Finite element-based machine learning approach for optimization of process parameters to produce silicon carbide ceramic complex parts
Ceramics International(2022)
摘要
Design and fabrication of silicon carbide ceramic complex parts introduce considerable difficulties during injection molding. Due to the great importance in processing optimization, an accurate prediction on the stress and displacement is required to obtain the desired final product. In this paper, a conceptual framework on combination of finite element method (FEM) and machine learning (ML) method was developed to optimize the injection molding process, which can be used to manufacture large-aperture silicon carbide mirror. The distribution characteristics of temperature field and stress field were extracted from FEM simulation to understand the injection molding process and construct database for ML modeling. To select the most appropriate model, the predictive performance of three ML models were estimated, including generalized regression neural network (GRNN), back propagation neural network (BPNN) and extreme learning machine (ELM). The results show that the developed ELM model exhibits exceptional predictive performance and can be utilized to predict the stress and displacement of the green body. This work allows us to obtain reasonable technique parameters with particular attention to the loading speed and provides some fundamental guidance for the fabrication of lightweight SiC ceramic optical mirror.
更多查看译文
关键词
Finite element analysis,Machine learning,Gelcasting process,Silicon carbide,Process optimization
AI 理解论文
溯源树
样例
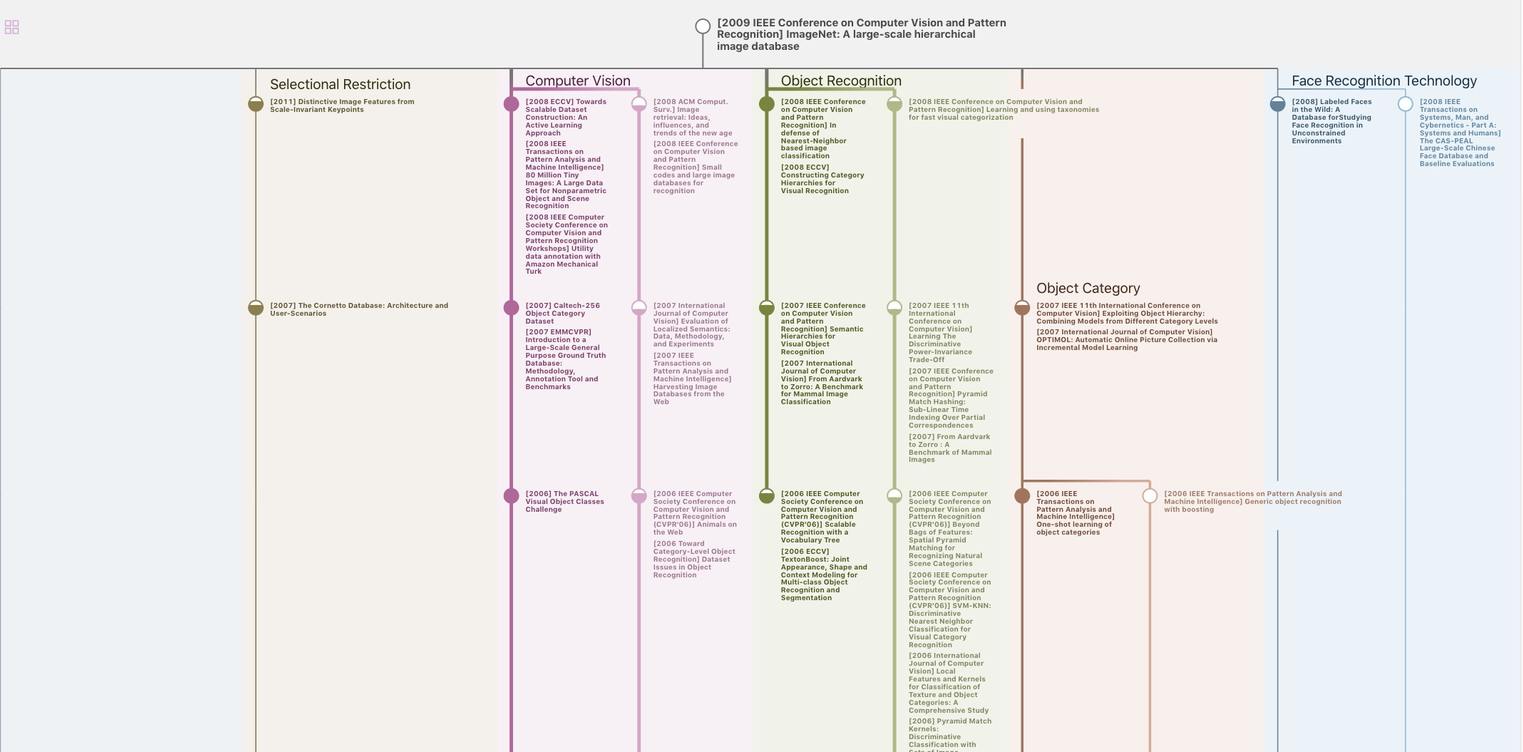
生成溯源树,研究论文发展脉络
Chat Paper
正在生成论文摘要