Measurement error-filtered machine learning in digital soil mapping
Spatial Statistics(2022)
摘要
This paper presents a two-stage maximum likelihood framework to deal with measurement errors in digital soil mapping (DSM) when using a machine learning (ML) model. The framework is implemented with random forest and projection pursuit regression to illustrate two different areas of machine learning, i.e. ensemble learning with trees and feature-learning. In our proposed framework, a measurement error variance (MEV) is incorporated as a weight in the log-likelihood function so that measurements with a larger MEV receive less weight when a ML model is calibrated. We evaluate the performance of the error-filtered ML models with an error-filtered regression kriging model, in a comprehensive simulation study and in a real-world case study of Namibian data. From the results we show that prediction accuracy can be increased by using our proposed framework, especially when the MEVs are large and heterogeneous.
更多查看译文
关键词
Digital soil mapping,Machine learning,Measurement error,Maximum likelihood,REML,E-BLUP
AI 理解论文
溯源树
样例
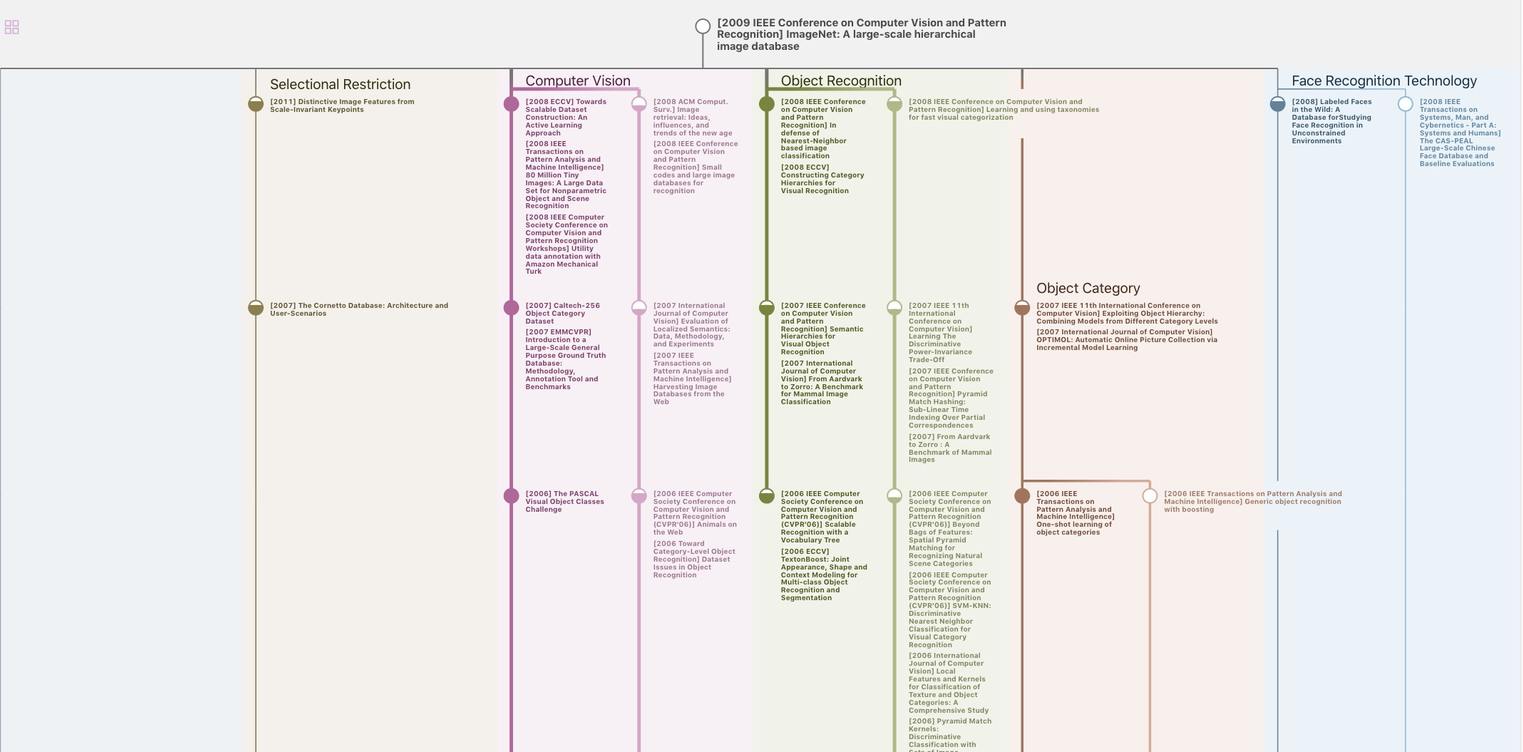
生成溯源树,研究论文发展脉络
Chat Paper
正在生成论文摘要