Heterogeneous Axonal Delay Improves the Spiking Activity Propagation on a Toroidal Network
COGNITIVE COMPUTATION(2022)
摘要
Several studies have looked into how noise affects neural networks and actual brains as evidenced by transcranial random noise stimulation, which improves cognitive performance. This research aims to broaden this understanding by concentrating on the network structural heterogeneity realized by adding noise to a neural model network’s axonal propagation delay. We utilized the pyNEST neural network simulator to model a network of 400 artificial Izhikevich neurons connected by a folded von Neumann neighborhood to form a toroidal shape where axonal propagation noise simulates a variable spatial spacing between neurons. In this network only one neuron is regularly spiking at first because it is specifically stimulated by a 10mA external current, while all the other neurons have no external input and are stimulated solely by the activity of their neighbors. The forward propagation of the spiking wave from the original neuron to its neighbors, and then to distant nodes on the toroidal network, was investigated. For each simulation, we recorded the activity of all the network changing several parameters to verify differences of spike activity in different positions on the torus. By manipulating heterogeneity, we discovered that adding noise helps the signal reach distant neurons in 20% less time, compared to when there is no heterogeneity. We demonstrated for the first time that structural heterogeneity in a neural network can favor the propagation of spiking waves. This result is in line with other findings that suggest that a certain level of noise is good for the brain, extending this concept to the network physical structure.
更多查看译文
关键词
Noise delay, Spike, Stochastic resonance
AI 理解论文
溯源树
样例
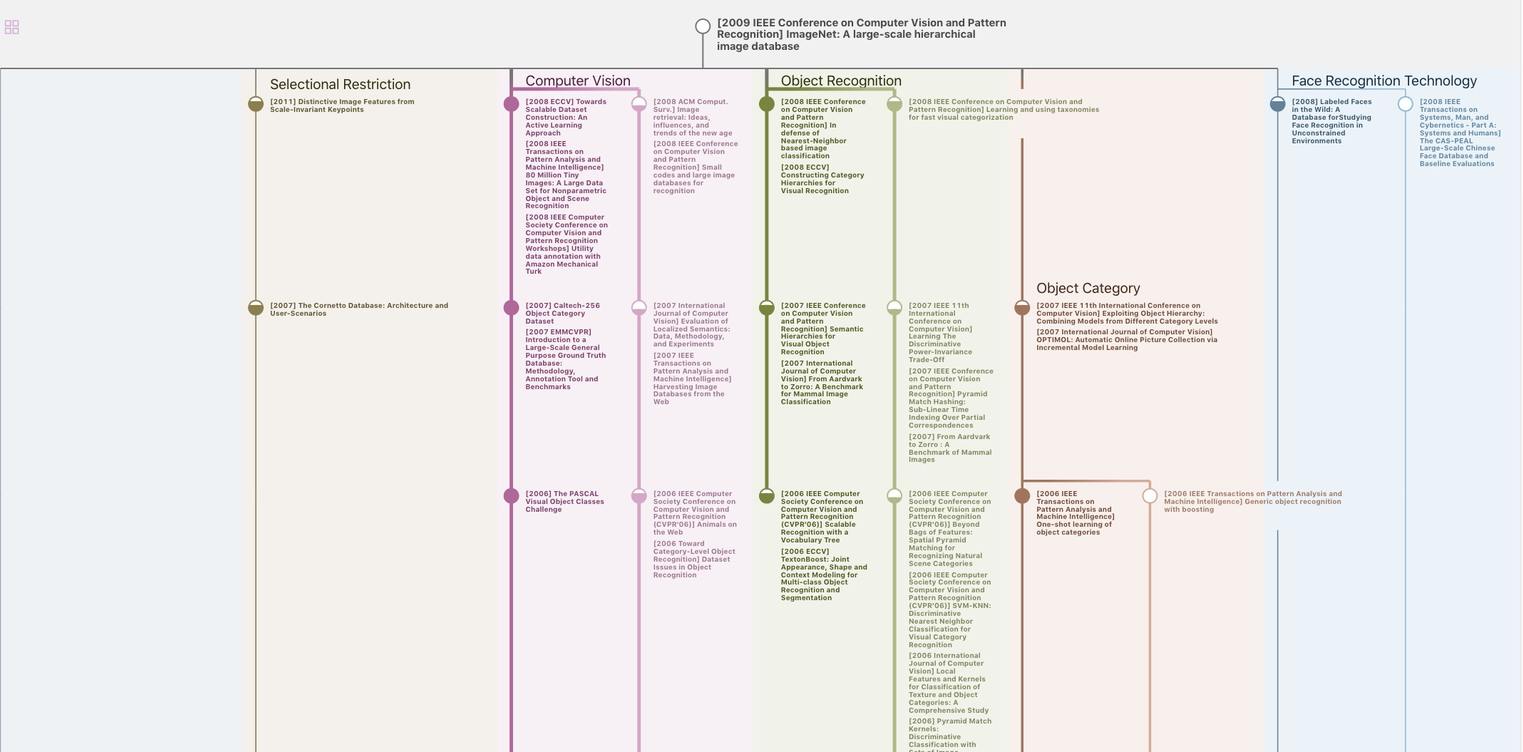
生成溯源树,研究论文发展脉络
Chat Paper
正在生成论文摘要