Count-Based Exploration via Embedded State Space for Deep Reinforcement Learning
WIRELESS COMMUNICATIONS & MOBILE COMPUTING(2022)
摘要
Count-based exploration algorithms have shown to be effective in dealing with various deep reinforcement learning tasks. However, existing count-based exploration algorithms cannot work well in high-dimensional state space due to the complexity of state representation. In this paper, we propose a novel count-based exploration method, which can explore high-dimensional continuous state space and combine with any reinforcement learning algorithms. Specifically, by introducing the embedding network to encode the state space and to merge the states with similar key characteristics, we can compress the high-dimensional state space. By utilizing the state binary code to count the occurrence number of states, we generate additional rewards which can encourage the agent to explore the environment. Extensive experimental results on several commonly used environments show that our proposed method outperforms other strong baselines significantly.
更多查看译文
AI 理解论文
溯源树
样例
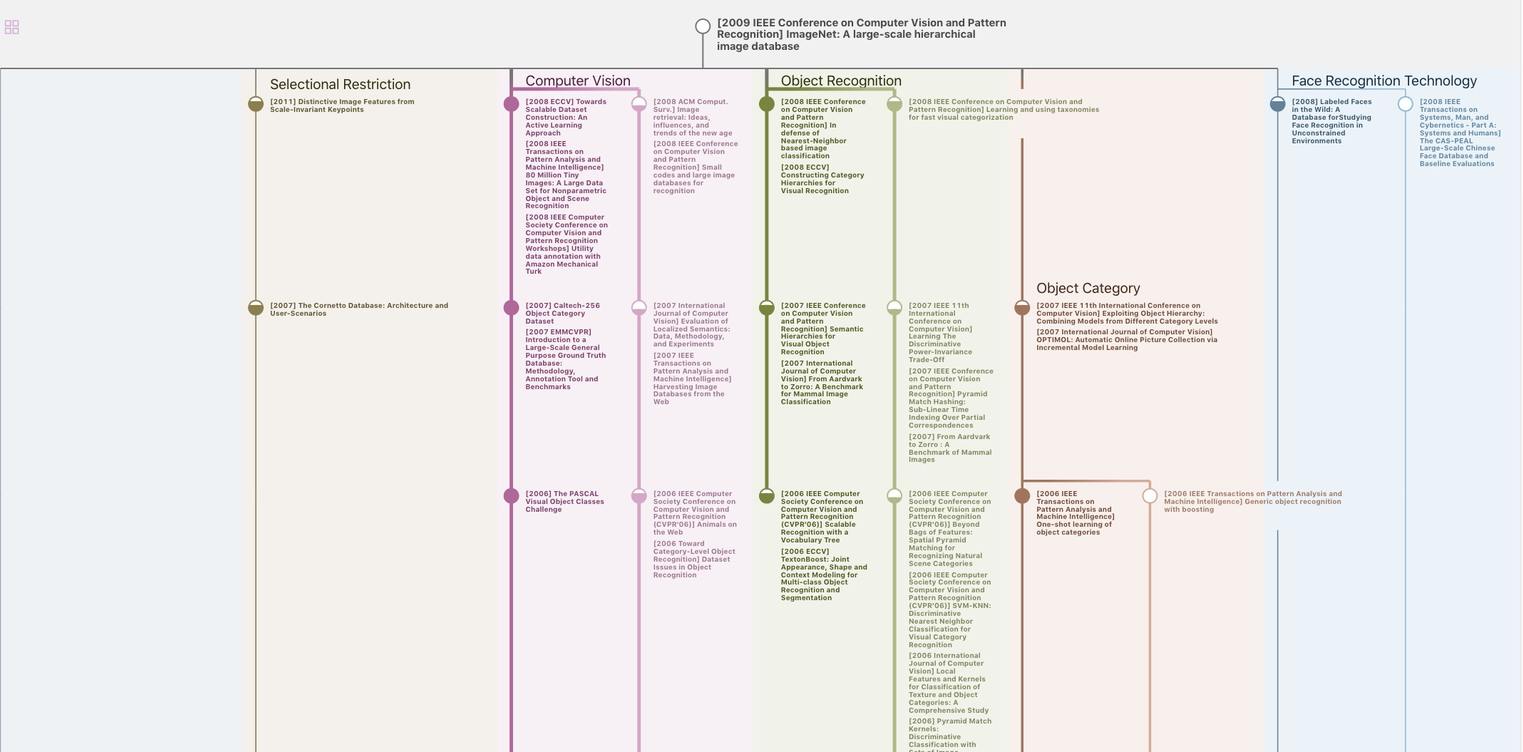
生成溯源树,研究论文发展脉络
Chat Paper
正在生成论文摘要