Physical-Parameter-Free Learning of Inverse Dynamics for Multi-DOF Industrial Robots via Sparsity and Feature Learning
Journal of Intelligent & Robotic Systems(2022)
摘要
Since learning models from data allows direct and accurate model approximation, it has lately become a tool of increasing interest in robotics. However, most learning methods require significant amounts of samples in the anticipated region to achieve adequate performance. In this paper, a novel modeling framework via sparsity and feature learning (SFL) is proposed for approximating multi-DOF manipulator inverse dynamics. A large number of features and their weights are recognized and selected from the analytical form of the dynamical equation. A matrix-free procedure based on the Alternating Direction Multiplier Method (ADMM) and reweighted L1-minimization technique are presented for time and space efficiency. Finally, we conducted the torque prediction and control experiments on a 7-DOF industrial robot to show the effectiveness of the proposed learning framework and compared the performance with different model classes and procedures. Superior to existing modeling methods, the merits of our method are that it needs no prior knowledge of the mechanical parameters of the robot thus avoiding modeling and assembly errors introduced by the geometric parameter identification procedure. Moreover, as the restriction of the features with specific forms is viewed as a strong priority of learning, the SFL method outperforms the universal approximation method on real data while generalizing for finite training samples.
更多查看译文
关键词
Robot manipulator,Inverse dynamics,Learning algorithm,Convex optimization,ADMM,Reweighted L1-minimization
AI 理解论文
溯源树
样例
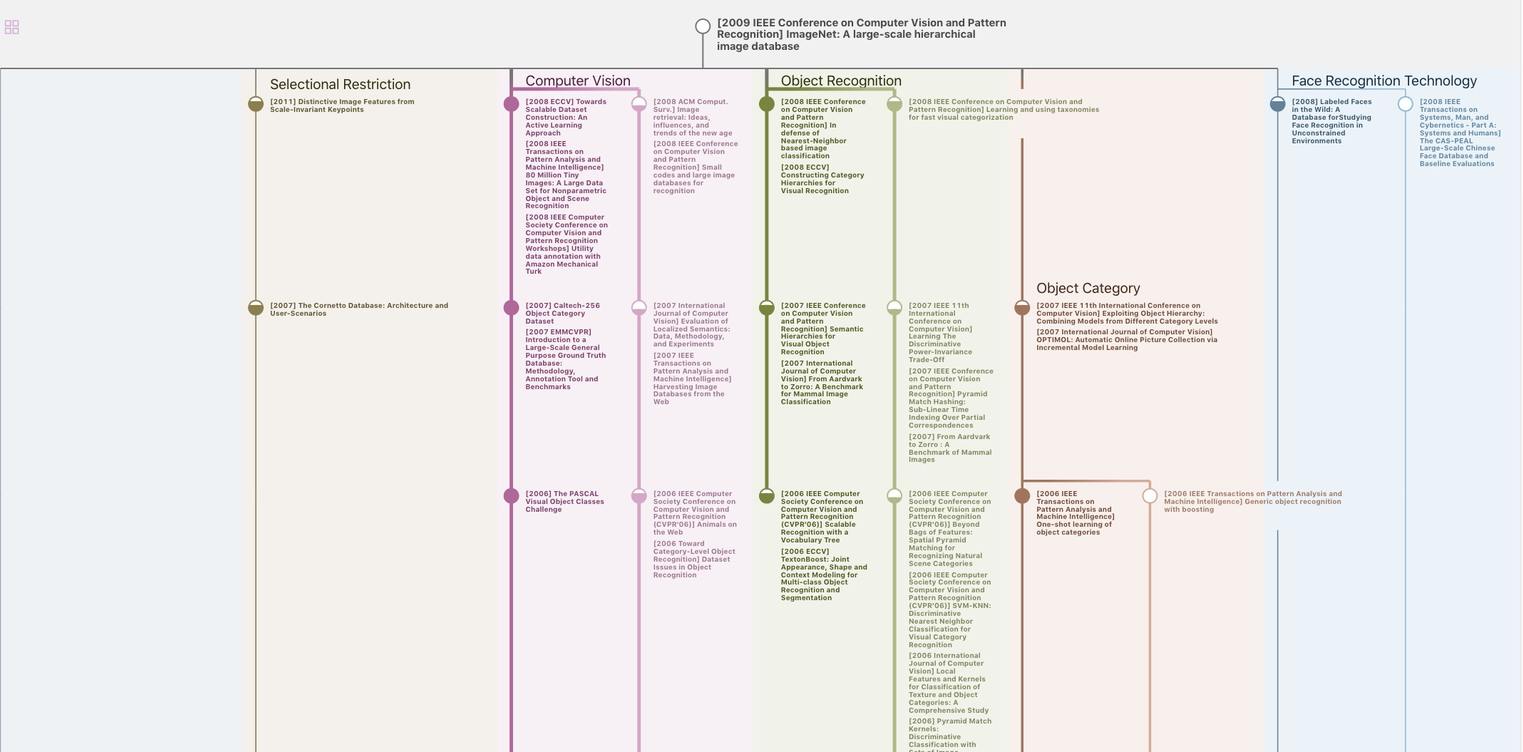
生成溯源树,研究论文发展脉络
Chat Paper
正在生成论文摘要