Attributed multiplex graph clustering: A heuristic clustering-aware network embedding approach
Physica A: Statistical Mechanics and its Applications(2022)
摘要
Attributed multiplex graph clustering, which partitions graph nodes from different relationships into different clusters with each cluster retaining structure closeness and attribute homogeneity simultaneously, is a fundamental graph data analysis task. Although network embedding has shown to be useful in a variety of graph analysis tasks, research dedicated to the attributed multiplex graph clustering, an important aspect of graph network analysis, is limited. Some efforts have been made for multiplex graph networks with network embedding techniques. However, most of this work has overlooked the clustering-aware information, which has not been fully explored. To solve these issues, we propose a fast method with sequenced-based network embedding for attributed multiplex graph clustering, namely NEAMC, to efficiently capture the clustering-aware information between nodes and different relationships and place them into a uniform vector space. A novel and heuristic clustering-aware across multi-relationship random walk node sampling strategy is proposed, which can not only select the relationship to proceed according to the information level of each relationship to retain the clustering-aware relationship and interaction among different relationships, but also leverage the node similarity to sample node sequences to retain the clustering-aware interactive information between nodes. Therefore, NEAMC can improve the attributed multiplex graph clustering to maintain the structure closeness and attribute homogeneity of the cluster. NEAMC is evaluated on five datasets, and the results demonstrate that it outperforms existing competitive baselines.
更多查看译文
关键词
Attributed multiplex graph clustering,Sequence-based network embedding,Heuristic clustering-aware across multi-relationship random walk node sampling,Structure closeness,Attribute homogeneity
AI 理解论文
溯源树
样例
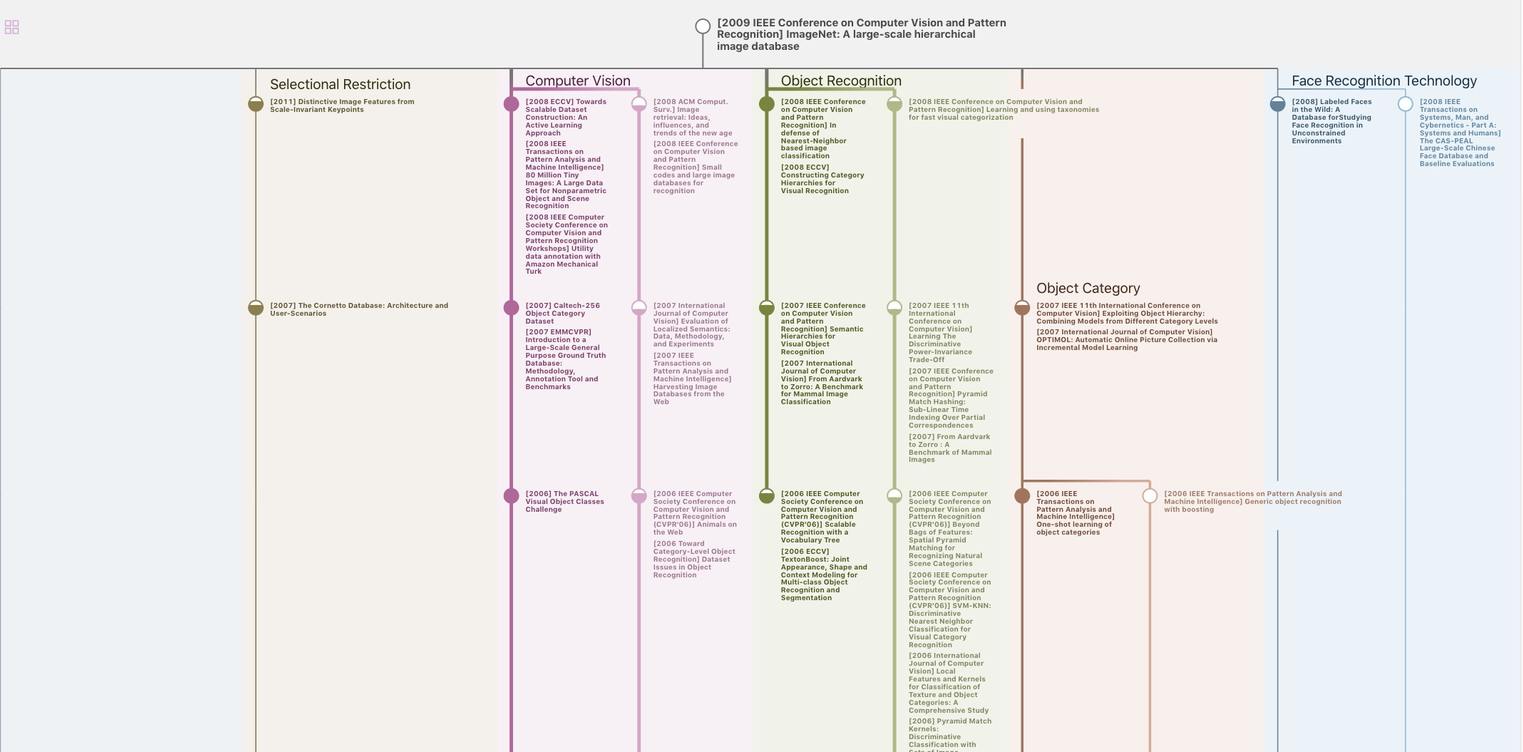
生成溯源树,研究论文发展脉络
Chat Paper
正在生成论文摘要