Joint Alignment and Compactness Learning for Multi-Source Unsupervised Domain Adaptation
FOURTEENTH INTERNATIONAL CONFERENCE ON MACHINE VISION (ICMV 2021)(2022)
摘要
Multi-source unsupervised domain adaptation (MUDA) has received increasing attention that leverages the knowledge from multiple relevant source domains with different distributions to improve the learning performance of the target domain. The most common approach for MUDA is to perform pairwise distribution alignment between the target and each source domain. However,existing methods usually treat each source domain identically in source-source and source-target alignment, which ignores the difference of multiple source domains and may lead to imperfect alignment. In addition, these methods often neglect the samples near the classification boundaries during adaptation process, resulting in misalignment of these samples. In this paper, we propose a new framework for MUDA, named Joint Alignment and Compactness Learning (JACL). We design an adaptive weighting network to automatically adjust the importance of marginal and conditional distribution alignment, and such weights are adopted to adaptively align each pair of source-target domains. We further propose to learn intra-class compact features for some target samples that lie in boundaries to reduce the domain shift. Extensive experiments demonstrate that our method can achieve remarkable results in three datasets (Digit-five, Office-31, and Office-Home) compared to recently strong baselines.
更多查看译文
关键词
Domain adaptation, transfer learning, multi-source
AI 理解论文
溯源树
样例
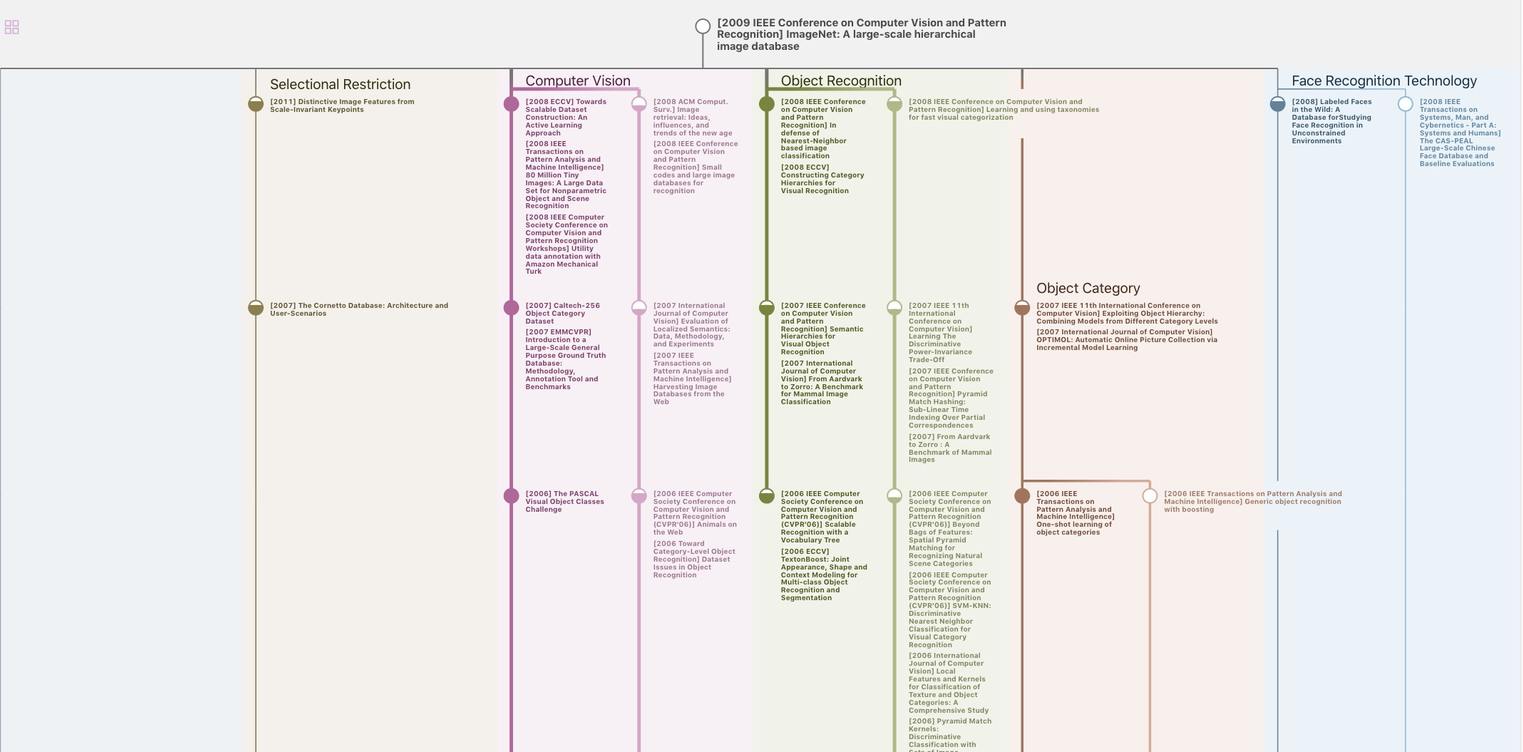
生成溯源树,研究论文发展脉络
Chat Paper
正在生成论文摘要