Sequential Clustering and Classification Approach to Analyze Sales Performance of Retail Stores Based on Point-of-Sale Data
INTERNATIONAL JOURNAL OF INFORMATION TECHNOLOGY & DECISION MAKING(2022)
摘要
Point-of-Sale (POS) data analysis is usually used to explore sales performance in business commence. This manuscript aims to combine unsupervised clustering and supervised classification methods in an integrated data analysis framework to analyze the real-world POS data. Clustering method, which is performed on sales dataset, is used to cluster the stores into several groups. The clustering results, data labels, are then combined with other information in store features dataset as the inputs of the classification model which classifies the clustering labels by using store features dataset. Non-dominated sorting generic algorithm-II (NSGA-II) is applied in the framework to employ the multi-objective of clustering and classification. The experimental case study shows clustering results can reveal the hidden structure of sales performance of retail stores while classification can reveal the major factors that effect to the sales performance under different group of retail stores. The correlations between sales clusters and the store information can be obtained sequentially under a series of data analysis with the proposed framework.
更多查看译文
关键词
Data Clustering, data classification, multi-objective optimization, non-dominated sorting genetic algorithm, point of sale data
AI 理解论文
溯源树
样例
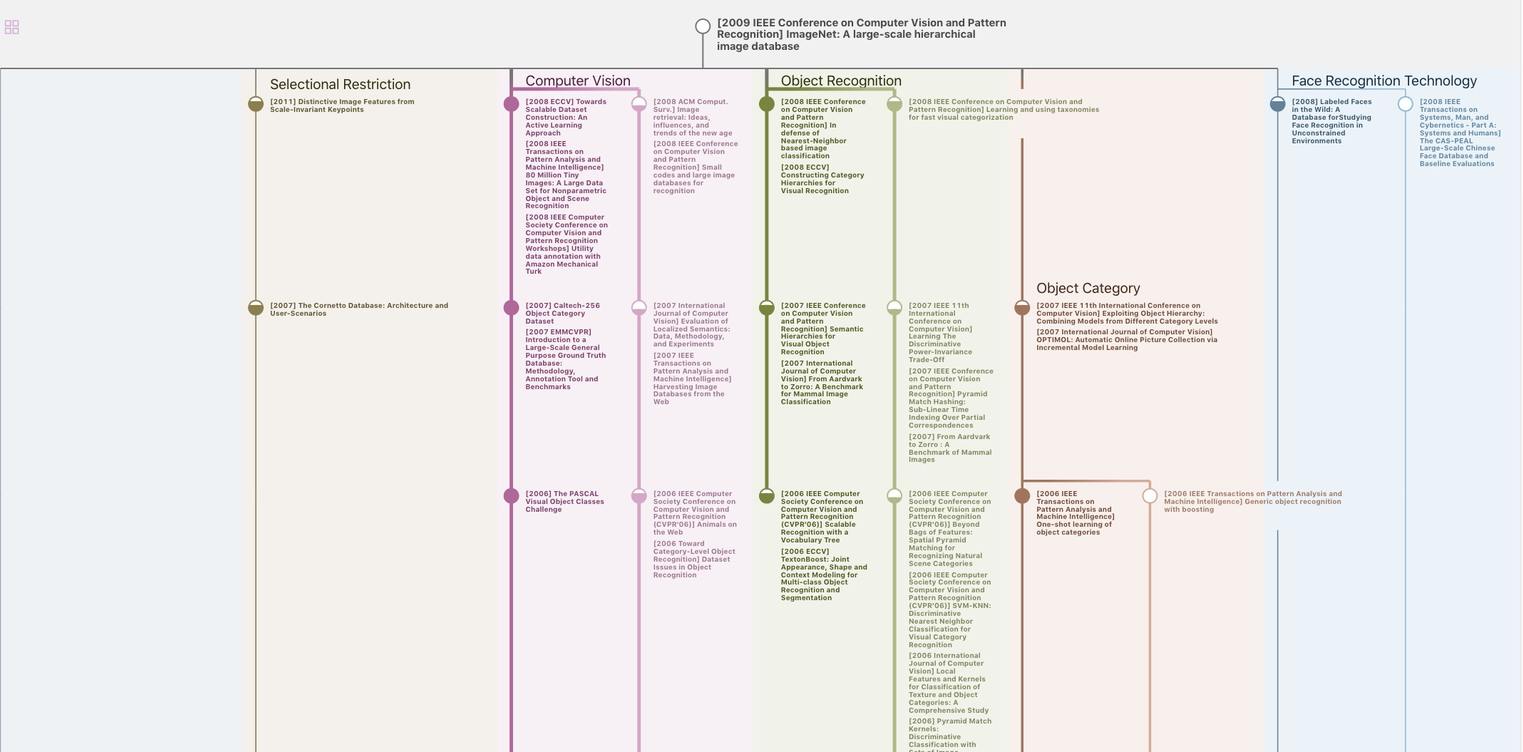
生成溯源树,研究论文发展脉络
Chat Paper
正在生成论文摘要