In-process comprehensive prediction of bead geometry for laser wire-feed DED system using molten pool sensing data and multi-modality CNN
The International Journal of Advanced Manufacturing Technology(2022)
摘要
For wire-feed laser additive manufacturing (WLAM), the build geometrical parameters are one of the indicators of build quality; thus, it is crucial to monitor the geometrical parameters in real-time for quality assurance. However, the current research and development for in situ geometry monitoring are in the early phase due to interweaved correlation of the sensing data and their comprehensive effects on the bead geometry, as well as the high characterization cost to model these effects. This paper focuses on using machine learning techniques to enable in-process geometry monitoring by comprehensively modeling the correlation between the real-time molten pool sensing data and bead geometry properties. A deep learning-based multi-modality convolutional neural network (m-CNN) is trained to take the molten pool image and thermal profile as the input to comprehensively estimate the geometric properties of the build bead. The network is configured by the hyperparameter optimization process and experimentally validated by the real-time molten pool sensing data collected on a wire-feed laser additive manufacturing (AM) system. The effect of using the temperature data from the leading, center, and tailing positions of the molten pool on the prediction performance of the CNN model is studied and analyzed. The CNN model’s performance is compared with a support vector regression model for comparison. The developed model represents an in-process monitoring framework for real-time estimation of post-processing bead geometric properties and takes a step towards developing in situ quality control strategy for the metal AM system.
更多查看译文
关键词
Wire-feed laser additive manufacturing (WLAM),Convolutional neural network (CNN),Molten pool,Property,Quality control
AI 理解论文
溯源树
样例
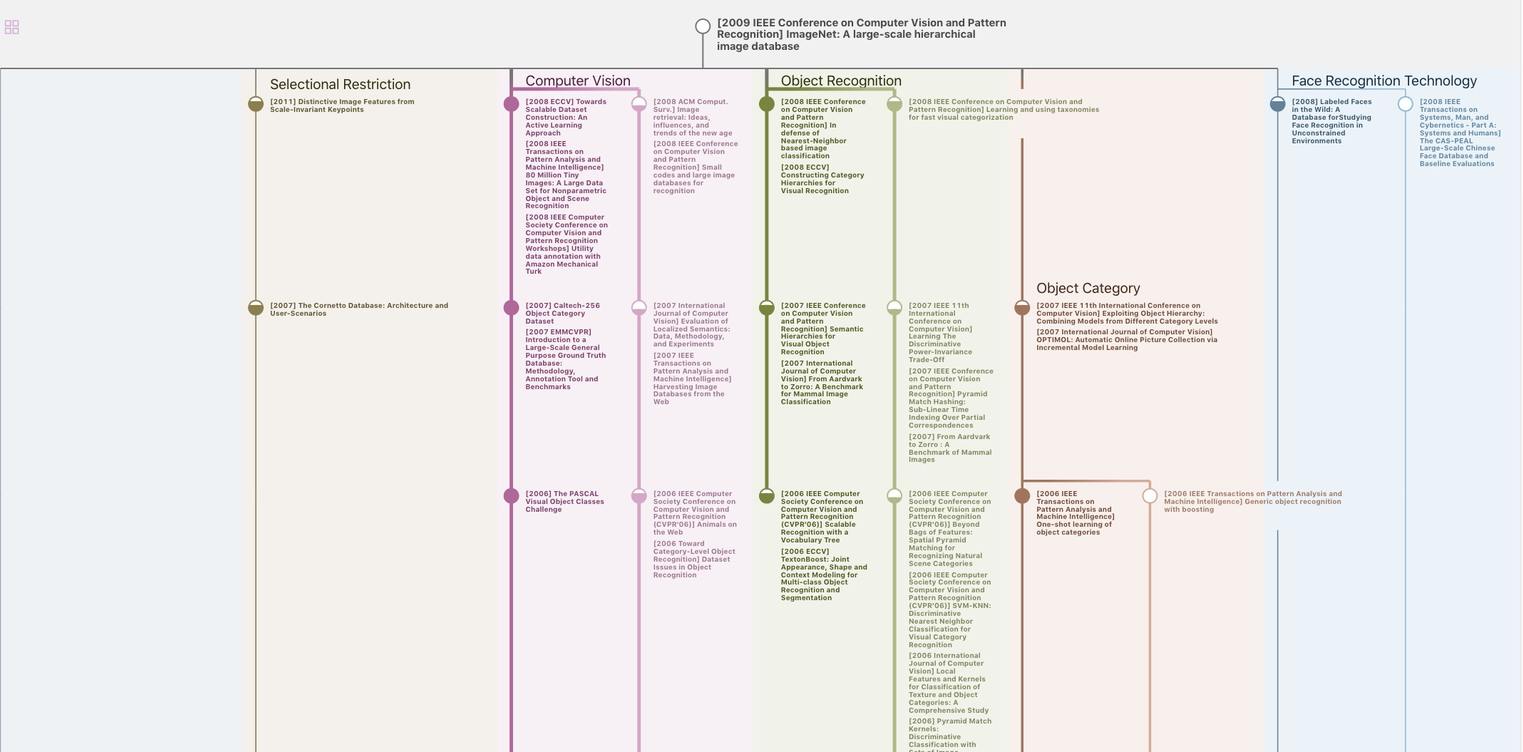
生成溯源树,研究论文发展脉络
Chat Paper
正在生成论文摘要