A Power Spectrum Pattern Difference-Based Time-Frequency Sub-Band Selection Method for MI-EEG Classification
IEEE Sensors Journal(2022)
摘要
Motor imagery-based brain-computer interface (MI-BCI) is considered to be the most promising technology, which can help patients with muscle disorders to carry out rehabilitation training and assist in activities of daily living. However, the time window and frequency band of the ERD/ERS pattern activated by motor imagery vary from person to person. In this study, we propose a power spectrum pattern difference-based time-frequency sub-band selection (PSPD) method, which can further improve the classification performance of MI EEG. To better analyze the sensorimotor rhythm, we divide the EEG channels and extract the average and maximum pattern difference of the power spectrum according to the division. Then, the difference metric of the power spectrum pattern difference of inter-sample sets is calculated by dynamic time warping distance, and the consistency metric of the power spectrum pattern difference of intra-sample sets is calculated by mean pooling, and the optimal time-frequency sub-band is selected based on these two metrics. Finally, the optimal time-frequency sub-bands are respectively used for the common spatial pattern feature extraction and the linear kernel support vector machine classification. The proposed PSPD method achieves an average classification accuracy of 84.51%, 84.10% and 73.21% in BCI Competition IV Dataset IIa, Dataset IIb and OpenBMI dataset, respectively. Compared with the state-of-the-art methods, the PSPD method achieves the highest classification accuracy and good stability on three datasets. Excellent experimental results show that the PSPD method has great potential in MI-BCI’s motion intention decoding.
更多查看译文
关键词
Brain–computer interface (BCI),electroencephalogram (EEG),motor imagery (MI),time-frequency selection,power spectrum pattern difference
AI 理解论文
溯源树
样例
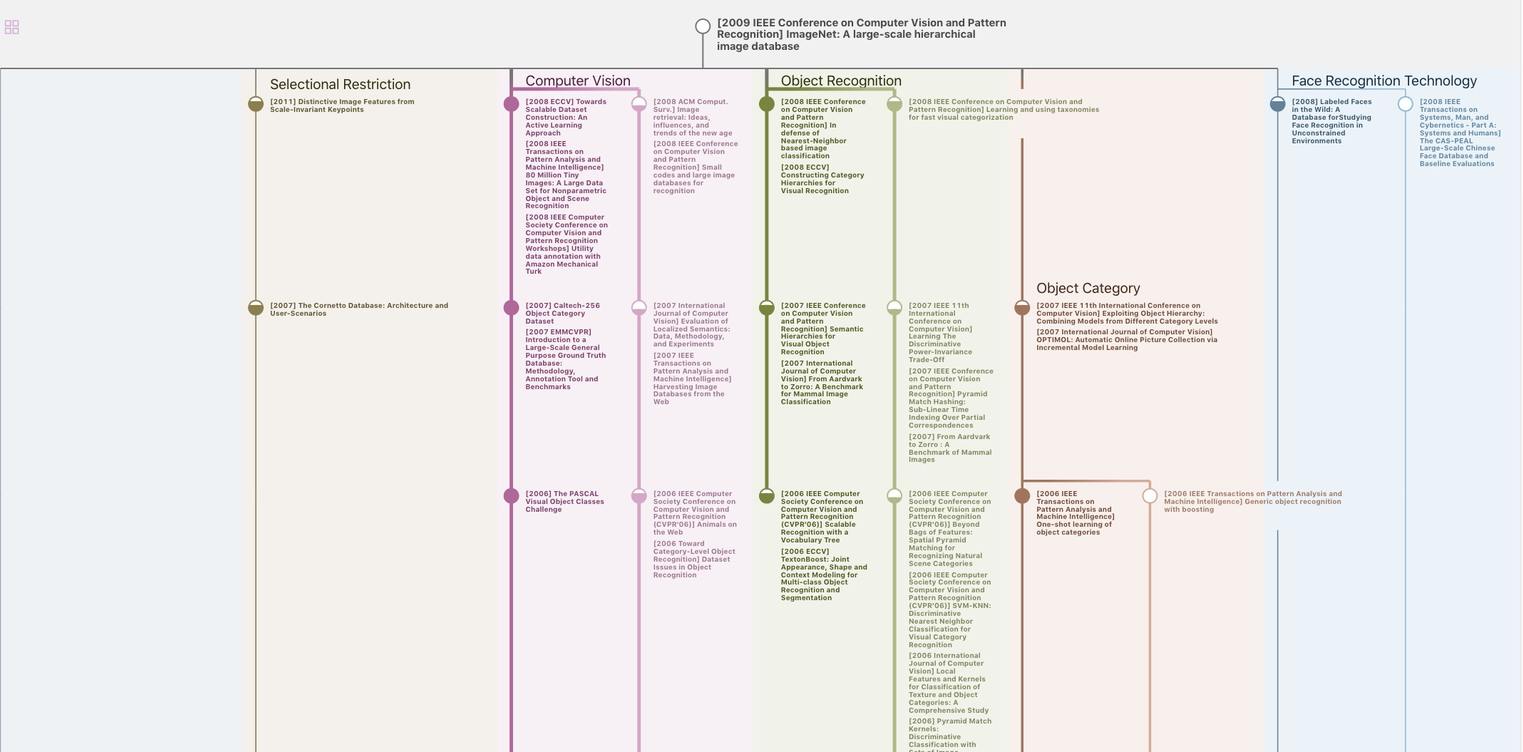
生成溯源树,研究论文发展脉络
Chat Paper
正在生成论文摘要