Robust sparse Bayesian learning for broad learning with application to high-speed railway track monitoring
STRUCTURAL HEALTH MONITORING-AN INTERNATIONAL JOURNAL(2023)
摘要
In this study, we focus on non-parametric probabilistic modeling for general regression analysis with large amounts of data and present an algorithm called the robust sparse Bayesian broad learning system. Robust sparse Bayesian learning is employed to infer the posterior distribution of the sparse connecting weight parameters in broad learning system. Regardless of the number of candidate features, our algorithm can always produce a compact subset of hidden-layer neurons of almost the same size learned from the data, which allows the algorithm to automatically adjust the model complexity of the network. This algorithm not only solves the regression problem of large amounts of data robustly but also possesses high computational efficiency and low requirements for computing hardware. Moreover, as a Bayesian probabilistic algorithm, it can provide the posterior uncertainty quantification of the predicted output, giving a measure of prediction confidence. The proposed algorithm is verified using simulated data generated by a benchmark function and also applied in non-parametric probabilistic modeling using high-speed railway track monitoring data. The results show that compared with several existing neural network algorithms, our proposed algorithm has strong model robustness, excellent prediction accuracy, and computational efficiency for regression analysis with large amounts of data, and has the potential to be widely used in general regression problems in science and engineering.
更多查看译文
关键词
robust sparse Bayesian learning, broad learning system, regression analysis, non-parametric probabilistic modeling, structural health monitoring, high-speed railway
AI 理解论文
溯源树
样例
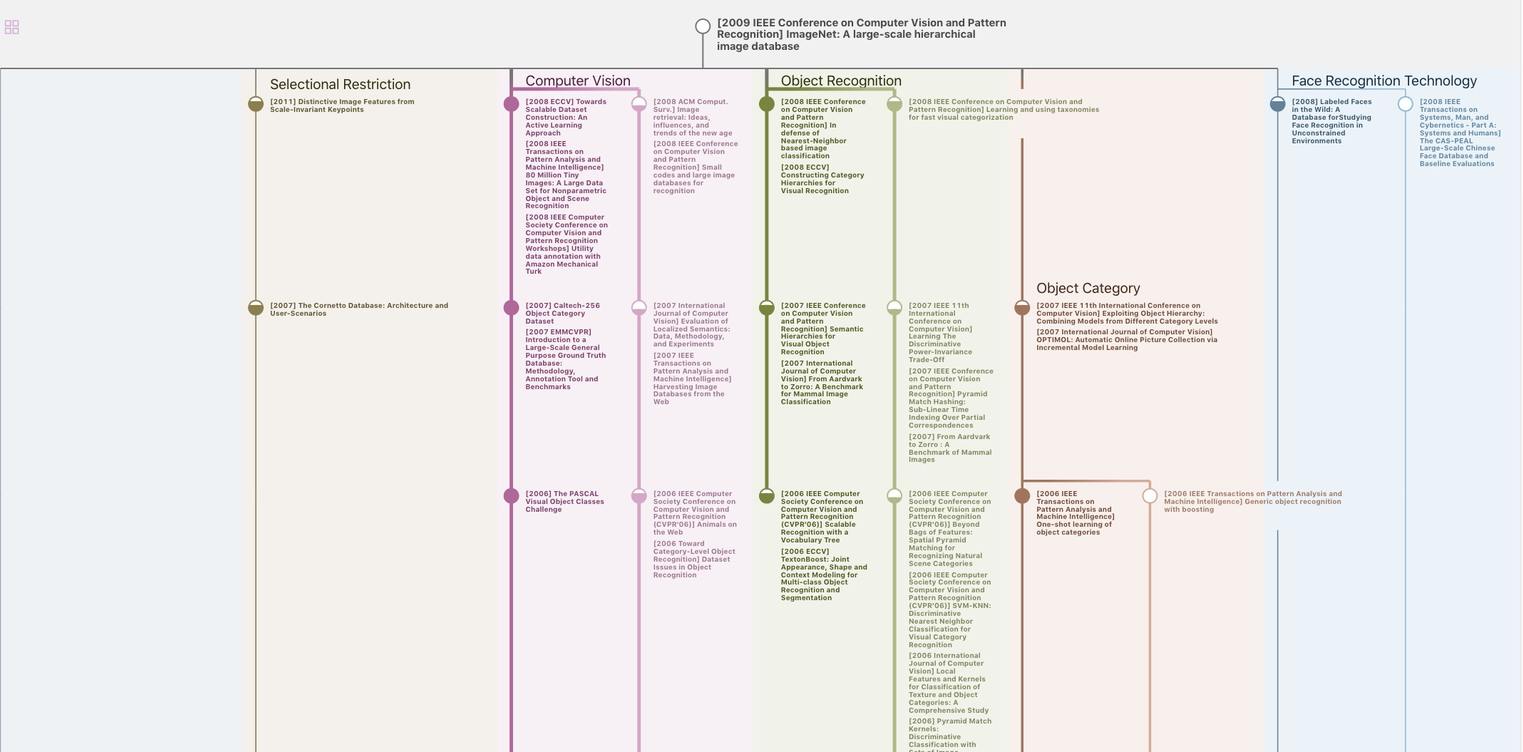
生成溯源树,研究论文发展脉络
Chat Paper
正在生成论文摘要