Leveraging Local Planar Motion Property for Robust Visual Matching and Localization
IEEE ROBOTICS AND AUTOMATION LETTERS(2022)
摘要
One primary difficulty preventing the visual localization for service robots is the robustness against changes, including environmental changes and perspective changes. In recent years, learning-based feature matching methods have been widely studied and effectively verified in practical applications. Learning-based feature matching effectively solves the problem of environmental changes, including illumination changes and man-made changes. However, there is still room for improvement dealing with large perspective changes. In this letter, we leverage local planar motion property to simplify the affine transform and propose an augmentation-based feature matching method that greatly enhances the robustness to perspective changes. The proposed feature matching approach maintains low matching costs as the augmentation is performed on the simplified affine matrix space. Combined with the motion property aided minimal solution for pose estimation, an end-to-end robust visual localization system is proposed which is shown to bring 67% improvement in localization performance under large perspective changes in publicly available OpenLORIS dataset, while increasing computational cost by only 20% by using batch processing techniques with a single GPU. In addition, a guide for map frame selection is presented to support robust localization with very sparse map frames in storage. Experiments on the classified dataset with environmental changes and perspective changes validate the effectiveness of the proposed system.
更多查看译文
关键词
Local planar motion property, robust visual matching, visual localization
AI 理解论文
溯源树
样例
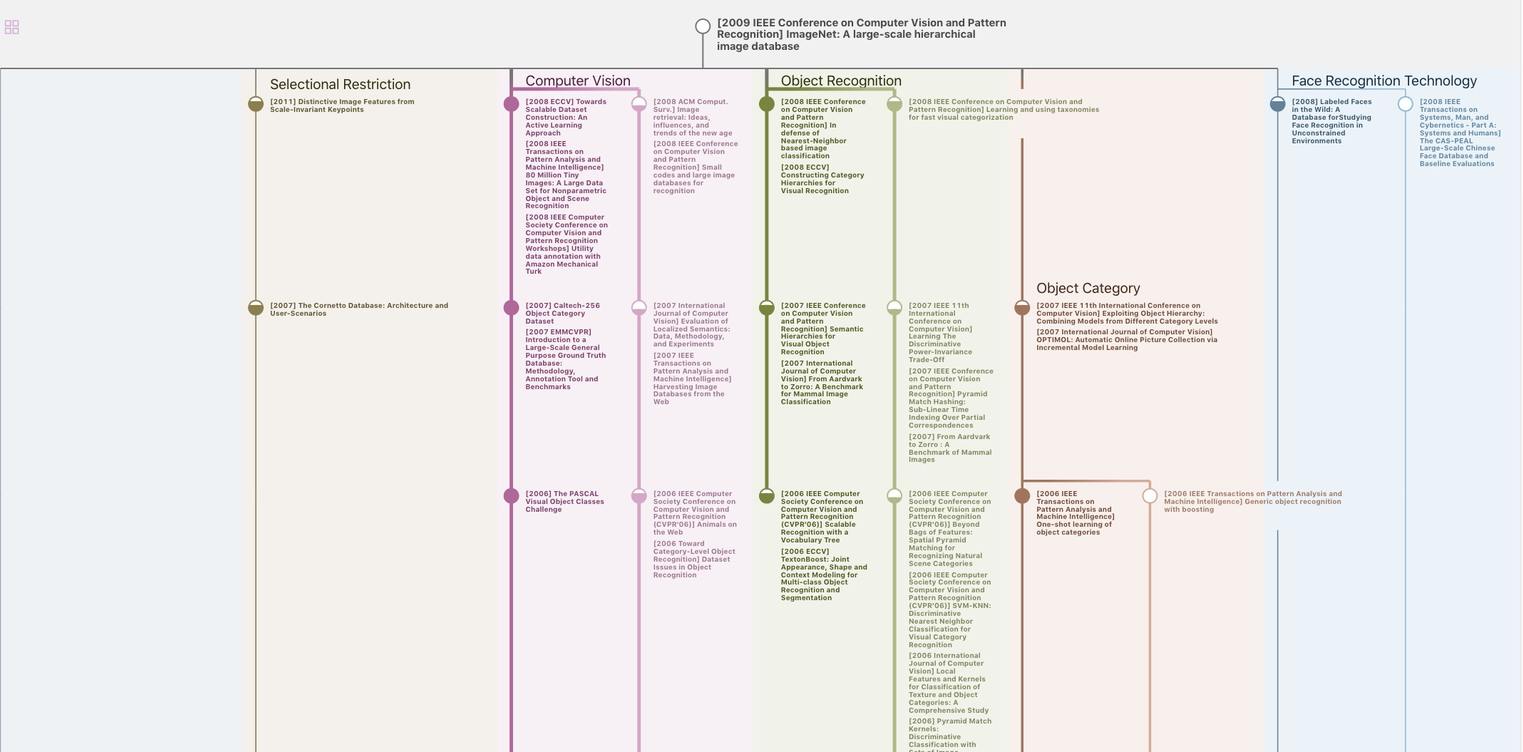
生成溯源树,研究论文发展脉络
Chat Paper
正在生成论文摘要