Simple Multiscale UNet for Change Detection With Heterogeneous Remote Sensing Images
IEEE GEOSCIENCE AND REMOTE SENSING LETTERS(2022)
摘要
Change detection with heterogeneous remote sensing images (HRSIs) is attractive for observing the Earth's surface when homogeneous images are unavailable. However, HRSIs cannot be compared directly because the imaging mechanisms for bitemporal HRSIs are different, and detecting change with HRSIs is challenging. In this letter, a simple yet effective deep learning approach based on the classical UNet is proposed. First, a pair of image patches are concatenated together to learn a shared abstract feature in both image patch domains. Then, a multiscale convolution module is embedded in a UNet backbone to cover the various sizes and shapes of ground targets in an image scene. Finally, a combined loss function, which incorporates the focal and dice losses with an adjustable parameter, was incorporated to alleviate the effect of the imbalanced quantity of positive and negative samples in the training progress. By comparisons with five state-of-the-art methods in three pairs of real HRSIs, the experimental results achieved by our proposed approach have the best overall accuracy (OA), average accuracy (AA), recall (RC), and F-Score that are more than 95%, 79%, 60%, and 61%, respectively. The quantitative results and visual performance indicated the feasibility and superiority of the proposed approach for detecting land cover change with HRSIs.
更多查看译文
关键词
Feature extraction, Optical sensors, Optical imaging, Shape, Training, Remote sensing, Convolution, Deep learning neural network, hetereogreous remote sensing images, land cover change detection, multi-scale feature
AI 理解论文
溯源树
样例
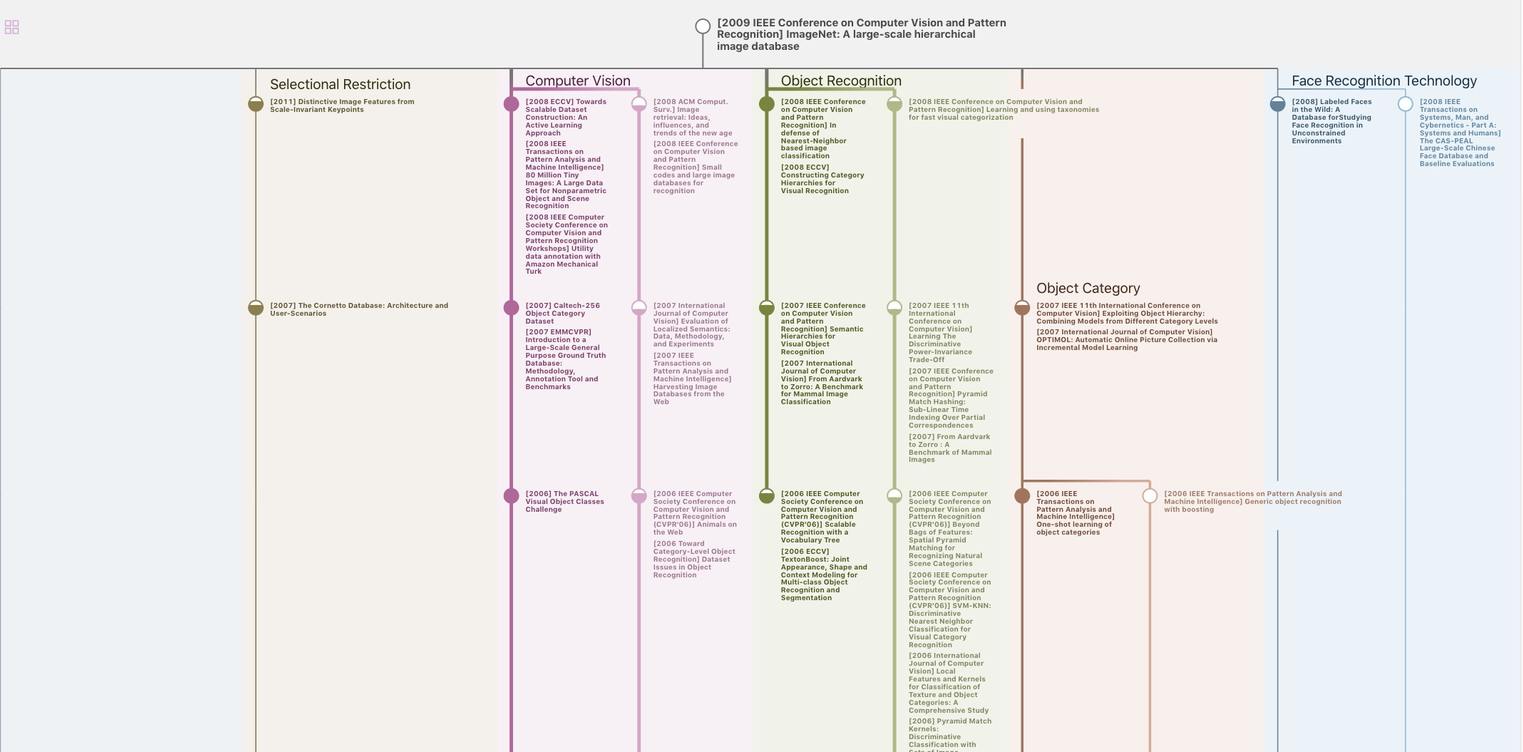
生成溯源树,研究论文发展脉络
Chat Paper
正在生成论文摘要