Predicting the Materials Properties Using a 3D Graph Neural Network With Invariant Representation
IEEE ACCESS(2022)
摘要
Accurate prediction of physical properties is critical for discovering and designing novel materials. Machine learning technologies have attracted significant attention in the materials science community for their potential for large-scale screening. Graph Convolution Neural Network (GCNN) is one of the most successful machine learning methods because of its flexibility and effectiveness in describing 3D structural data. Most existing GCNN models focus on the topological structure but overly simplify the three-dimensional geometric structure. However, in materials science, the 3D-spatial distribution of atoms is crucial for determining the atomic states and interatomic forces. This paper proposes an adaptive GCNN with a novel convolution mechanism that simultaneously models atomic interactions among all neighboring atoms in three-dimensional space. We apply the proposed model to two distinctly challenging materials properties prediction problems. The first is Henry's constant for gas adsorption in Metal-Organic Frameworks (MOFs), which is notoriously difficult because of its high sensitivity to atomic configurations. The second is the ion conductivity in solid-state crystal materials, which is difficult because of the few labeled data available for training. The new model outperforms existing graph-based models on both data sets, suggesting that the critical three-dimensional geometric information is indeed captured.
更多查看译文
关键词
Predictive models, Convolution, Solid modeling, Crystals, Adaptation models, Materials science and technology, Convolutional neural networks, Graph convolution neural network, metal-organic frameworks, Henry's constant, property prediction
AI 理解论文
溯源树
样例
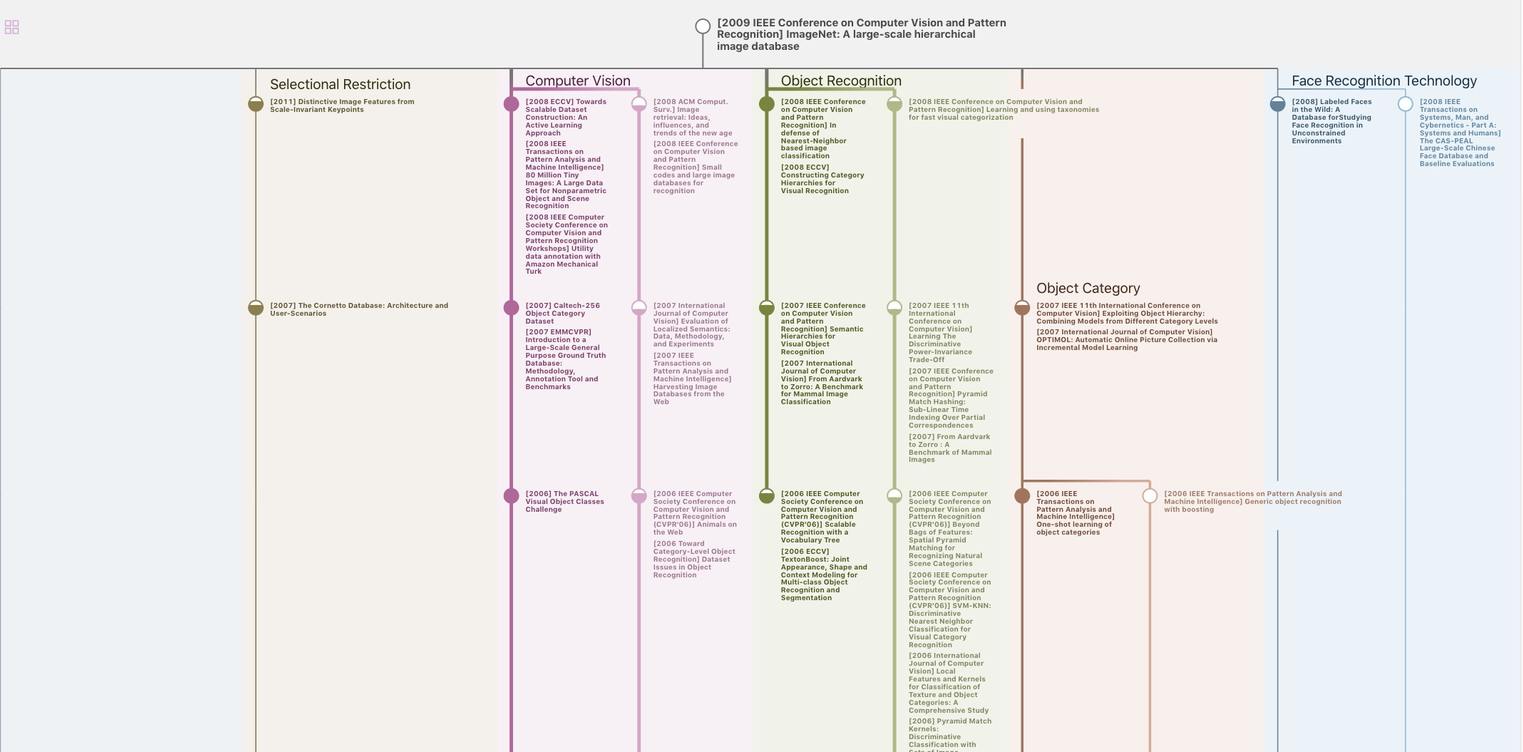
生成溯源树,研究论文发展脉络
Chat Paper
正在生成论文摘要