Investigating How Reproducibility and Geometrical Representation in UMAP Dimensionality Reduction Impact the Stratification of Breast Cancer Tumors
APPLIED SCIENCES-BASEL(2022)
摘要
Advances in next-generation sequencing have provided high-dimensional RNA-seq datasets, allowing the stratification of some tumor patients based on their transcriptomic profiles. Machine learning methods have been used to reduce and cluster high-dimensional data. Recently, uniform manifold approximation and projection (UMAP) was applied to project genomic datasets in low-dimensional Euclidean latent space. Here, we evaluated how different representations of the UMAP embedding can impact the analysis of breast cancer (BC) stratification. We projected BC RNA-seq data on Euclidean, spherical, and hyperbolic spaces, and stratified BC patients via clustering algorithms. We also proposed a pipeline to yield more reproducible clustering outputs. The results show how the selection of the latent space can affect downstream stratification results and suggest that the exploration of different geometrical representations is recommended to explore data structure and samples' relationships.
更多查看译文
关键词
UMAP, dimensionality reduction, clustering, embedding geometry, RNA-seq, breast cancer, tumor stratification, reproducibility
AI 理解论文
溯源树
样例
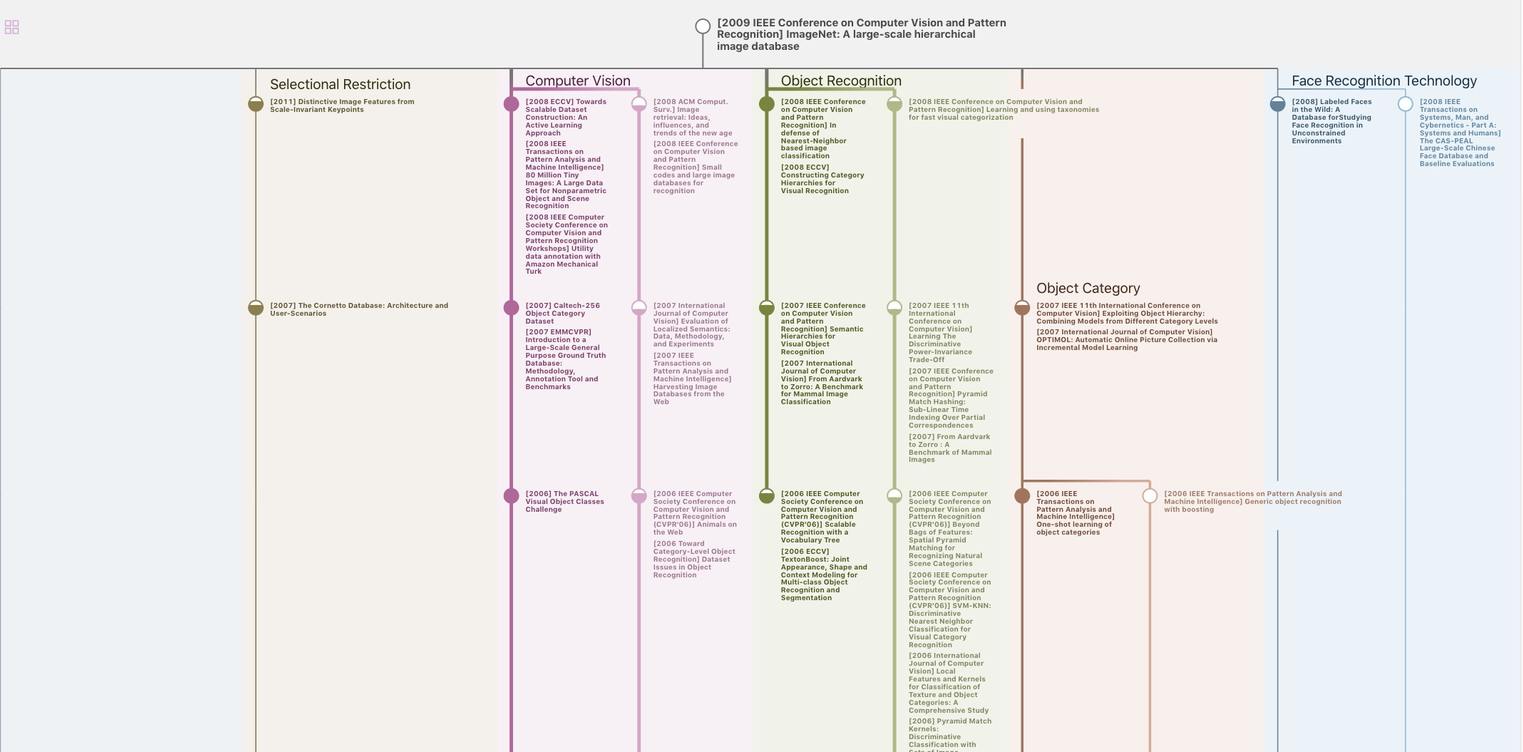
生成溯源树,研究论文发展脉络
Chat Paper
正在生成论文摘要