Hybrid Optimized Deep Neural Network with Enhanced Conditional Random Field Based Intrusion Detection on Wireless Sensor Network
NEURAL PROCESSING LETTERS(2022)
摘要
Security plays an important part in this Internet world because of the hasty improvement of Internet customers. Different Intrusion Detection Systems (IDS) have been advanced for various departments in history to describe and identify intruders utilizing data processing methods. Nonetheless, when using data processing, existing systems do not achieve adequate detection accuracy. For this reason, we suggest new IDS to offer preservation in statistics communications by completely describing intruders on wireless systems. Here, a new feature selection algorithm called enhanced conditional random field based feature selection to select the most contributed features and optimized hybrid deep neural network (OHDNN) is presented for the classification process. The hybrid deep neural network is a hybridization of convolution neural network (CNN) and long short-term memory (LSTM). To enhance the performance of the HDNN classifier, the parameters are optimized using adaptive golden eagle optimization. The performance of the presented approach is analyzed based on different metrics. For experimental analysis, the NSL-KDD and UNSW-NB15 datasets are used to compare its performance with other popular machine learning algorithms such as ANN, SVM, LSTM and CNN.
更多查看译文
关键词
Intrusion detection, Wireless sensor network, Optimized hybrid deep neural network, Conditional random field, Linear correlation coefficient, Adaptive golden eagle optimization
AI 理解论文
溯源树
样例
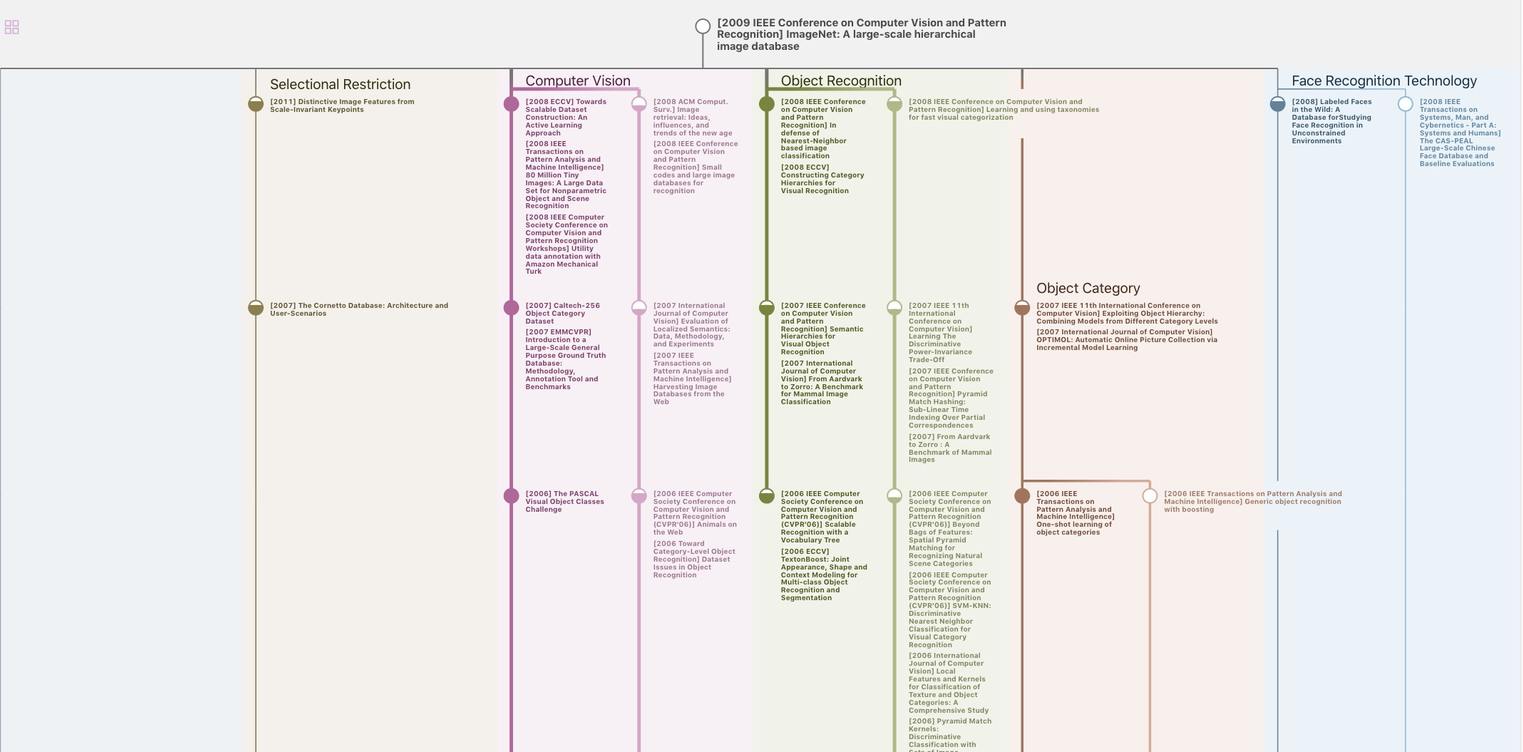
生成溯源树,研究论文发展脉络
Chat Paper
正在生成论文摘要