Ensemble Deep Learning for Sustainable Multimodal UAV Classification
IEEE TRANSACTIONS ON INTELLIGENT TRANSPORTATION SYSTEMS(2023)
摘要
Unmanned aerial vehicles (UAVs) have increasingly shown to be useful in civilian applications (such as agriculture, public safety, surveillance) and mission critical military applications. Despite the growth in popularity and applications, UAVs have also been used for malicious purposes. In such instances, their timely detection and identification has garnished rising interest from government, industry and academia. While much work has been done for detecting UAVs, there still exist limitations related to the impact of extreme environmental conditions and big dataset requirements. This paper proposes a novel ensemble deep learning framework that has hybrid synthetic and deep features to detect unauthorized or malicious UAVs by using acoustic, image/video and wireless radio frequency (RF) signals for robust UAV detection and classification. We present the performance evaluation of the proposed approach using numerical results obtained from experiments using acoustic, image/video and wireless RF signals. The proposed approach outperforms the existing related approaches for detecting malicious UAVs.
更多查看译文
关键词
Feature extraction, Convolutional neural networks, Convolution, Hidden Markov models, Acoustics, Deep learning, Autonomous aerial vehicles, Ensemble deep learning, multi-modal UAV classification, UAV detection, machine learning, CNN
AI 理解论文
溯源树
样例
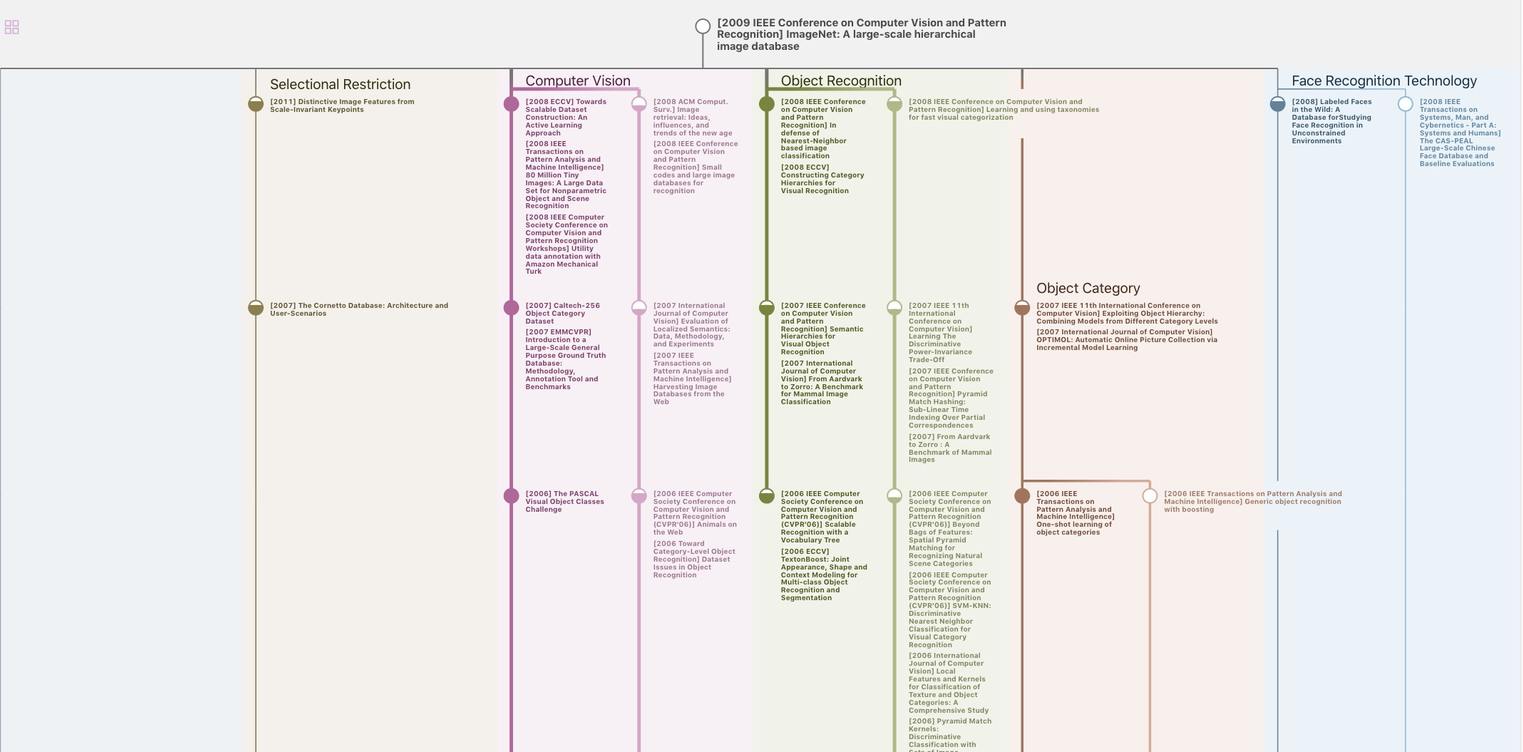
生成溯源树,研究论文发展脉络
Chat Paper
正在生成论文摘要