Forecasting strong subsequent earthquakes in California clusters by machine learning
Physics of the Earth and Planetary Interiors(2022)
摘要
In this paper, we propose an innovative machine learning approach called NESTORE, which analyses seismic clusters to forecast strong earthquakes of magnitudes similar or greater to those of the mainshock. The method analyzes the seismicity in the first hours/days after the mainshock and provides the probability of having a strong subsequent earthquake. The analysis is conducted at various stages of time to simulate the increase in knowledge over time. We address the main problem of statistics and machine learning when applied to spatiotemporal variation of seismicity: the small datasets available, on the order of tens or fewer instances, need a more accurate analysis with respect to the classical testing procedures, where hundreds or thousands of data are available. In addition, we develop a more robust NESTORE method based on a jackknife approach (rNESTORE), and we successfully apply it to California seismicity.
更多查看译文
关键词
Largest aftershock,Seismicity,Machine learning,Bath's law,Aftershock sequence,California seismicity
AI 理解论文
溯源树
样例
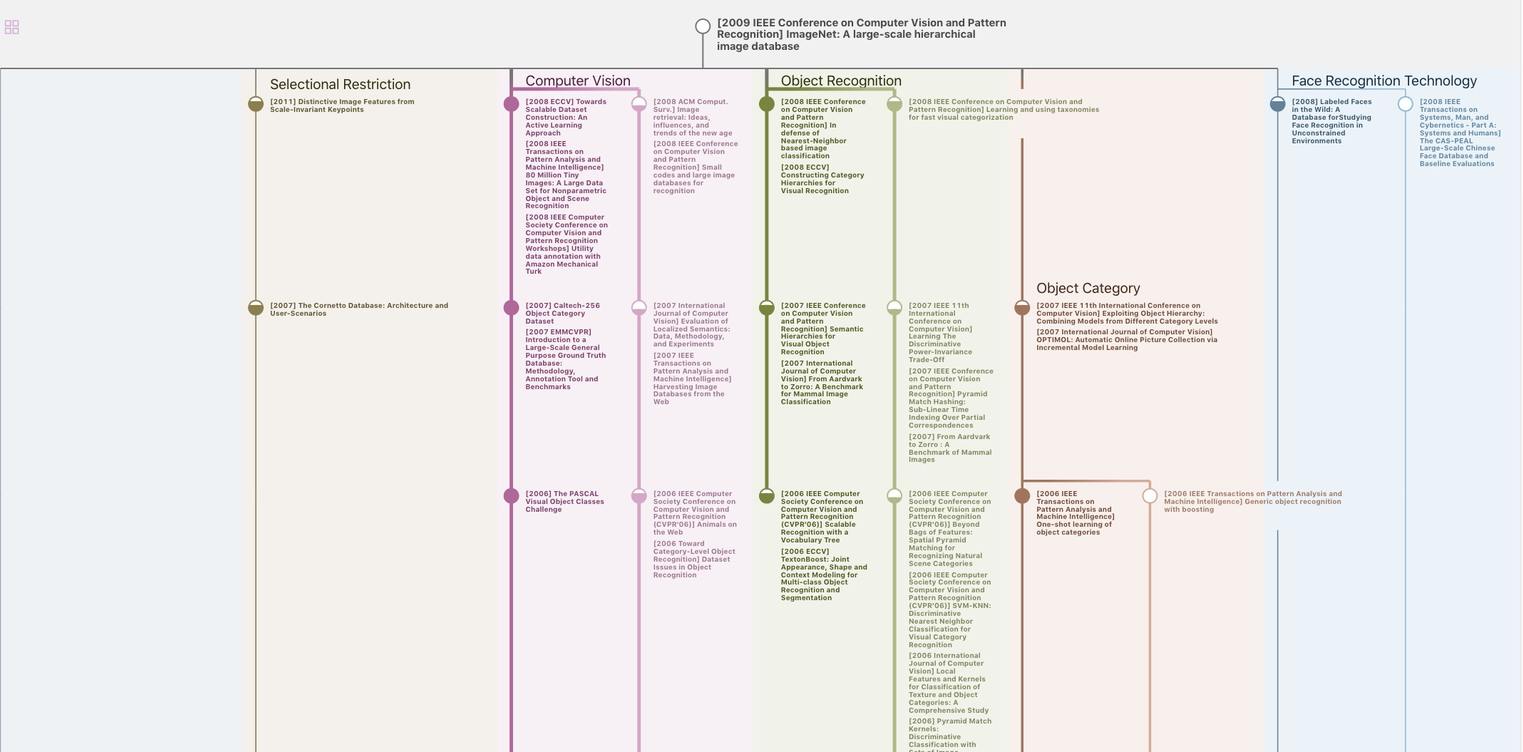
生成溯源树,研究论文发展脉络
Chat Paper
正在生成论文摘要