Adaptive Handling of Dependence in High-Dimensional Regression Modeling
JOURNAL OF COMPUTATIONAL AND GRAPHICAL STATISTICS(2023)
摘要
Dependence within a high-dimensional profile of explanatory variables affects estimation and prediction performance of regression models. However, the strong belief that dependence should not be ignored, based on our well-proven knowledge of low-dimensional regression modeling, is not necessarily true in high dimension. To investigate this point, we introduce a new class of prediction scores defined as linear combinations of a same random vector, including the naive prediction score obtained when ignoring dependence and the Ordinary Least Squares (OLS) prediction score that, on the contrary, fully accounts for dependence by a preliminary whitening of the explanatory variables. Interestingly, the former class also contains Ridge and Partial Least Squares prediction scores, that both offer intermediate ways of dealing with dependence. Through a theoretical comparative study, it is first shown how the best handling of dependence should depend on the interplay between the structure of conditional dependence across explanatory variables and the pattern of the association signal. We also derive the closed form expression of the prediction score with best prediction performance within the proposed class, leading to an adaptive handling of dependence. Finally, it is demonstrated through simulation studies and using benchmark datasets that this prediction score outperforms existing methods in various settings. Supplementary materials for this article are available online.
更多查看译文
关键词
Decorrelation, Naive prediction, Penalized estimation, Prediction, Rank-reduced estimation
AI 理解论文
溯源树
样例
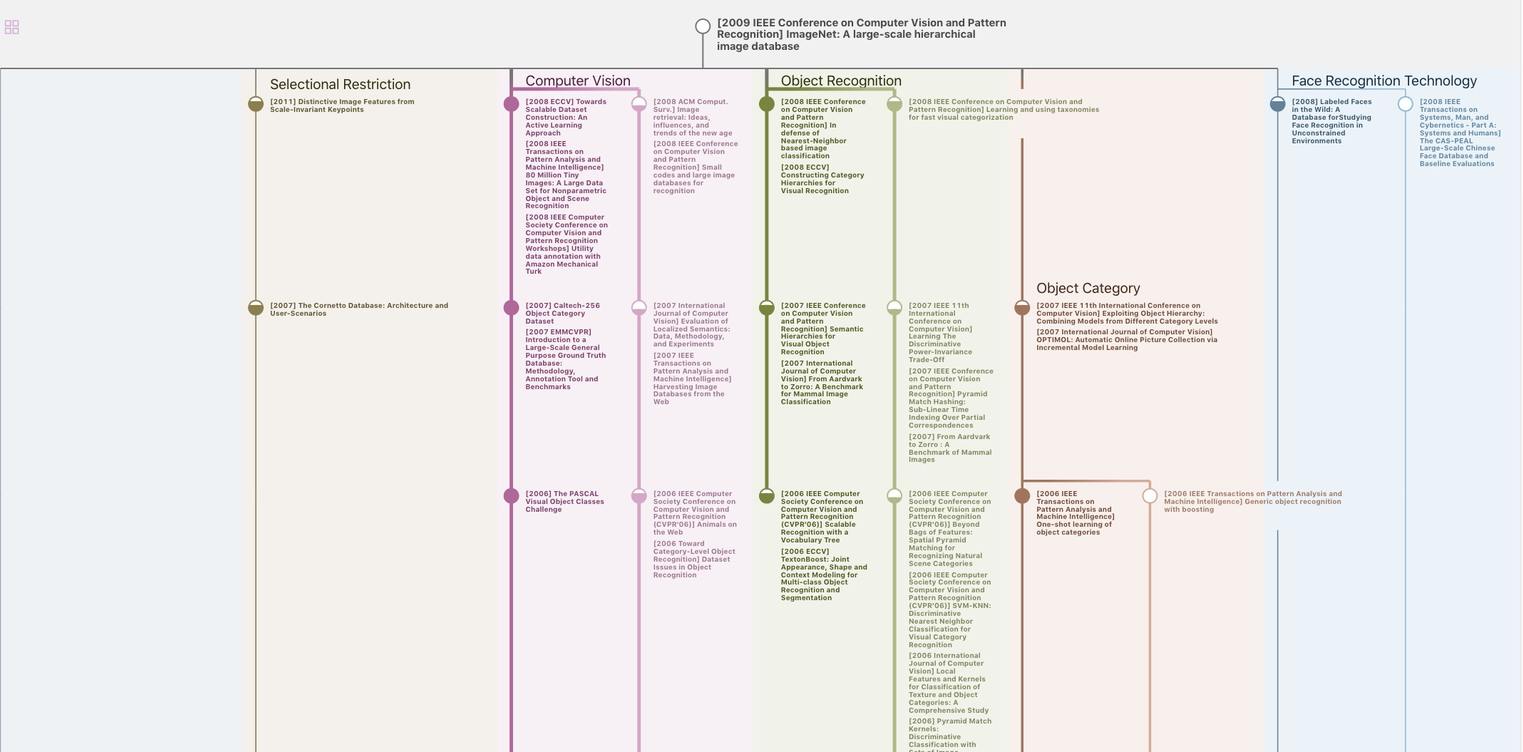
生成溯源树,研究论文发展脉络
Chat Paper
正在生成论文摘要