Graft: A graph based time series data mining framework
Engineering Applications of Artificial Intelligence(2022)
摘要
Rapid technology integration causes a high dimensional time series data accumulation in multiple domains and applying the classical data mining tools and techniques becomes a challenging task. Hence, the time series data representation have gained popularity over the years, which ease the task of mining, analysis and visualization. Graph based representation is one such emerging tool in which the time series data is represented as nodes and edges of graph. The current graph based representation is designed either to mine the motif or discords from a single time series or cluster the time series where each node represents a time series sample. Such representation technique causes information loss and also no further analysis could be performed other than clustering. To address these challenges, we propose a unique graph representation for time series dataset that works on multiple domains. Novelty of the graph representation is that it is unique for multiple time series and it acts as a framework for whole time series clustering, temporal pattern extraction from each cluster and temporally dependent rare event discovery. A new research direction for the proposed graph based framework is shown. Comparative analysis reveal the superiority of the proposed framework particularly as a clustering technique. The key contributions of the paper are: (i) transformation strategy of time series database from time domain to graph structure in topological domain (ii) time series clustering using path level analysis (iii) identification of temporally dependent co-occurring patterns (iv) rare event detection using component level analysis
更多查看译文
关键词
Clustering,Time series similarity,Graph based networks,Directed and undirected graphs,Eigen values
AI 理解论文
溯源树
样例
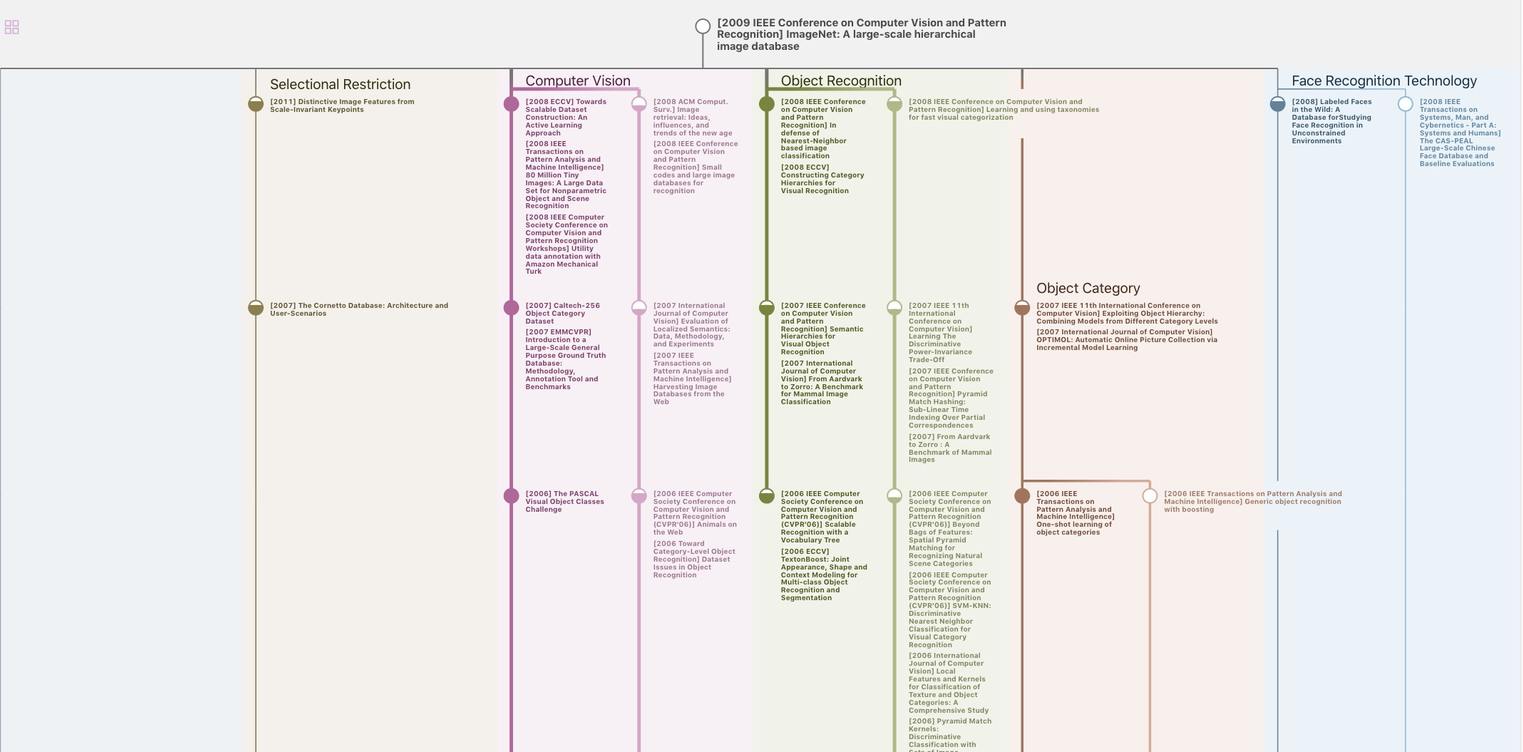
生成溯源树,研究论文发展脉络
Chat Paper
正在生成论文摘要