GIAD-ST: Detecting anomalies in human monitoring based on generative inpainting via self-supervised multi-task learning
JOURNAL OF INTELLIGENT INFORMATION SYSTEMS(2022)
摘要
In this paper, we propose a generative inpainting-based method to detect anomalous images in human monitoring via self-supervised multi-task learning. Our previous methods, where a deep captioning model is employed to find salient regions in an image and exploit caption information for each of them, detect anomalies in human monitoring at region level by considering the relations of overlapping regions. Here, we focus on image-level detection, which is preferable when humans prefer an immediate alert and handle them by themselves. However, in such a setting, the methods could show their deficiencies due to their reliance on the salient regions and their neglect of non-overlapping regions. Moreover, they take all regions equally important, which causes the performance to be easily influenced by unimportant regions. To alleviate these problems in image-level detection, we first employ inpainting techniques with a designed local and global loss to better capture the relation between a region and its surrounding area in an image. Then, we propose an attention-based Gaussian weighting anomaly score to combine all the regions by considering their importance for mitigating the influences of unimportant regions. The attention mechanism exploits multi-task learning for higher accuracy. Extensive experiments on two real-world datasets demonstrate the superiority of our method in terms of AUROC, precision, and recall over the baselines. The AUROC has improved from 0.933 to 0.989 and from 0.911 to 0.953 compared with the best baseline on the two datasets.
更多查看译文
关键词
Anomaly detection,Generative inpainting,Human monitoring,Self-supervised learning,Multi-task learning
AI 理解论文
溯源树
样例
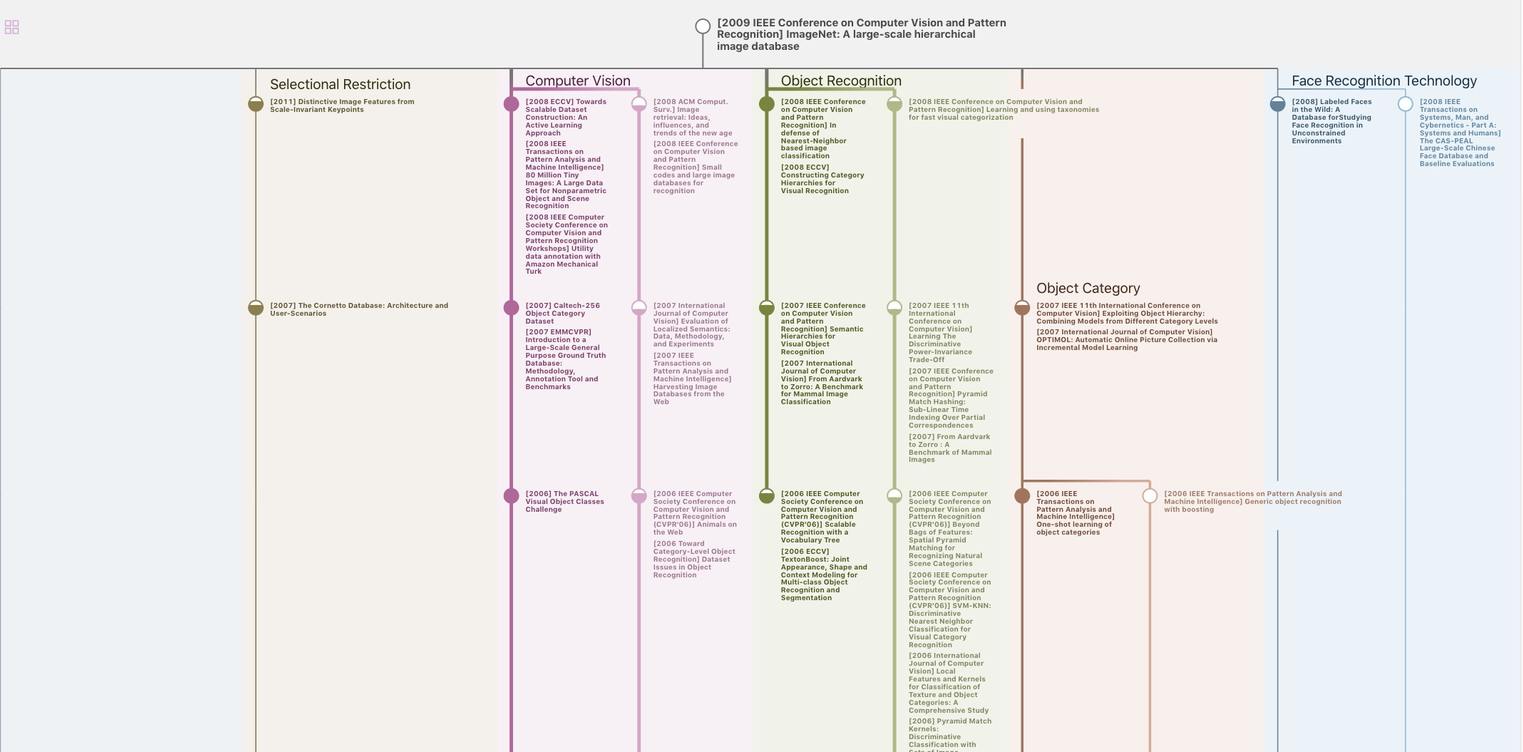
生成溯源树,研究论文发展脉络
Chat Paper
正在生成论文摘要