Machine learning-assisted design of biomedical high entropy alloys with low elastic modulus for orthopedic implants
Journal of Materials Science(2022)
摘要
This paper focuses on finding an optimum composition for the TiTaHfNbZr quinary high entropy alloy (HEA) system with an elastic modulus close to that of bone in order to attain a better biomechanical compatibility between the bone and the implant in orthopedic applications. To obtain the composition providing the desired structural match, machine learning (ML) tools were implemented in the current work instead of conventional trial-and-error methods. The ML algorithms utilized in this study were trained using experimental data available in the literature and then utilized to predict the optimum HEA compositions with the lowest elastic moduli. Consequently, the Ti 23 Ta 10 Hf 27 Nb 12 Zr 28 and Ti 28 Ta 10 Hf 30 Nb 14 Zr 18 compositions were predicted as the optimum HEA compositions with elastic moduli of 83.5 ± 2.9 and 87.4 ± 2.2 GPa, respectively. The materials were manufactured, and the elastic moduli were validated with nanoindentation experiments. The samples were also exposed to static immersion experiments in simulated body fluid (SBF) for 28 days to gain insight and information regarding the ion release and ensure that the new HEAs are biocompatible. The findings of the work reported herein demonstrate that the proposed ML model can successfully predict HEA compositions for an optimized biomechanical compatibility for orthopedic applications and warrant further biomedical research on the two new HEAs prior to their utility as orthopedic implant materials. Graphical abstract
更多查看译文
关键词
biomedical high entropy alloys,low elastic modulus,implants,learning-assisted
AI 理解论文
溯源树
样例
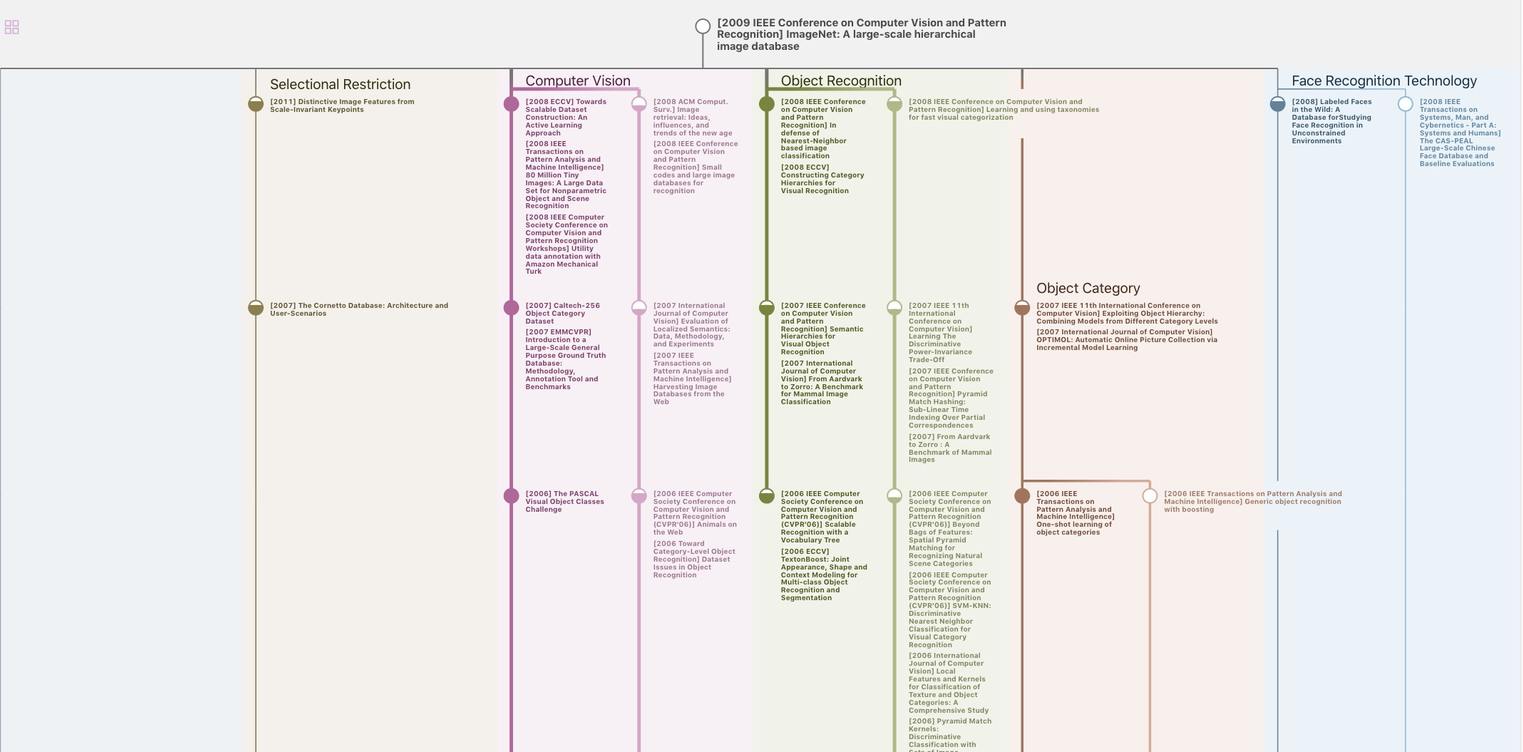
生成溯源树,研究论文发展脉络
Chat Paper
正在生成论文摘要