Deep learning-based hyperspectral image reconstruction from emulated and real computed tomography imaging spectrometer data
OPTICAL ENGINEERING(2022)
摘要
The computed tomography imaging spectrometer (CTIS) is a hyperspectral imaging (HSI) approach where spectral and spatial information of a scene is mixed during the imaging process onto a monochromatic sensor. This mixing is due to a diffractive optical element integrated into the underlying optics and creates a set of diffraction orders. To reconstruct a three-dimensional hyperspectral cube from the CTIS sensor image, iterative algorithms were applied. Unfortunately, such methods are highly sensitive to noise and require high computational time for reconstruction thus hindering their applicability in real-time and high frame-rate applications. To overcome such limitations, we propose a lightweight and efficient deep convolutional neural network for hyperspectral image reconstruction from CTIS sensor images. Compared with classical approaches our model delivers considerably better reconstruction results on synthetic as well as real CTIS images in under 0.17 s, which is over 60 times faster compared with the standard iterative approach. In addition, the reshaping method we have developed enables a lightweight network architecture with over 100 times fewer parameters than previously reported. (c) The Authors. Published by SPIE under a Creative Commons Attribution 4.0 International License. Distribution or reproduction of this work in whole or in part requires full attribution of the original publication, including its DOI.
更多查看译文
关键词
hyperspectral imaging, neural networks, computed tomography imaging spectrometer, hyperspectral reconstruction
AI 理解论文
溯源树
样例
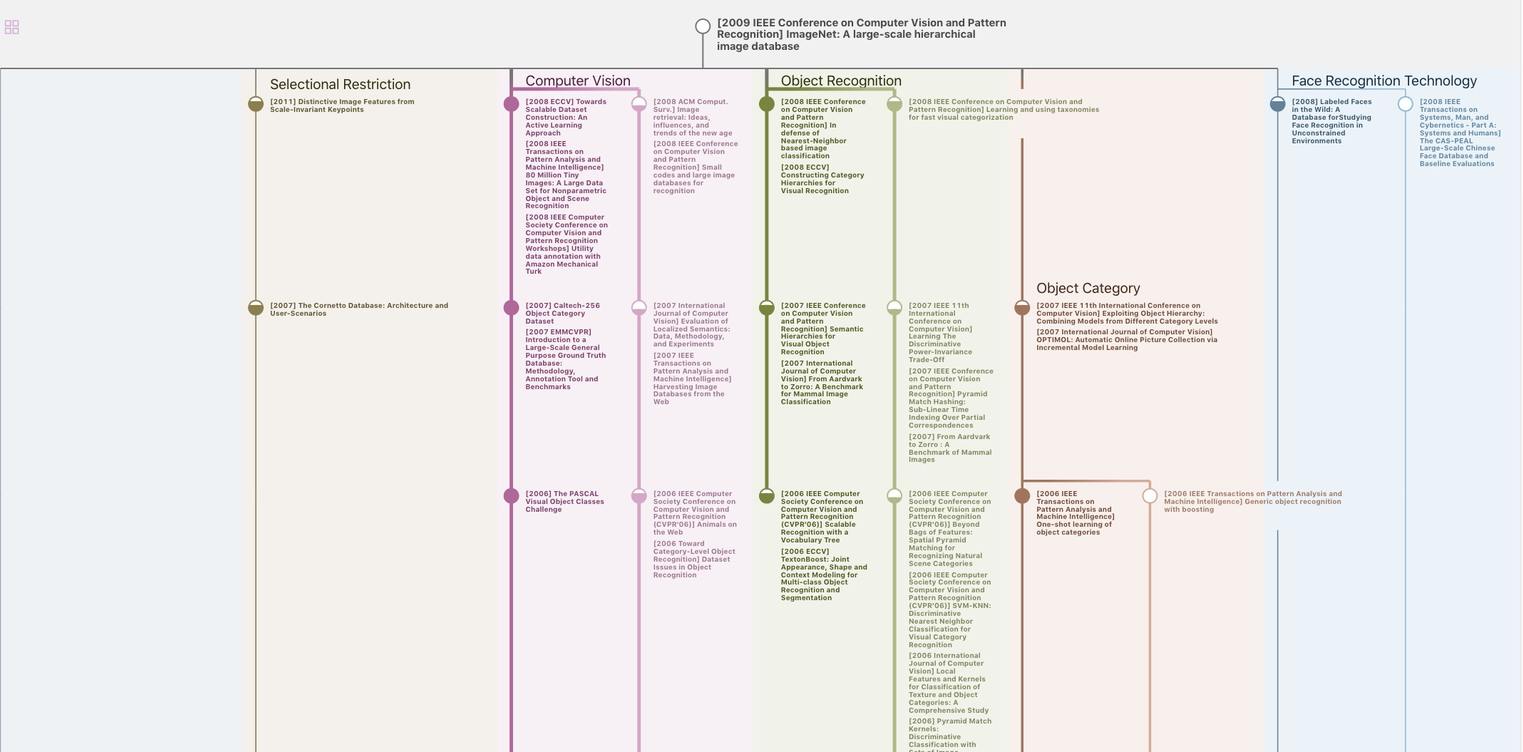
生成溯源树,研究论文发展脉络
Chat Paper
正在生成论文摘要