Digital Mapping of Soil Organic Carbon with Machine Learning in Dryland of Northeast and North Plain China
REMOTE SENSING(2022)
摘要
Due to the importance of soil organic carbon (SOC) in supporting ecosystem services, accurate SOC assessment is vital for scientific research and decision making. However, most previous studies focused on single soil depth, leading to a poor understanding of SOC in multiple depths. To better understand the spatial distribution pattern of SOC in Northeast and North China Plain, we compared three machine learning algorithms (i.e., Cubist, Extreme Gradient Boosting (XGBoost) and Random Forest (RF)) within the digital soil mapping framework. A total of 386 sampling sites (1584 samples) following specific criteria covering all dryland districts and counties and soil types in four depths (i.e., 0-10, 10-20, 20-30 and 30-40 cm) were collected in 2017. After feature selection from 249 environmental covariates by the Genetic Algorithm, 29 variables were used to fit models. The results showed SOC increased from southern to northern regions in the spatial scale and decreased with soil depths. From the result of independent verification (validation dataset: 80 sampling sites), RF (R-2: 0.58, 0.71, 0.73, 0.74 and RMSE: 3.49, 3.49, 2.95, 2.80 g kg(-1) in four depths) performed better than Cubist (R-2: 0.46, 0.63, 0.67, 0.71 and RMSE: 3.83, 3.60, 3.03, 2.72 g kg(-1)) and XGBoost (R-2: 0.53, 0.67, 0.70, 0.71 and RMSE: 3.60, 3.60, 3.00, 2.83 g kg(-1)) in terms of prediction accuracy and robustness. Soil, parent material and organism were the most important covariates in SOC prediction. This study provides the up-to-date spatial distribution of dryland SOC in Northeast and North China Plain, which is of great value for evaluating dynamics of soil quality after long-term cultivation.
更多查看译文
关键词
soil organic carbon, Northeast and North Plain China, model comparison, spatial distribution, controlling factor
AI 理解论文
溯源树
样例
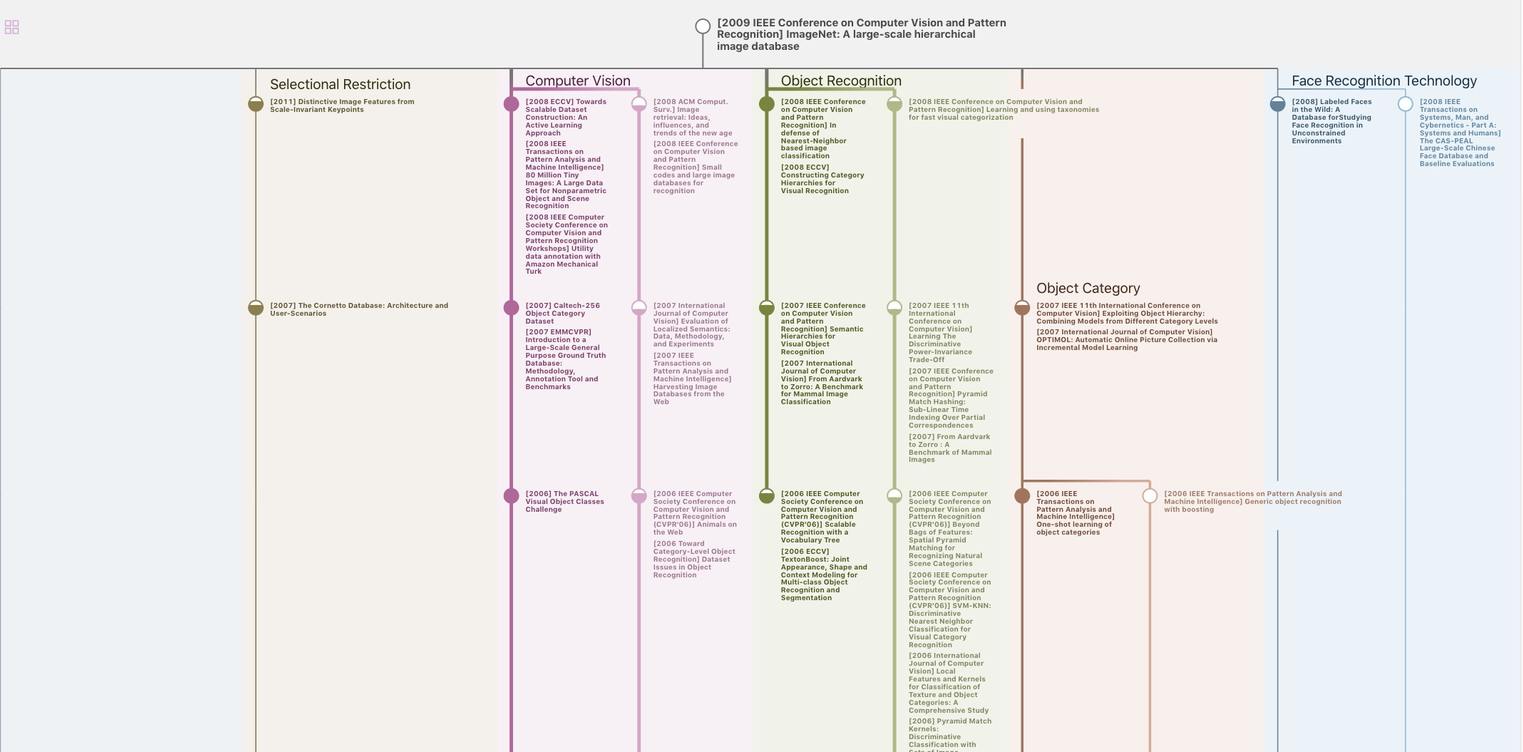
生成溯源树,研究论文发展脉络
Chat Paper
正在生成论文摘要