Combined Prediction of Photovoltaic Power Based on Sparrow Search Algorithm Optimized Convolution Long and Short-Term Memory Hybrid Neural Network
ELECTRONICS(2022)
摘要
To address the problem of strong uncertainty in the high proportion of new energy output, an improved convolutional long- and short-term memory (CLSTM) hybrid neural network is proposed for PV power combination prediction. Firstly, considering the large impact of weather changes on PV power output, a fluctuation feature identification model is used to classify historical PV power series samples into slow weather change type and severe weather change type. Secondly, taking into account the multimodal characteristics of PV power output, an improved variational modal decomposition technique is used to adaptively determine the number of modal components, K, and decompose the two types of samples. Regarding the existence of the low-frequency steady state component and the high-frequency fluctuation component of PV power output, the high-frequency component is used to train the long- and short-term memory (LSTM) model and the low-frequency component is used to train the convolutional neural network (CNN) model. The improved sparrow search algorithm (SSA) is used to optimize the parameters of the LSTM and CNN models during the training process. Finally, the predicted component values of each model are superimposed and reconstructed to obtain PV power prediction values. The actual operation data of a PV plant in northern China were used for comparison and validation, and the experiments showed that the accuracy of the prediction results, based on the improved SSA to optimize the parameters of the CLSTM hybrid neural network for predicting PV output, was significantly better than that of the BP, CNN, LSTM single neural network prediction results, and of the prediction accuracy of the unoptimized CLSTM hybrid neural network. At the same time, compared with the above single neural network and unoptimized hybrid prediction model, the proposed method converged faster and was more adaptable to weather changes.
更多查看译文
关键词
photovoltaic power combination prediction, fluctuation feature recognition model, improved variational modal decomposition, CLSTM hybrid neural network
AI 理解论文
溯源树
样例
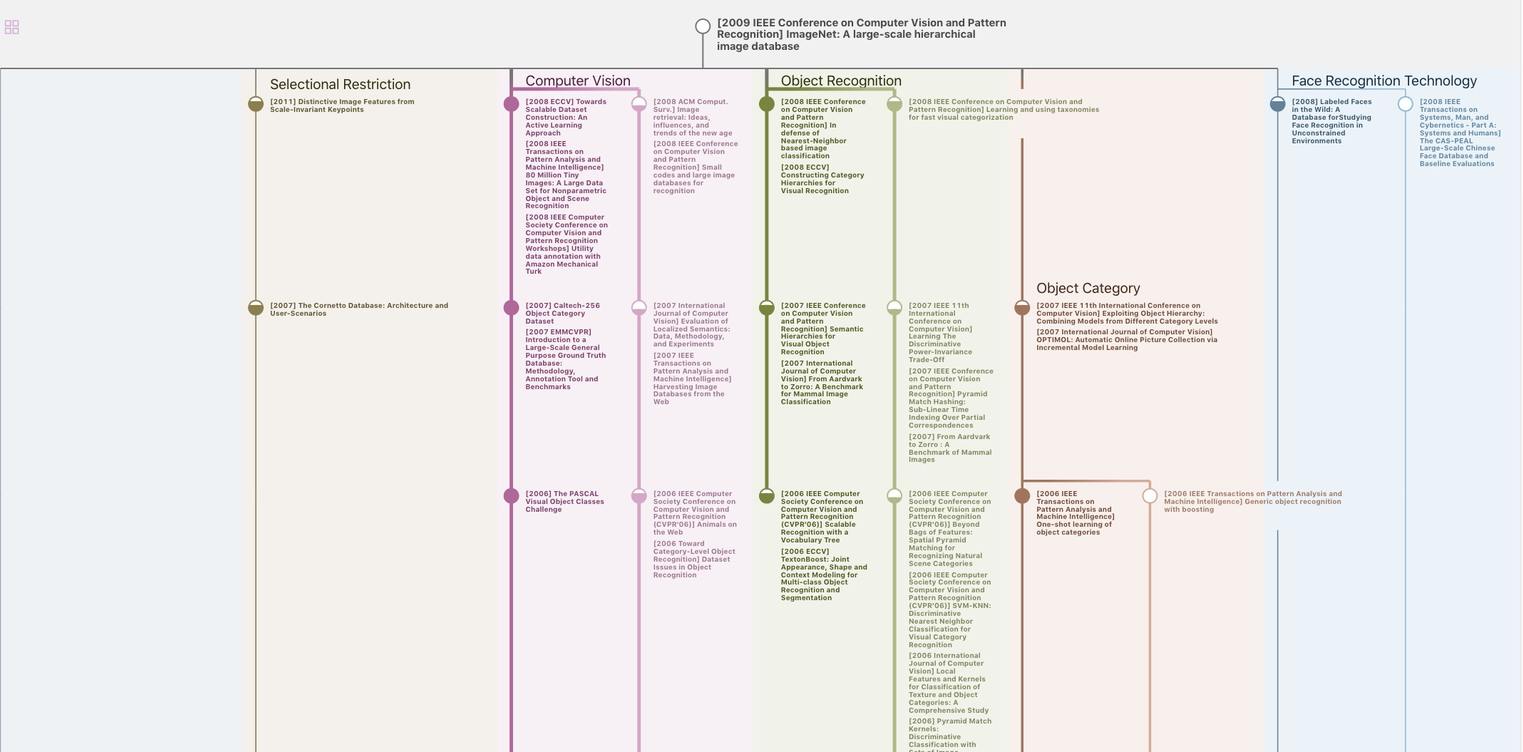
生成溯源树,研究论文发展脉络
Chat Paper
正在生成论文摘要