Usefulness of Automatic Hyperparameter Optimization in Developing Radiation Emulator in a Numerical Weather Prediction Model
ATMOSPHERE(2022)
摘要
To improve the forecasting accuracy of a radiation emulator in a weather prediction model over the Korean peninsula, the learning rate used in neural network training was automatically optimized using the Sherpa. The Sherpa experiment results were compared with two control simulation results using learning rates of 0.0001 and 1 for different batch sizes (full to 500). In the offline evaluation, the Sherpa results showed significant improvements in predicting longwave/shortwave heating rates and fluxes compared to the lowest learning rate results, whereas the improvements compared to the highest learning rate were relatively small because the optimized values by the Sherpa were 0.4756-0.6656. The online evaluation results over one month, which were linked with the weather prediction model, demonstrated the usefulness of Sherpa on a universal performance for the radiation emulator. In particular, at the full batch size, Sherpa contributed to reducing the one-week forecast errors for longwave/shortwave fluxes, skin temperature, and precipitation by 39-125%, 137-159%, and 24-26%, respectively, compared with the two control simulations. Considering the widespread use of parallel learning based on full batch, Sherpa can contribute to producing robust results regardless of batch sizes used in neural network training for developing radiation emulators.
更多查看译文
关键词
Sherpa, WRF, radiation, emulator, neural network
AI 理解论文
溯源树
样例
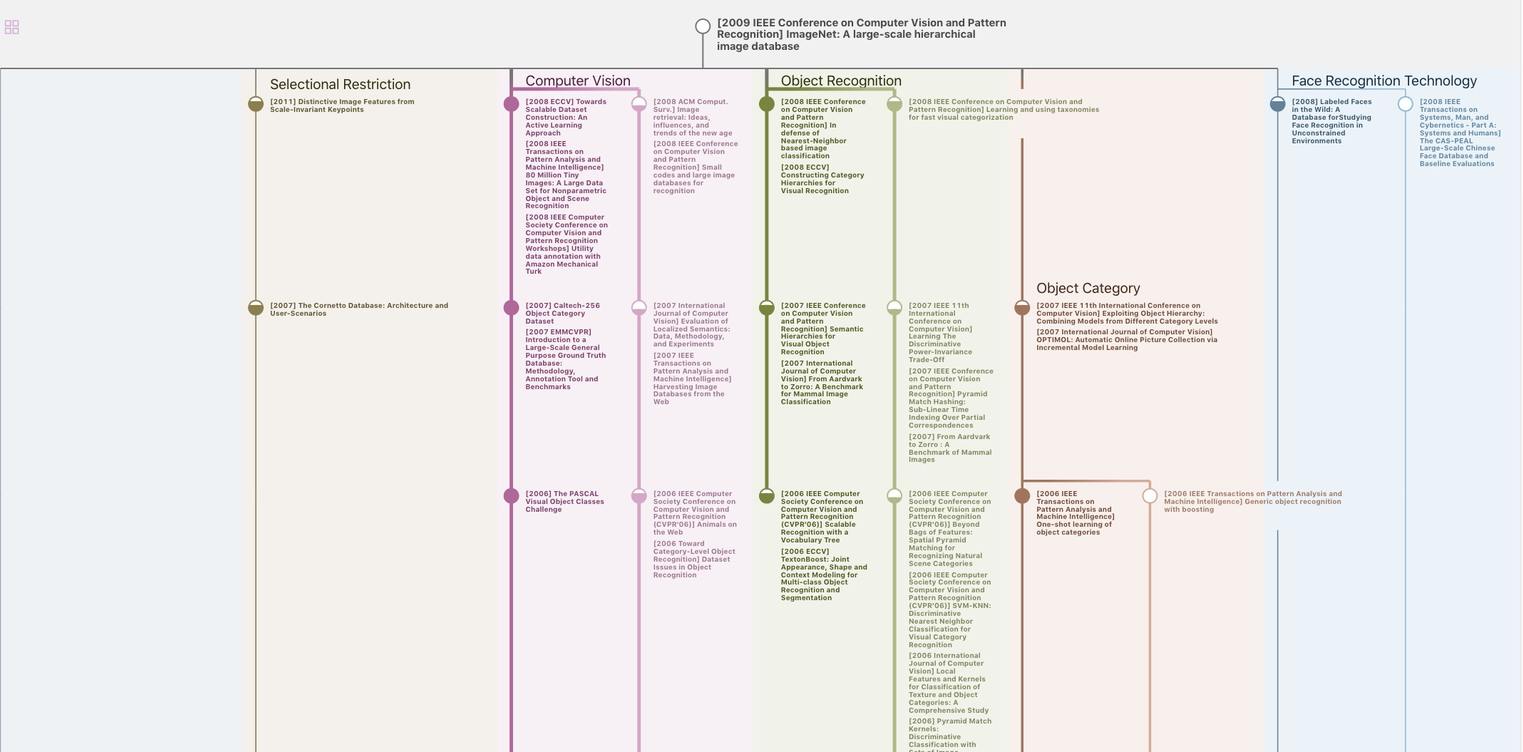
生成溯源树,研究论文发展脉络
Chat Paper
正在生成论文摘要