What makes multi-class imbalanced problems difficult? An experimental study
Expert Systems with Applications(2022)
摘要
Multi-class imbalanced classification is more difficult and less frequently studied than its binary counterpart. Moreover, research on the causes of the difficulty of multi-class imbalanced data is quite limited and insufficient. Therefore, we experimentally study the impact of various multi-class imbalanced difficulty factors on the performance of three popular classifiers. The results demonstrated a strong influence of the class overlapping with the extent of its impact related to the types of overlapped classes. In particular, overlapping between minority and majority classes was more difficult than the others. The type of the class size configuration turned out to be another important factor, highlighting the special role of the configurations with classes of intermediate sizes. The obtained results could support studying the nature of the multi-class imbalanced data as well as the development of new methods for improving classifiers.
更多查看译文
关键词
Imbalanced data,Classification,Learning from multiple classes,Data difficulty factors
AI 理解论文
溯源树
样例
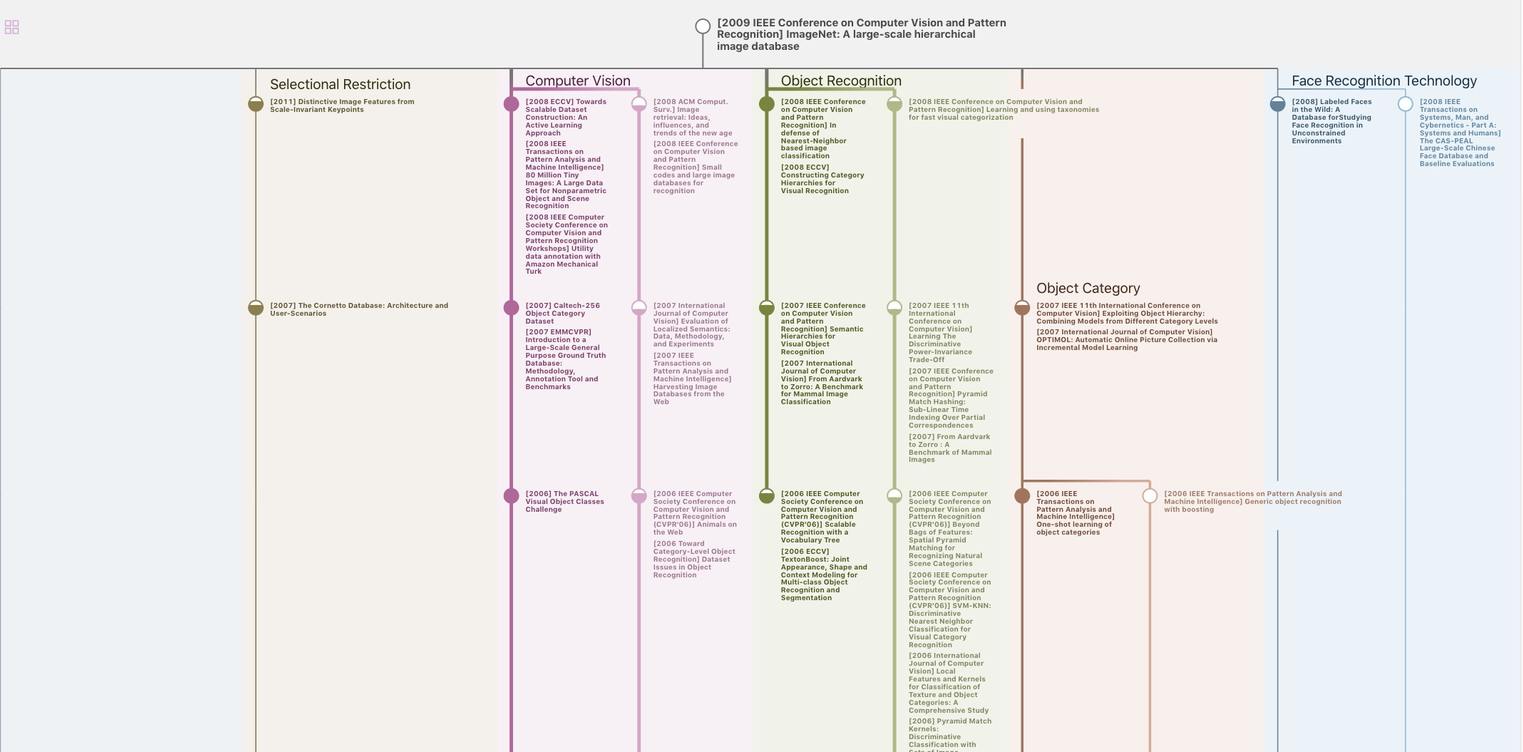
生成溯源树,研究论文发展脉络
Chat Paper
正在生成论文摘要