General Aviation Aircraft Identification at Non-Towered Airports Using a Two-Step Computer Vision-Based Approach
IEEE ACCESS(2022)
摘要
Aircraft identification in airport operations is critical to various applications, including airport planning and environmental studies. Previous research and commercially available systems heavily rely on recognizing aircraft tail numbers using text recognition. However, this approach alone does not provide accurate results in situations when the tail number visibility is reduced or obstructed. Furthermore, general aviation aircraft are harder to identify because they are small in size, and their tail numbers include substantial variations in fonts, sizes, and orientations. To tackle these issues, we propose a two-step computer vision-based aircraft identification method, which first identifies the aircraft type and then recognizes the tail number in a probabilistic multi-frame-based (MFB) framework. In the first step, a convolutional neural network (CNN)-based aircraft classifier is customized to decrease the search space in the registration database. In the second step, the identification process is finalized by integrating the text recognition results into the designed probabilistic MFB framework. The proposed method achieves approximately 90% identification accuracy when tested on video data collected from three general aviation airports. This is a significant improvement compared to text recognition alone, which recognizes 67% of the individual tail number characters.
更多查看译文
关键词
Aircraft, Tail, Airports, Text recognition, Aircraft manufacture, Atmospheric modeling, Air traffic control, Intelligent transportation systems, computer vision, airports, aircraft
AI 理解论文
溯源树
样例
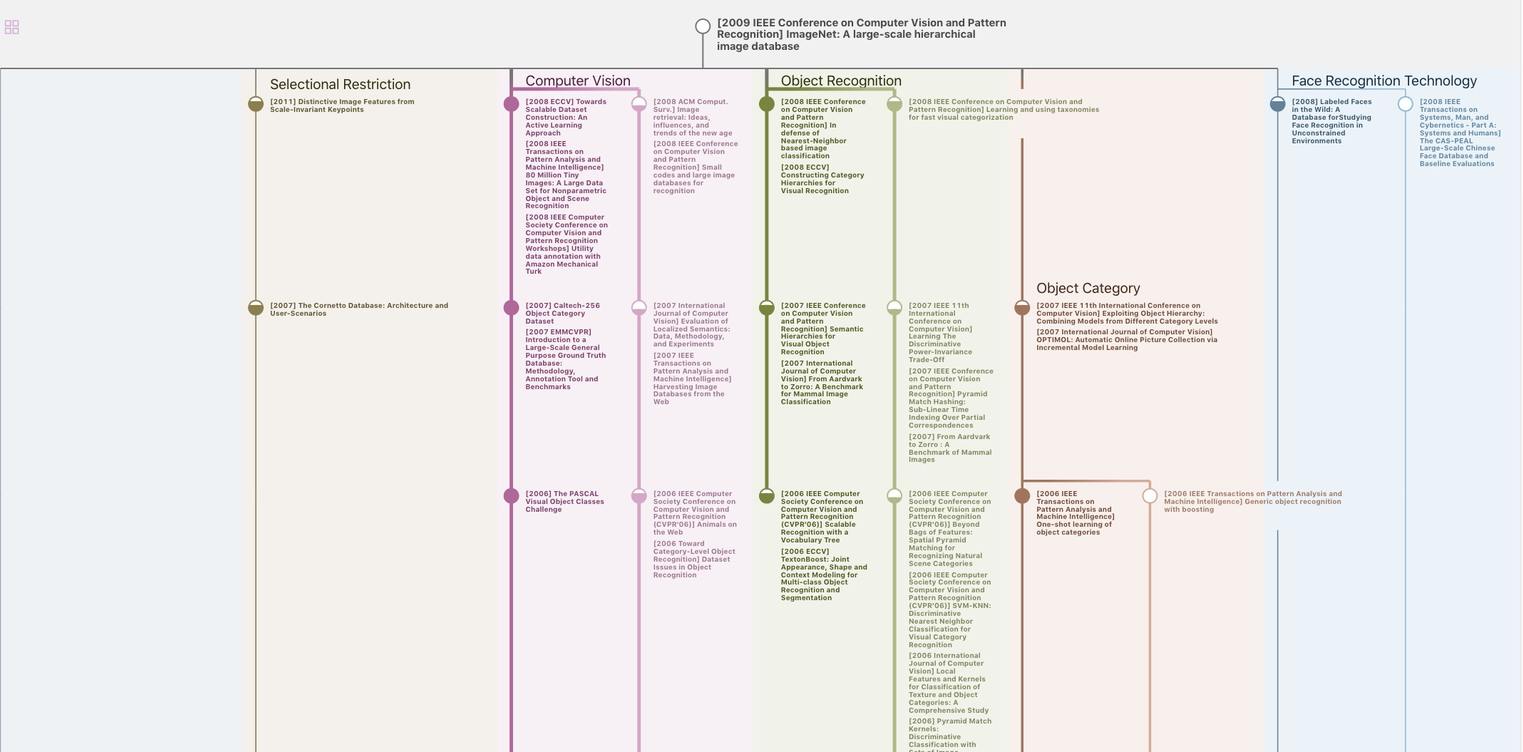
生成溯源树,研究论文发展脉络
Chat Paper
正在生成论文摘要