Multilabel causal variable discovery in multisource
CONCURRENCY AND COMPUTATION-PRACTICE & EXPERIENCE(2022)
摘要
Multilabel causal feature selection, as a well-known and effective approach in dealing with high-dimensional multilabel data, is a popular topic. Amount of causal feature selection algorithms have achieved a great deal of success in classification and prediction tasks. However, the descriptive information of data is collected from different data sources in many practical applications. While few researches focus on the causal variable discovery in multisource environments due to the complex causal relationships. To address these problems, we propose a causal feature selection framework in multisource environments to solve the above problems. Firstly, we mine the causal mechanism with respect to the class attribute under the assumption that only a single data source is included. Secondly, by utilizing the concept of causal invariance in causal inference, we formulate the problem of causal feature selection with multiple data sources as a search problem for an invariant set across data sources. In addition, we give the upper and lower bounds of the causal invariant set. Finally, we design a novel multisource multilabel causal feature selection (MMCFS) algorithm. To verify the effectiveness of the proposed algorithm, we compare it with 12 feature selection methods on synthetic datasets. Experiment results show that the classification performance of MMCFS achieves highly competitive performance against other comparing algorithms.
更多查看译文
关键词
causal invariant, causal variable discovery, Markov boundary, multilabel feature selection, multisource
AI 理解论文
溯源树
样例
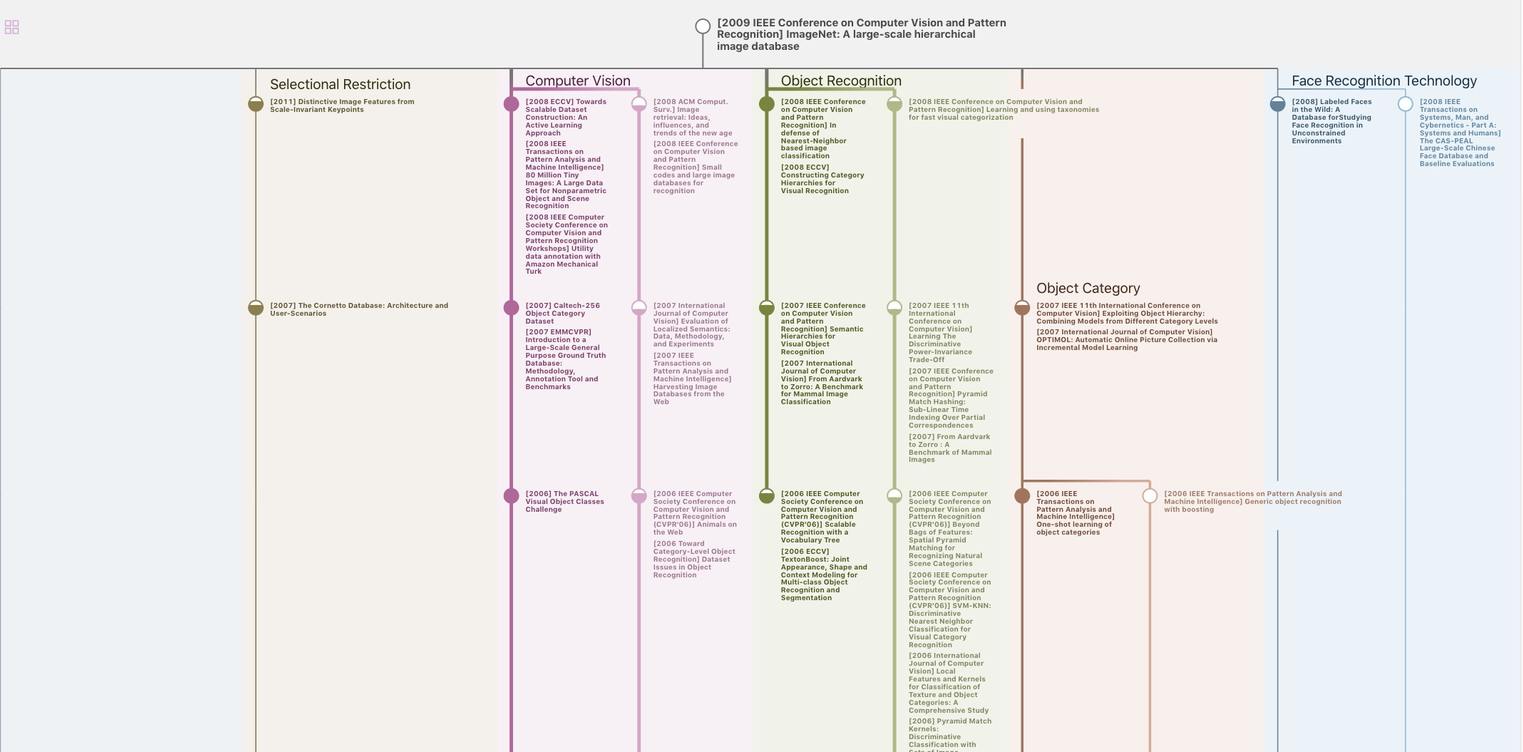
生成溯源树,研究论文发展脉络
Chat Paper
正在生成论文摘要