Arrhythmia detection based on multi-scale fusion of hybrid deep models from single lead ECG recordings: A multicenter dataset study
BIOMEDICAL SIGNAL PROCESSING AND CONTROL(2022)
摘要
Background: Electrocardiogram automated arrhythmia detection plays a crucial role in the early prevention and diagnosis of cardiovascular diseases. However, previous research relies on noise removal algorithms and extracting solid features from raw ECGs. Besides, existing heartbeat classifiers ignore underlying complementary information of various scales, and intra-patient paradigms often lead to biased results.Methods: We constructed a novel end-to-end Multi-Scale Convolutional Neural Network-Sequence to Sequence architecture for heartbeat classification to address these issues. We have verified this approach on the clinical data collected by wearable devices and two heterogeneous datasets.Results: The proposed model can effectively capture multi-granularity frequency and longitudinal temporal in-formation by fusion representation and sequence learning. The overall F1 score of our approach was achieved at 99.57%, which exceeded the reference pure cascade model by 4.36%.
更多查看译文
关键词
Arrhythmia detection, Deep learning, Multi-scale temporal fusion, Sequence to Sequence Network
AI 理解论文
溯源树
样例
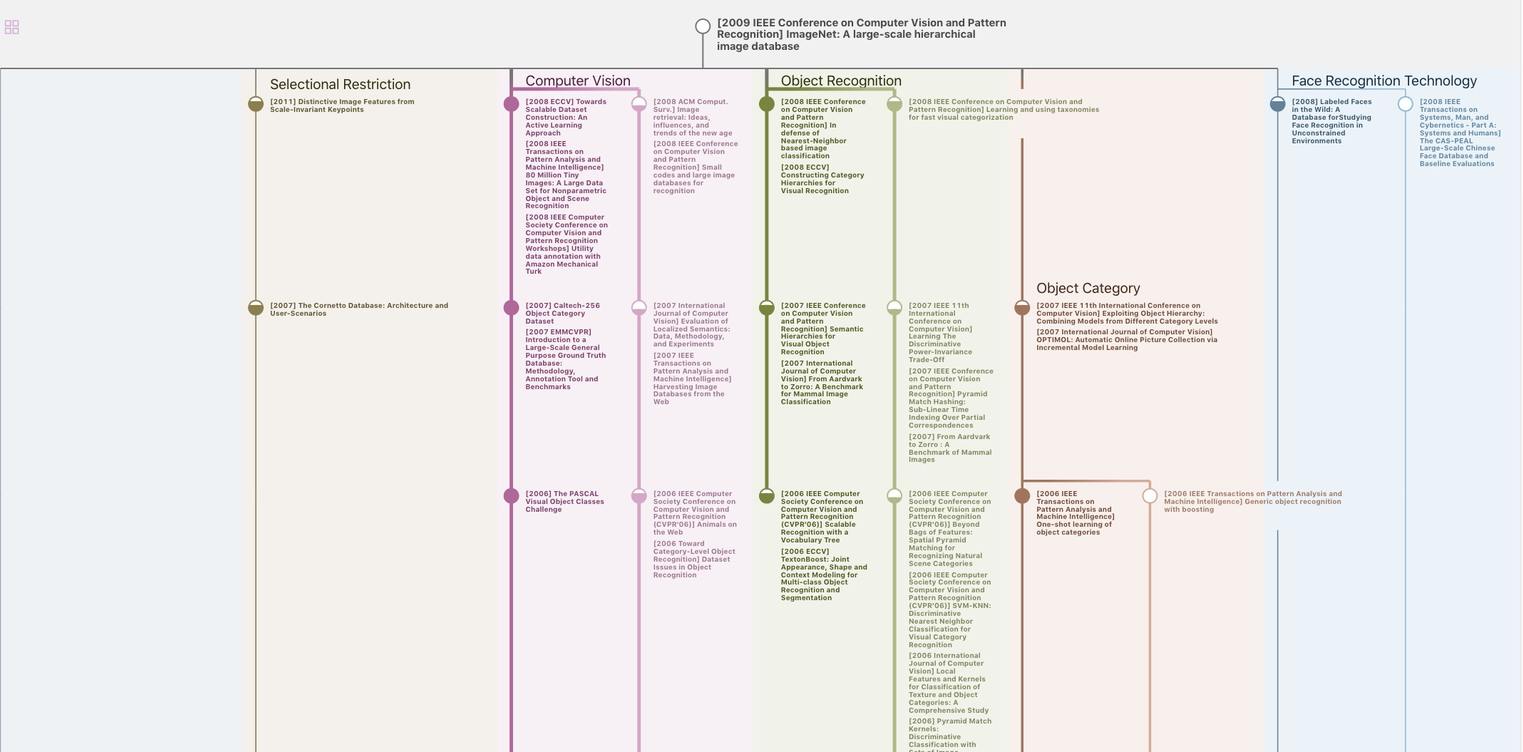
生成溯源树,研究论文发展脉络
Chat Paper
正在生成论文摘要