A genetic programming approach for real-time crash prediction to solve trade-off between interpretability and accuracy
JOURNAL OF TRANSPORTATION SAFETY & SECURITY(2023)
摘要
Real-time crash risk prediction is a hot topic of emerging technology. Due to the lack of basic risk formation theory, previous studies focussed on the application of complex models to improve the accuracy of prediction, ignoring the interpretation of variables, while the traditional statistical analysis method can interpret variables, but the prediction accuracy is poor, which falls into a dilemma of trade-off. In this study, based on the traffic flow information of elevated expressway, an improved genetic programming (GP) approach with elite gene bank is applied to obtain an explicit traffic flow crash risk function to solve the above trade-off problem. Logistic regression and backward-propagation neural network combined with partial dependency plot were used as baseline methods to examine the interpretability and accuracy of GP. It is found that GP prediction model has been proved to be able to select important variables and solve the trade-off dilemma, which has good interpretability and accuracy. The results show that crash risk in the traffic flow mainly comes from the traffic volume, speed of the upstream section, and the speed of the current section. Furthermore, the error of GP comes from the unobserved heterogeneity and crash mechanism theory is proposed.
更多查看译文
关键词
real-time crash risk prediction, traffic flow, genetic programming, interpretability, crash mechanism
AI 理解论文
溯源树
样例
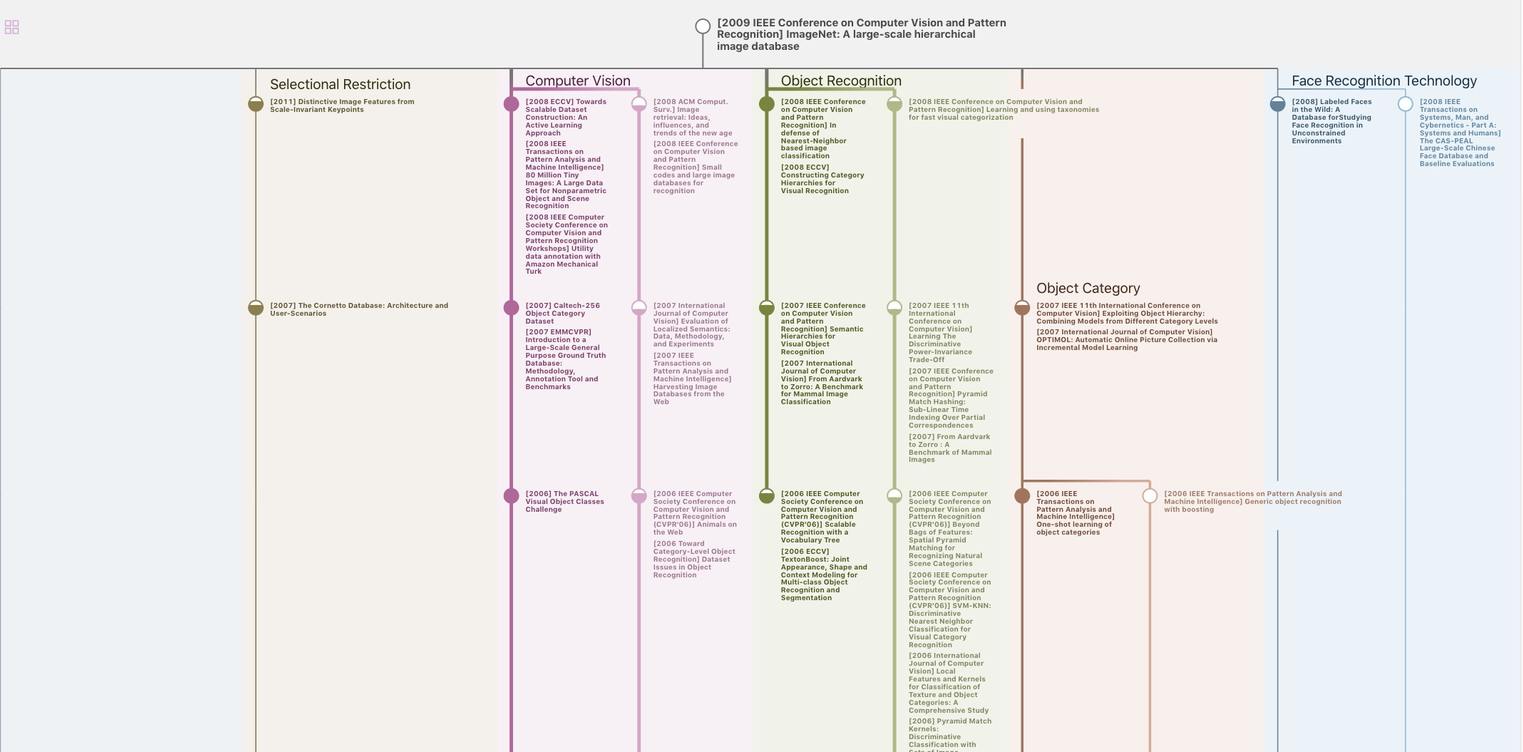
生成溯源树,研究论文发展脉络
Chat Paper
正在生成论文摘要