Modeling the butterfly behavior of SMA actuators using neural networks
COMPTES RENDUS MECANIQUE(2022)
摘要
Shape memory alloy (SMA) actuators are an important application of smart materials for robotics. However, the nonlinear behavior of SMA leads to difficulties in real-time simulations using numerical methods. Artificial Intelligence can be used to bypass this problem. In this paper, we study several neural networks (NNs) to model the superelastic or pseudo-elasticity effect (SEE) as well as the shape memory effect (SME) used in SMA. Focusing on antagonistic actuating, we first model a single wire to train the best NN with the proper characteristics that fit the behavior of SEE. Then, we model the SME of two linear antagonistic SMA wires used as an actuator. In both systems, single and antagonistic wires, we train the networks to obtain the stress-strain diagrams representing the behavior. The network type and training algorithm are key factors and are evaluated depending on the RMSE values. As a result, we find that the long short-term memory NN, used with a regression layer on standardized data sets, models the butterfly-shaped behavior of the actuator system with less RMSE value.
更多查看译文
关键词
Neural networks, Shape memory alloys, Shape memory effect, Superelastic effect, Hysteresis behavior, Antagonistic systems
AI 理解论文
溯源树
样例
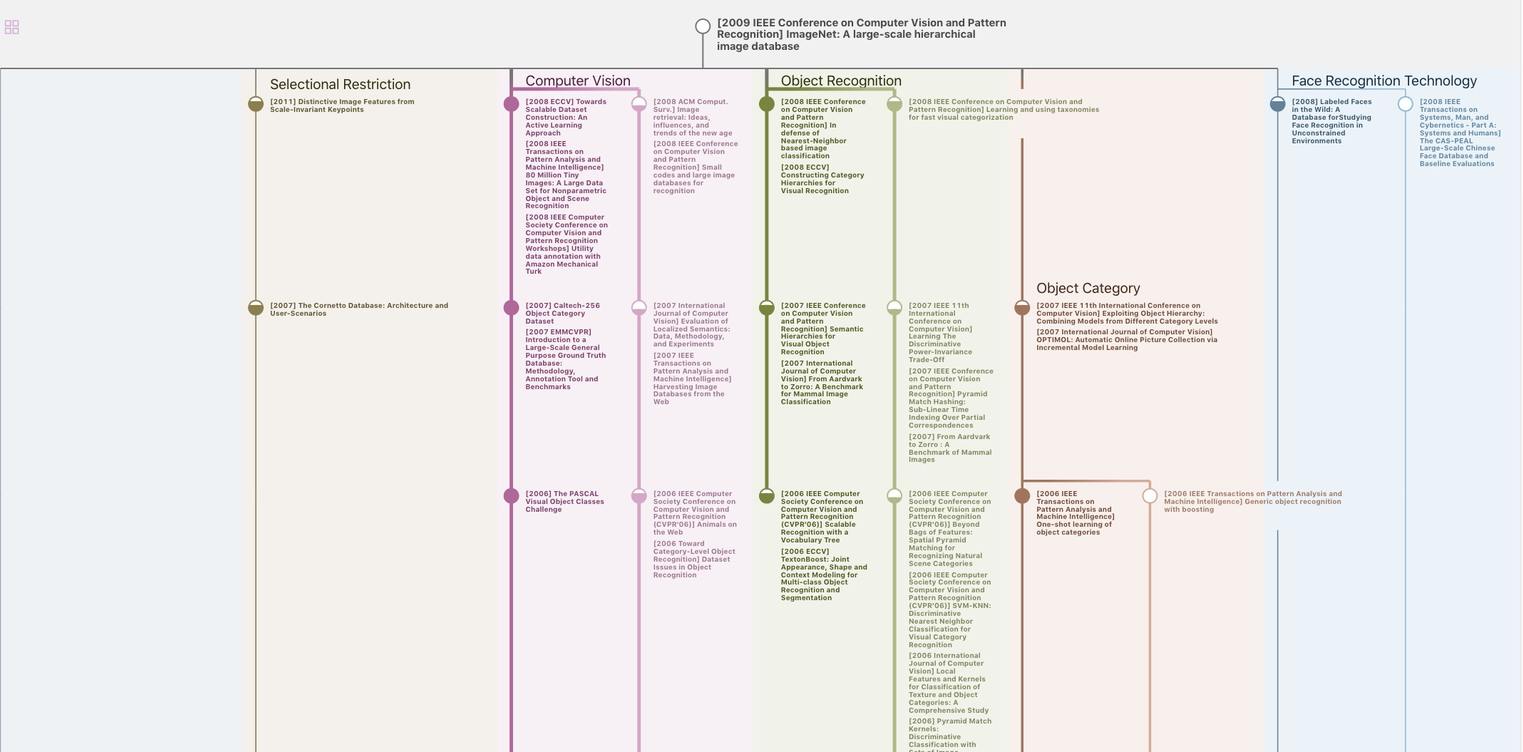
生成溯源树,研究论文发展脉络
Chat Paper
正在生成论文摘要