Systolic-RAM: Scalable Direct Convolution Using In-Memory Data Movement
IEEE Solid-State Circuits Letters(2022)
摘要
A 12.8-kbit static random access memory (SRAM) is demonstrated in 40-nm CMOS for signed 8-bit convolution in-memory. While conventional compute-in-memory (CIM) approaches rely on the indirect convolution algorithm, the proposed “Systolic-RAM” performs a form of direct convolution which eliminates the need for data duplication and near-memory registers. To achieve this, an in-memory data pipeline is employed to move data within the array and mimic the physical movement of the convolution kernel. In between data movement cycles, back-end-of-line (BEOL) structures perform charge-domain vector-matrix multiplication (VMM). The indirect convolution algorithm is illustrated, and the supporting circuits are presented in detail. Quantized neural network training methods are also employed to achieve test accuracy close to that of a floating-point network. The demonstrated array is configured for a
$5 \times 5 $
kernel, achieves 175(113) peak (continuous) multiply accumulate (MAC) operations per clock cycle and consumes 3.0 mW at 100 MHz.
更多查看译文
关键词
Analog computing,artificial neural networks,convolution,random access memory
AI 理解论文
溯源树
样例
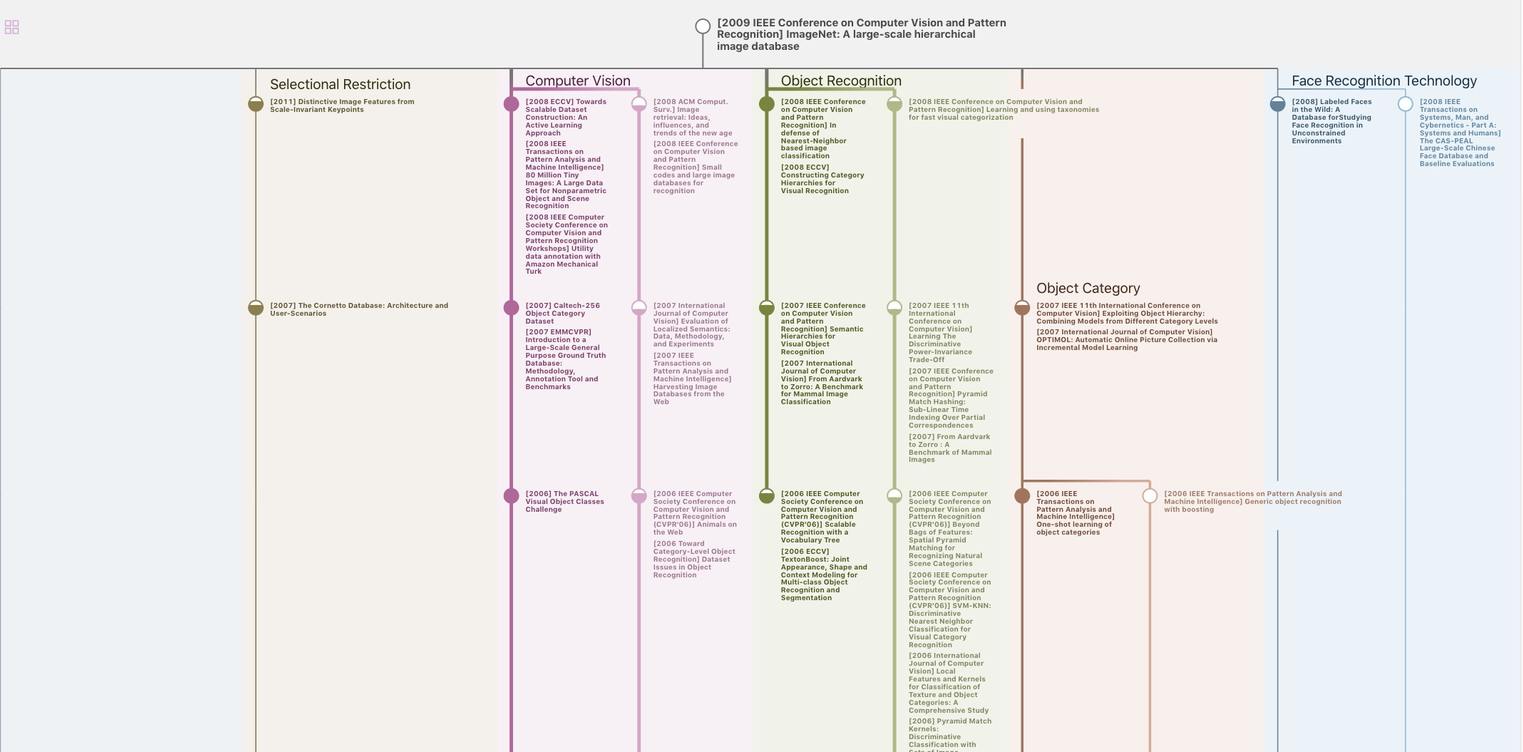
生成溯源树,研究论文发展脉络
Chat Paper
正在生成论文摘要