Rumor detection on social media using hierarchically aggregated feature via graph neural networks
APPLIED INTELLIGENCE(2022)
摘要
In the era of the Internet and big data, online social media platforms have been developing rapidly, which accelerate rumors circulation. Rumor detection on social media is a worldwide challenging task due to rumor’s feature of high speed, fragmental information and extensive range. Most existing approaches identify rumors based on single-layered hybrid features like word features, sentiment features and user characteristics, or multimodal features like the combination of text features and image features. Some researchers adopted the hierarchical structure, but they neither used rumor propagation nor made full use of its retweet posts. In this paper, we propose a novel model for rumor detection based on Graph Neural Networks (GNN), named Hierarchically Aggregated Graph Neural Networks (HAGNN). This task focuses on capturing different granularities of high-level representations of text content and fusing the rumor propagation structure. It applies a Graph Convolutional Network (GCN) with a graph of rumor propagation to learn the text-granularity representations with the spreading of events. A GNN model with a document graph is employed to update aggregated features of both word and text granularity, it helps to form final representations of events to detect rumors. Experiments on two real-world datasets demonstrate the superiority of the proposed method over the baseline methods. Our model achieves the accuracy of 95.7 % and 88.2 % on the Weibo dataset Ma et al. 2017 and the CED dataset Song et al. IEEE Trans Knowl Data Eng 33(8):3035–3047, 2019 respectively.
更多查看译文
关键词
Rumor detection,Graph neural networks,Hierarchical aggregation,Rumor propagation
AI 理解论文
溯源树
样例
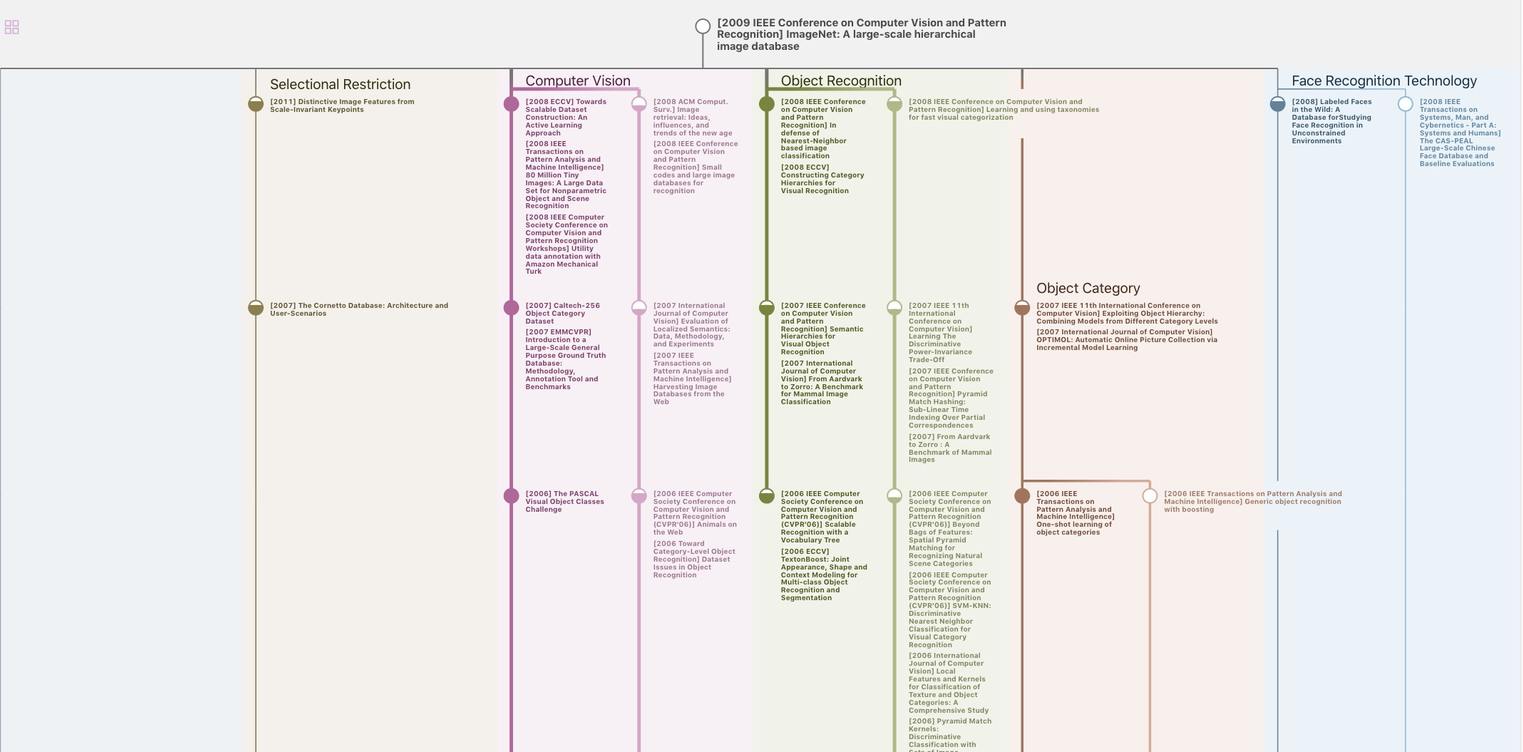
生成溯源树,研究论文发展脉络
Chat Paper
正在生成论文摘要