Predicting Topographic Effect Multipliers in Complex Terrain With Shallow Neural Networks
FRONTIERS IN BUILT ENVIRONMENT(2022)
摘要
This study applies computationally efficient shallow neural networks to predict topographic effect multipliers directly from digital elevation data obtained from complex terrain, such as mountainous areas. Data were obtained from boundary layer wind tunnel (BLWT) modeling of surface wind flow over six regions in mainland Puerto Rico and its municipal islands. The results demonstrate an improvement over linear regression models, even for computationally efficient low neuron count and single hidden layer models. The paper proposes the development of a global BLWT data atlas to inform development of methods to predict topographic wind speedup for a diverse range of topography and surface roughness conditions. It also identifies knowledge gaps that could prevent standardization of data collected from different BLWT experimental designs.
更多查看译文
关键词
complex topography, neural network, boundary layer wind tunnel, complex terrain, wind speedup
AI 理解论文
溯源树
样例
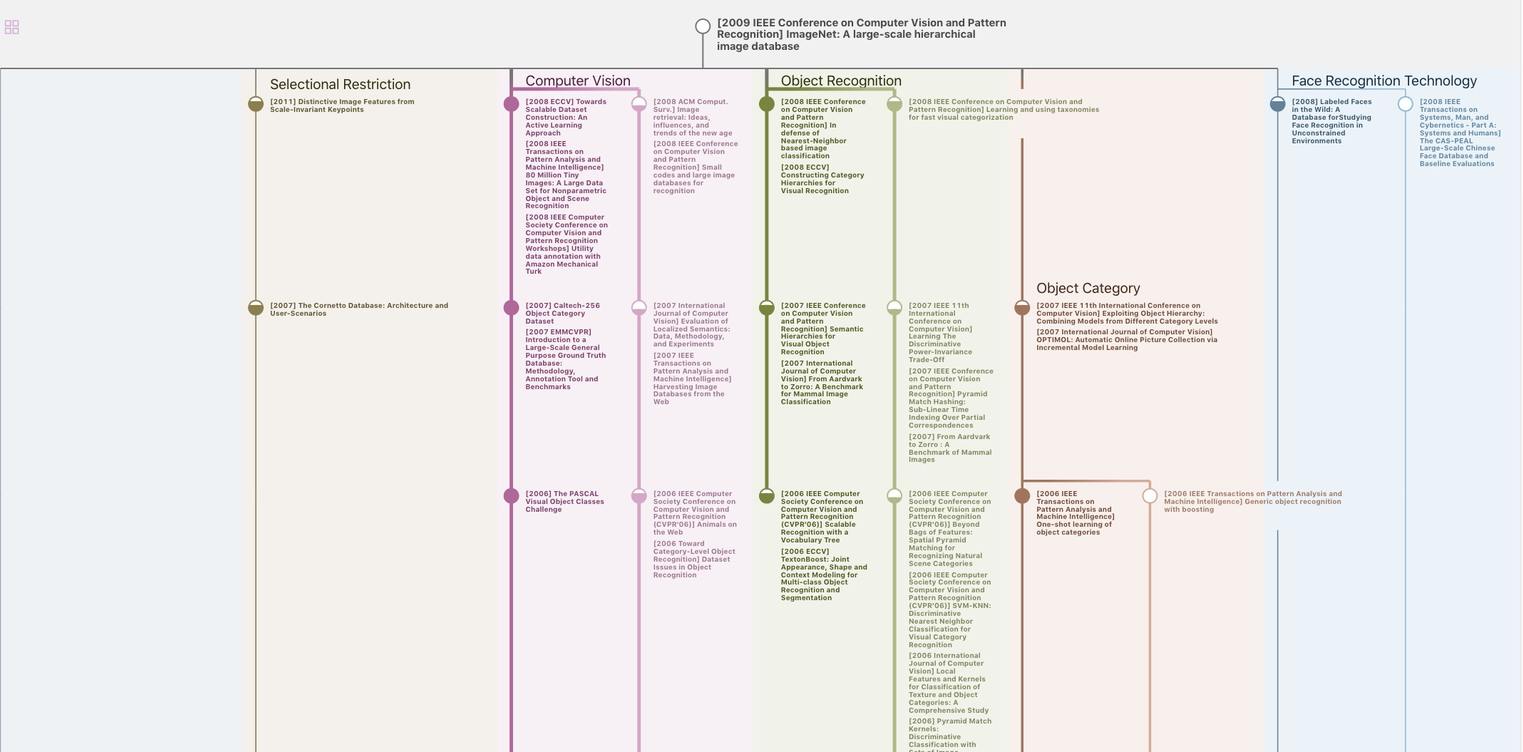
生成溯源树,研究论文发展脉络
Chat Paper
正在生成论文摘要