Hyperspectral Image Classification Based on Graph Transformer Network and Graph Attention Mechanism
IEEE GEOSCIENCE AND REMOTE SENSING LETTERS(2022)
摘要
Graph convolutional networks (GCNs) have begun to show their potential in hyperspectral image (HSI) classification in recent years. However, most of the current GCN methods are designed to learn node features on fixed and homogeneous graphs, and it is difficult for them to learn effective node features on heterogeneous graphs. The limitation is particularly evident in hyperspectral classification because of the different types of nodes and edges. The graph transformer network with the graph attention mechanism (GTN-A) is proposed to address this shortcoming in this letter. It can generate a new graph structure, which is represented by a more useful meta-path, so that node features can be better aggregated. The experiments conducted on two benchmark datasets illustrate the effectiveness of our method.
更多查看译文
关键词
Hyperspectral imaging, Convolution, Image edge detection, Transformers, Classification algorithms, Image segmentation, Feature extraction, Graph convolution network, graph transformer, hyperspectral image (HSI) classification
AI 理解论文
溯源树
样例
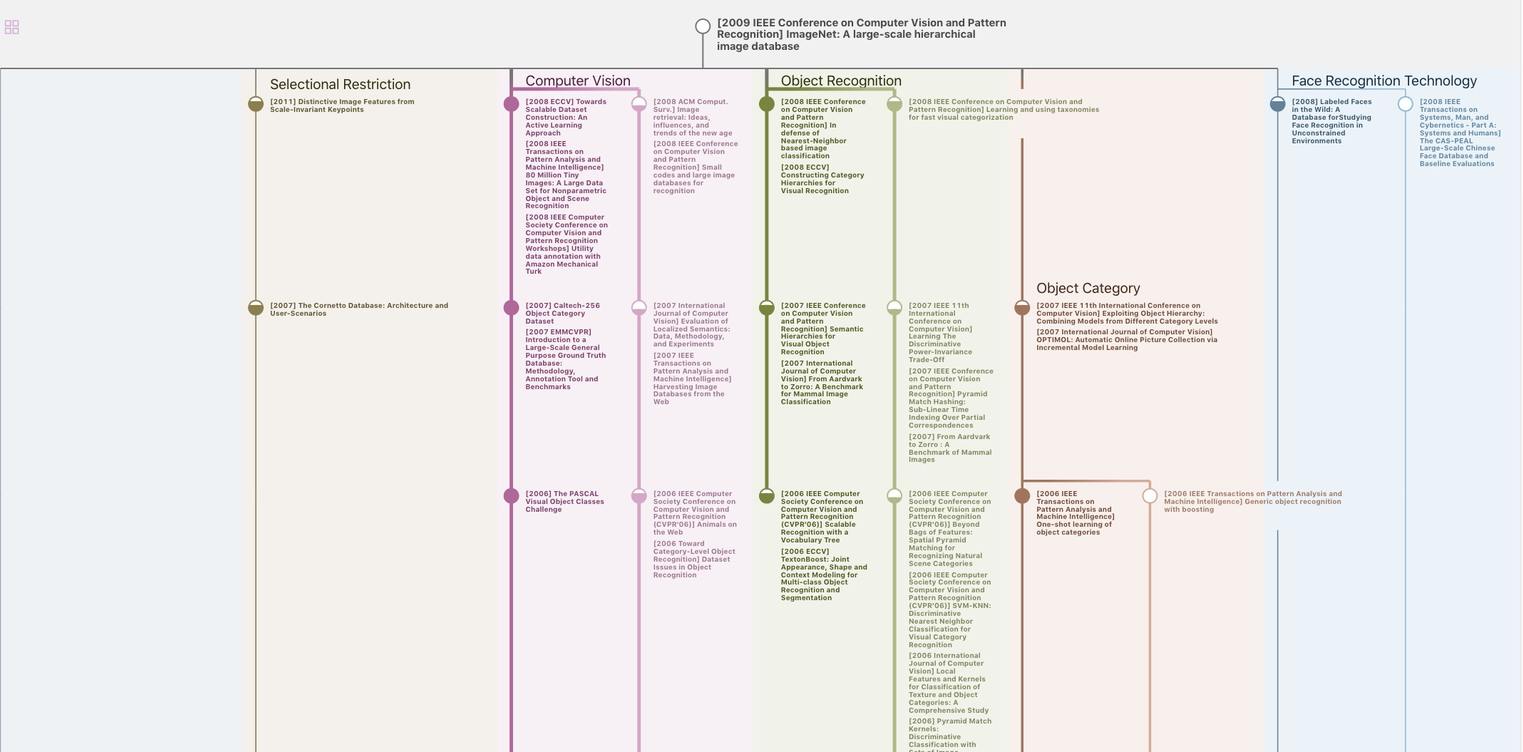
生成溯源树,研究论文发展脉络
Chat Paper
正在生成论文摘要