A theory-driven machine learning system for financial disinformation detection
PRODUCTION AND OPERATIONS MANAGEMENT(2022)
摘要
Maliciously false information (disinformation) can influence people's beliefs and behaviors with significant social and economic implications. In this study, we examine news articles on crowd-sourced digital platforms for financial markets. Assembling a unique dataset of financial news articles that were investigated and prosecuted by the Securities and Exchange Commission, along with the propagation data of such articles on digital platforms and the financial performance data of the focal firm, we develop a well-justified machine learning system to detect financial disinformation published on social media platforms. Our system design is rooted in the truth-default theory, which argues that communication context and motive, coherence, information correspondence, propagation, and sender demeanor are major constructs to assess deceptive communication. Extensive analyses are conducted to evaluate the performance and efficacy of the proposed system. We further discuss this study's theoretical implications and its practical value.
更多查看译文
关键词
fake news, financial disinformation, social media platform, theory-driven machine learning, truth-default theory
AI 理解论文
溯源树
样例
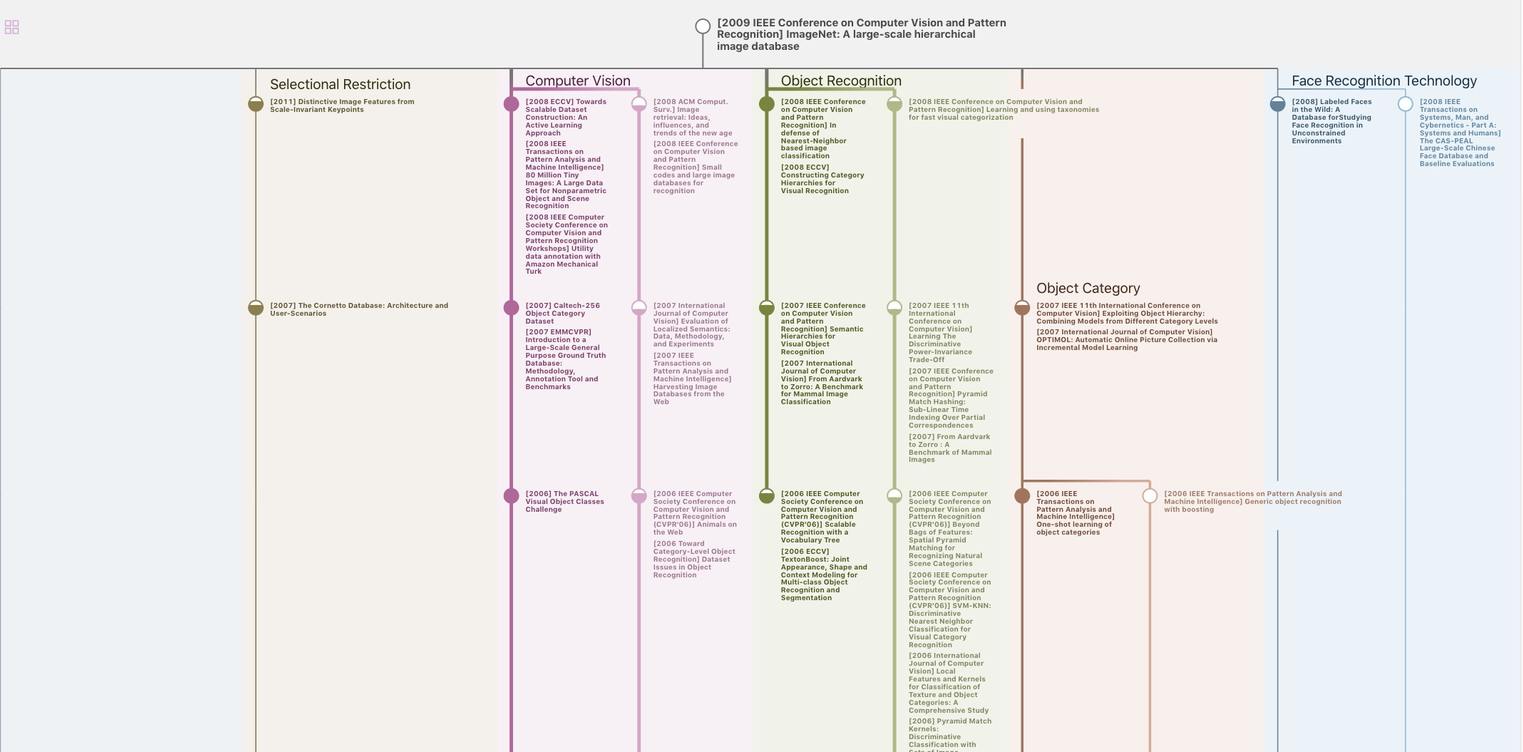
生成溯源树,研究论文发展脉络
Chat Paper
正在生成论文摘要