Transformer fault diagnosis method based on IFCM-DNN adjudication network
INTERNATIONAL CONFERENCE ON INTELLIGENT TRAFFIC SYSTEMS AND SMART CITY (ITSSC 2021)(2022)
摘要
At present, the transformer fault diagnosis methods based on clustering and neural network generally ignore the influence of unbalanced data sets, which could lead the model fall into local optimum. In order to improve the accuracy of transformer fault diagnosis with unbalanced data as samples, a decision network based on improved fuzzy clustering and deep neural network is proposed. A distance correction term is introduced to modify the fuzzy membership function, improve the membership relationship of the boundary data to majority class cluster, and reduce the influence of the wrong division of the boundary data on the cluster center of a minority one, so that the cluster center tends to be stable in the ideal position, and the imbalanced data set is effectively divided, the resolution of data set is improved. In addition, referring to the process of human learning and voting activities, a DNN adjudication network is established after the IFCM. Through learning the partitioned data sets to different degrees, the common network and expert network are used for joint voting, so as to obtain more reliable prediction results. The experimental results show that this method can effectively improve the accuracy of transformer fault diagnosis under unbalanced data sets.
更多查看译文
关键词
transformer, fault diagnosis, unbalanced data set, improved fuzzy clustering, deep neural network
AI 理解论文
溯源树
样例
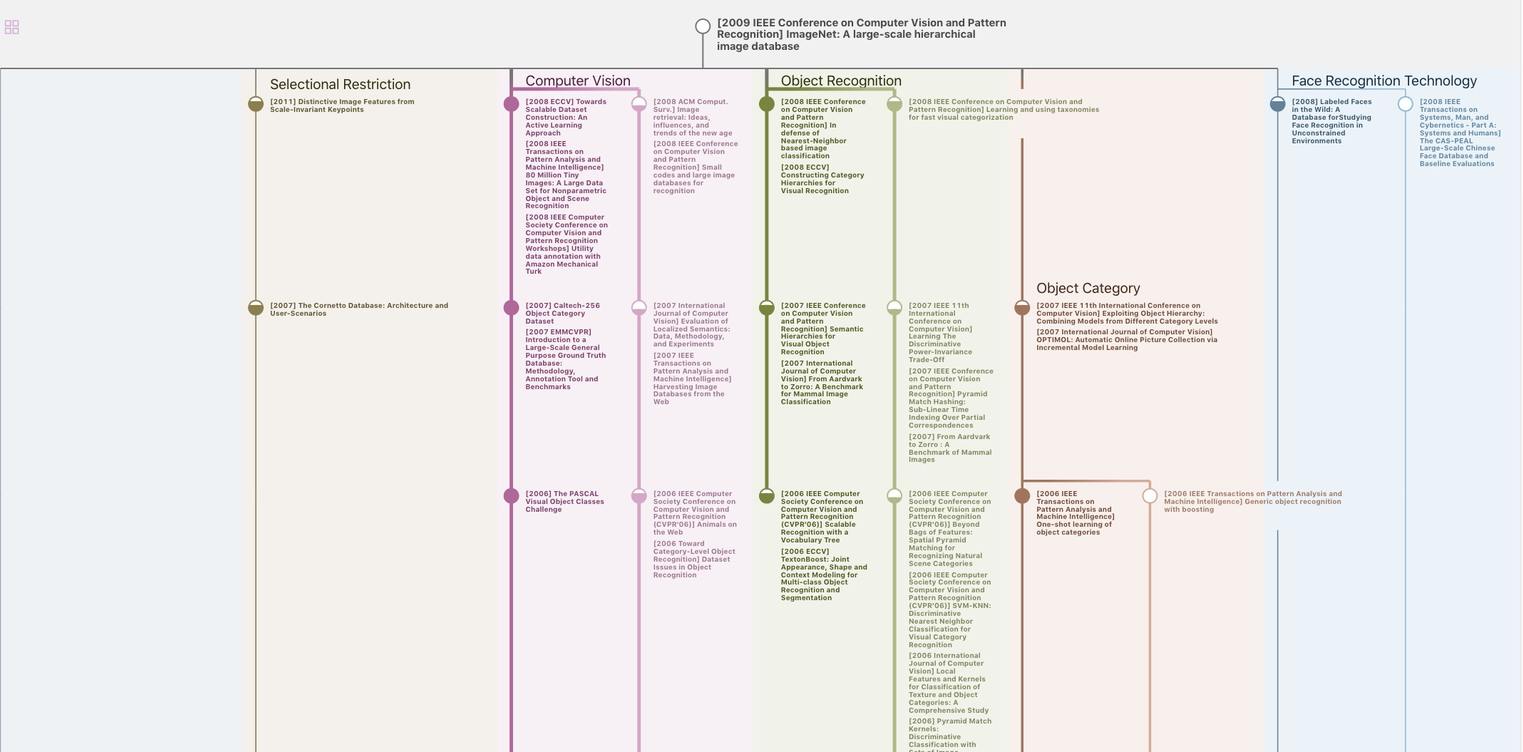
生成溯源树,研究论文发展脉络
Chat Paper
正在生成论文摘要