Resource Management in 5G Networks Assisted by UAV Base Stations: Machine Learning for Overloaded Macrocell Prediction Based on Users' Temporal and Spatial Flow
DRONES(2022)
摘要
The rapid growth of data traffic due to the demands of new services and applications poses new challenges to the wireless network. Unmanned aerial vehicles (UAVs) can be a solution to support wireless networks during congestion, especially in scenarios where the region has high traffic peaks due to the temporal and spatial flow of users. In this paper, an intelligent machine-learning-based system is proposed to deploy UAV base stations (UAV-BS) to temporarily support the mobile network in regions suffering from the congestion effect caused by the high density of users. The system includes two main steps, the load prediction algorithm (LPA) and the UAV-BSs clustering and positioning algorithm (UCPA). In LPA, the load history generated by the mobile network is used to predict which macrocells are congested. In UCPA, planning is performed to calculate the number of UAV BSs needed based on two strategies: naive and optimized, in addition to calculating the optimal positioning for each device requested to support the overloaded macrocells. For prediction, we used two models, generalized regression neural networks (GRNN) and random forest, and the results showed that both models were able to make accurate predictions, and the random forest model was better with an accuracy of over 85%. The results showed that the intelligent system significantly reduced the overhead of the affected macrocells, improved the quality of service (QoS), and reduced the probability of blocking users, as well as defined the preventive scheduling for the UAV BSs, which benefited the scheduling and energy efficiency.
更多查看译文
关键词
machine learning, UAV-BS, macrocell, QoS, temporal and spatial flow
AI 理解论文
溯源树
样例
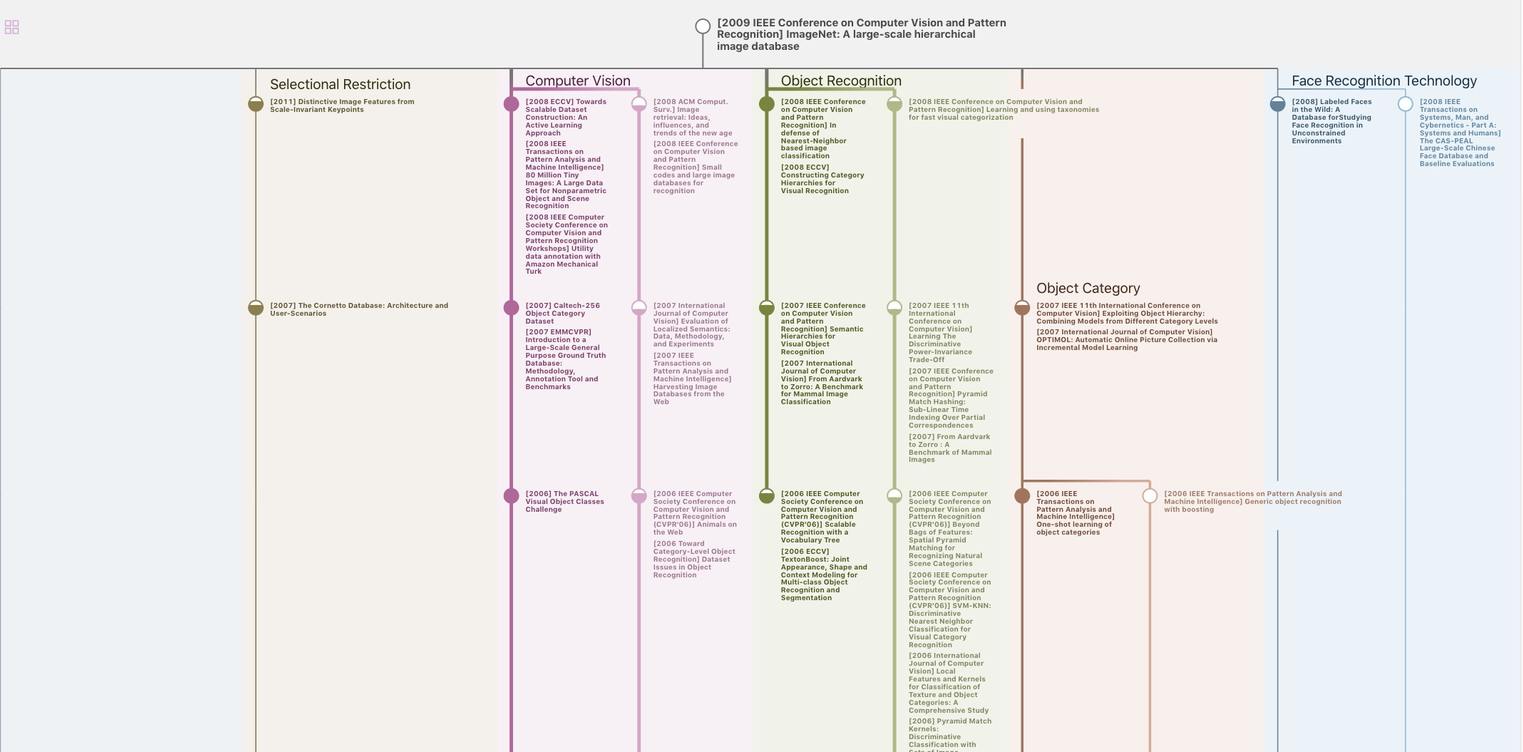
生成溯源树,研究论文发展脉络
Chat Paper
正在生成论文摘要