HDBRR: a statistical package for high-dimensional Bayesian ridge regression without MCMC
JOURNAL OF STATISTICAL COMPUTATION AND SIMULATION(2022)
摘要
Ridge regression dealswith collinearity in the homoscedastic linear regression model. When the number of predictors (p) is much larger than the number of observations (n), it gives unique least-square estimators. From both, classical and Bayesian approaches, parameter estimation is a highly demanding computational task, in the first one being an optimization problem and in the second one a high-dimensional integration problem usually faced up through Markov chain Monte Carlo (MCMC). The main drawback of MCMC is the practical impossibility of checking convergence to the posterior distribution, which is commonly very slow due to the large number of regression parameters. Here, a computational algorithm is proposed to obtain posterior estimates of regression parameters, variance components and predictions for the conventional ridge regression model. The algorithm is based on a reparametrization of the model which allows us to obtain the marginal posterior means and variances by integrating out a nuisance parameter whose marginal posterior is defined on the open interval (0, 1).
更多查看译文
关键词
Bayesian methods, regression, variable selection, shrinkage, ridge regression, MCMC, R
AI 理解论文
溯源树
样例
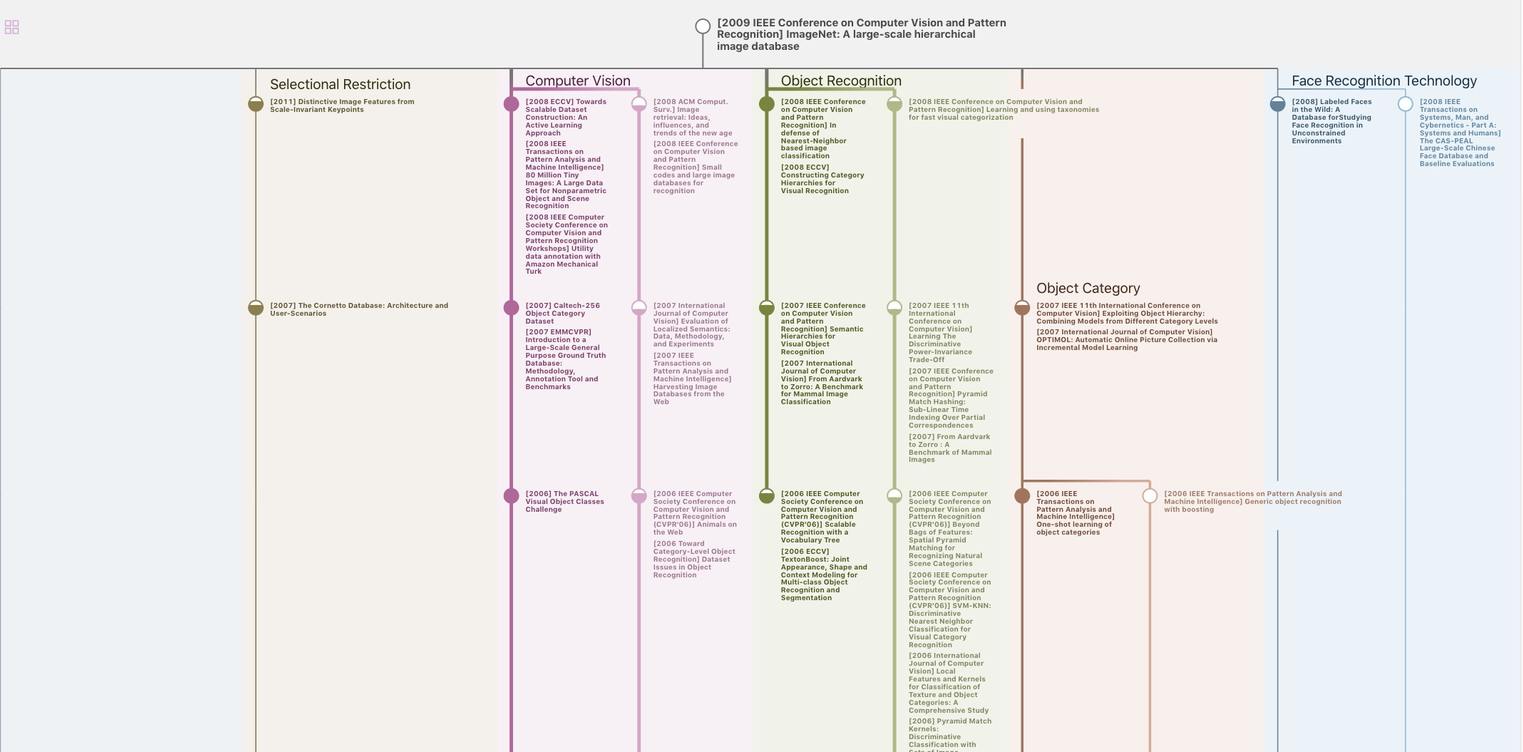
生成溯源树,研究论文发展脉络
Chat Paper
正在生成论文摘要