Joint Collaborative Big Spectrum Data Sensing and Reinforcement Learning Based Dynamic Spectrum Access for Cognitive Internet of Vehicles
IEEE TRANSACTIONS ON INTELLIGENT TRANSPORTATION SYSTEMS(2022)
摘要
Cognitive Internet of Vehicles (CIoV) is an intelligent vehicle network envisioned to opportunistically access spectrum licensed to primary users (PUs) on the premise of not interrupting their normal communications. Dynamic spectrum access enables the CIoV to choose the best possible spectrum for communications based on the outcomes of spectrum data sensing, which can improve the spectrum access performance effectively. In this paper, we enable the CIoV to adapt to various spectrum states through: a) a collaborative big spectrum data sensing scheme to sense a massive amount of spectrum data; and b) a reinforcement learning (RL) based dynamic spectrum access scheme to optimize spectrum selection strategies. Q-learning, which is a popular RL approach, is proposed for underlay, overlay, and collaborative spectrum access modes to allocate spectrum resources to the CIoV intelligently. The Q-learning models, which include the spectrum state vector, the action vector of CIoV, and the spectrum access reward received in different spectrum situations, are defined for the spectrum access modes. A Q-learning based spectrum access algorithm is proposed to improve the communication performance of the CIoV in different spectrum access modes. Simulation results indicate that the collaborative spectrum access mode can achieve higher average throughput, lower interference power and lower communication outage compared with the underlay and overlay spectrum access modes.
更多查看译文
关键词
Sensors,Collaboration,Interference,Q-learning,Vehicle dynamics,Dynamic spectrum access,Fading channels,CIoV,reinforcement learning,big spectrum data,dynamic spectrum access,Q-learning
AI 理解论文
溯源树
样例
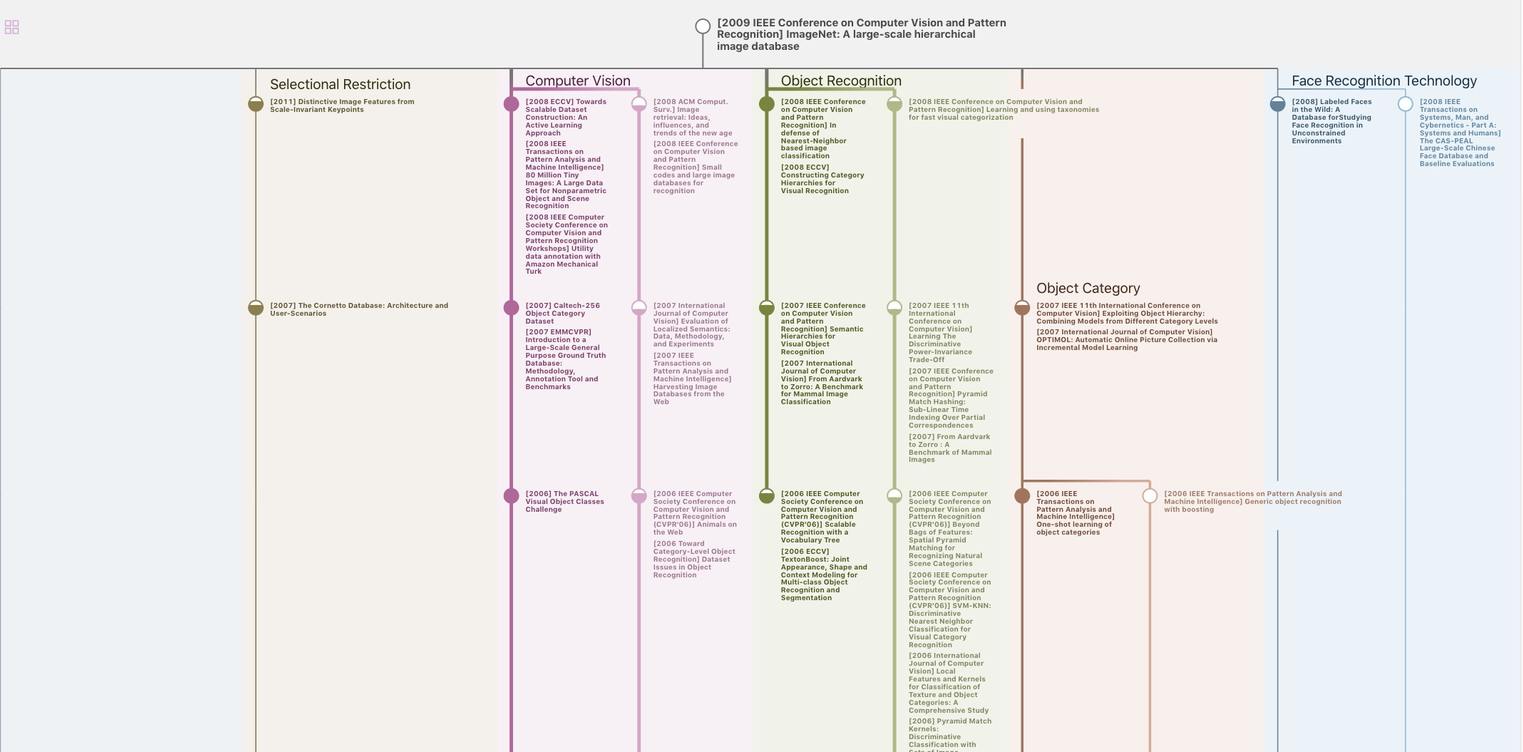
生成溯源树,研究论文发展脉络
Chat Paper
正在生成论文摘要