Improving estimation of urban land cover fractions with rigorous spatial endmember modeling
ISPRS Journal of Photogrammetry and Remote Sensing(2022)
摘要
The spatial information has been widely utilized in Spectral Mixture Analysis (SMA) studies over the years. However, the spatial autocorrelation, as a prerequisite of these studies, was seldomly examined. In the complex urban systems, it remains largely unknown whether and how land cover spectra at different locations are spatially autocorrelated and to which extent spatial autocorrelation can explain the great spectral variability. This study proposed an SMA method with Spatial Endmember Modeling (SEMSMA) to improve the fraction estimation of major urban land cover types. SEMSMA used the Incremental Spatial Autocorrelation (ISA) analysis to quantify spatial autocorrelation patterns in broadband reflectance. With the patterns, SEMSMA predicted endmember signatures that incorporated both spatial (location-oriented) and non-spatial spectral variability via the Restricted Maximum Likelihood-Empirical Best Linear Unbiased Predictor (REML-EBLUP) and Monte Carlo sampling. The spectral variabilities were then addressed in pixel unmixing by an iterative mixture analysis method. Results of ISA demonstrated diverse spatial autocorrelation patterns in reflectance of urban land covers. These patterns can be related to the pathways that spatial processes acted on land cover properties. The predicted endmember signatures explained most of the spectral variability for all land cover types. Subpixel fraction estimates showed that SEMSMA outperformed multiple endmember SMA (MESMA) and spatially adaptive SMA (SASMA), based on the root mean square error (0.04–0.15), the mean absolute error (0.01–0.09), and the Systematic Error (SE) (−80.00–0.01). The low SE of SEMSMA revealed unbiased estimation of land cover fractions. Results of this study underscored the necessity of spatial autocorrelation examination and the advantage of rigorous spatial endmember modeling when incorporating spatial information into SMA.
更多查看译文
关键词
Urban land cover,Incremental spatial autocorrelation,Spatial endmember modeling,REML-EBLUP,Multiple criteria spectral mixture analysis
AI 理解论文
溯源树
样例
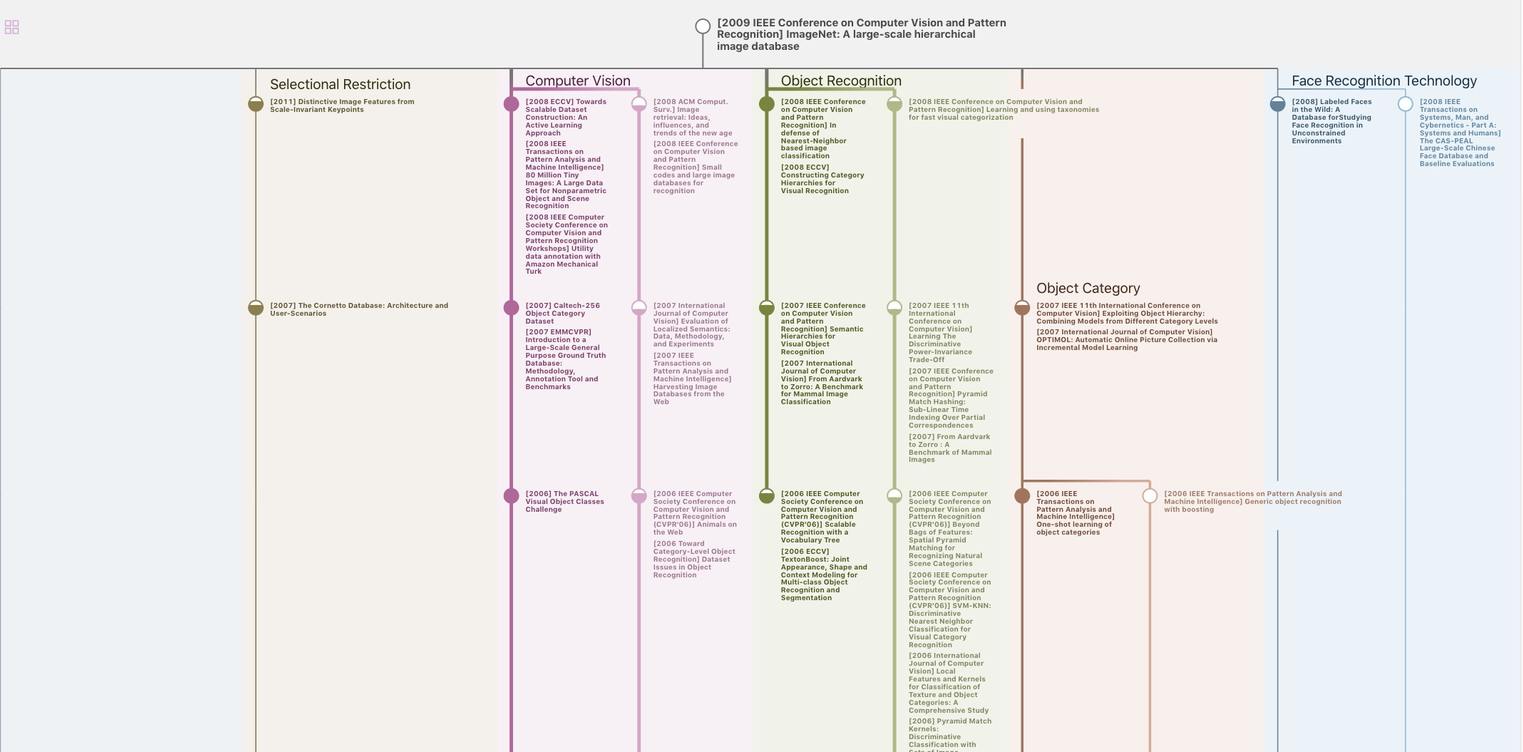
生成溯源树,研究论文发展脉络
Chat Paper
正在生成论文摘要