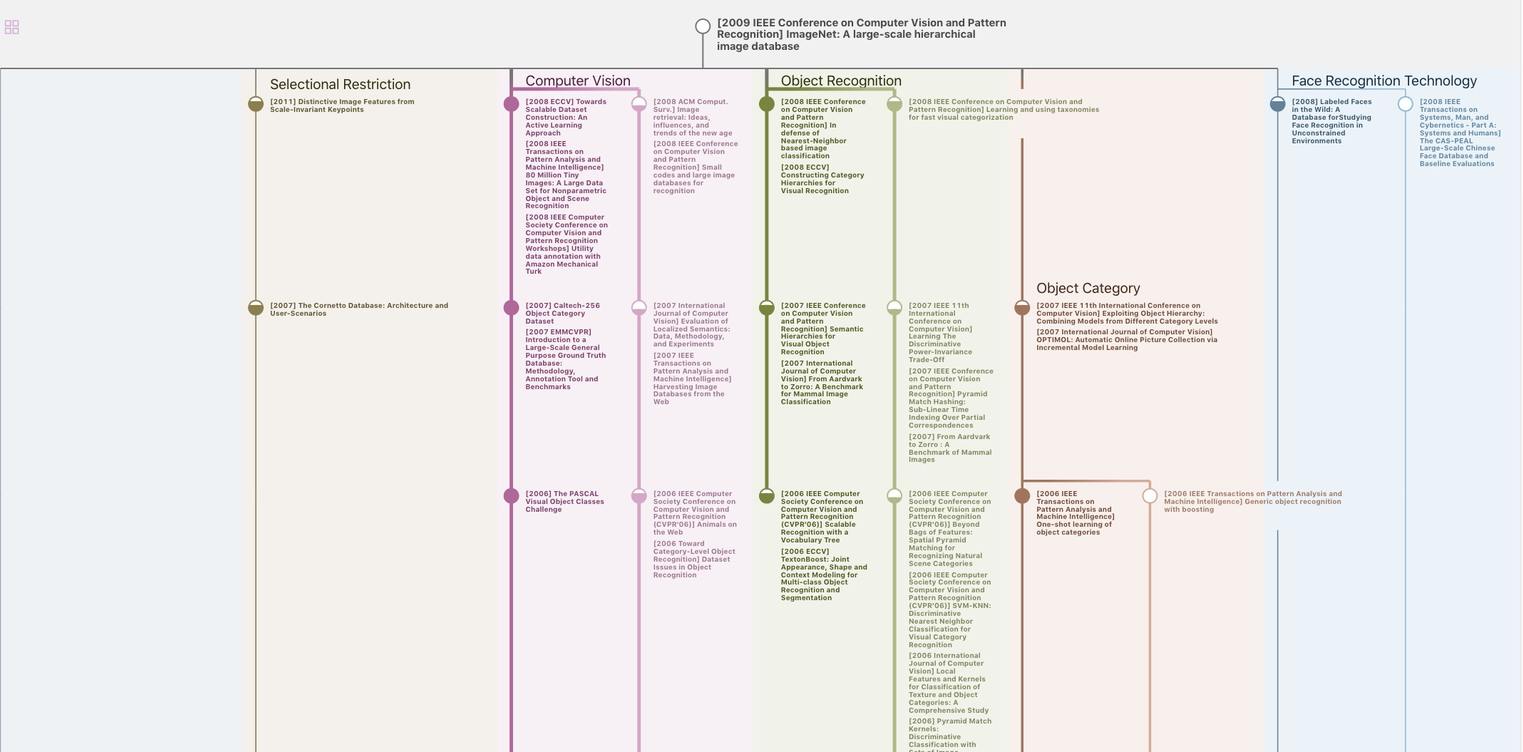
生成溯源树,研究论文发展脉络
Chat Paper
正在生成论文摘要