Complex graph convolutional network for link prediction in knowledge graphs
Expert Systems with Applications(2022)
摘要
Knowledge graph (KG) embedding models map nodes and edges to fixed-length vectors and obtain the similarity of nodes as the output of a scoring function to predict missing links between nodes. KG embedding methods based on graph convolutional networks (GCNs) have recently gained significant attention due to their ability to add information of neighboring nodes into the nodes’ embeddings. However, existing GCNs are primarily based on real-valued embeddings, which have high distortion, particularly when modeling graphs with varying geometric structures. In this paper, we propose complex graph convolutional network (ComplexGCN), a novel extension of the standard GCNs in complex space to combine the expressiveness of complex geometry with GCNs for improving the representation quality of KG components. The proposed ComplexGCN comprises a set of complex graph convolutional layers and a complex scoring function based on PARATUCK2 decomposition: the former includes information of neighboring nodes into the nodes’ embeddings, while the latter leverages these embeddings to predict new links between nodes. The proposed model demonstrates enhanced performance compared to existing methods on the two recent standard link prediction datasets.
更多查看译文
关键词
Knowledge graph,Link prediction,Graph convolutional network,Complex embeddings,Tensor decomposition
AI 理解论文
溯源树
样例
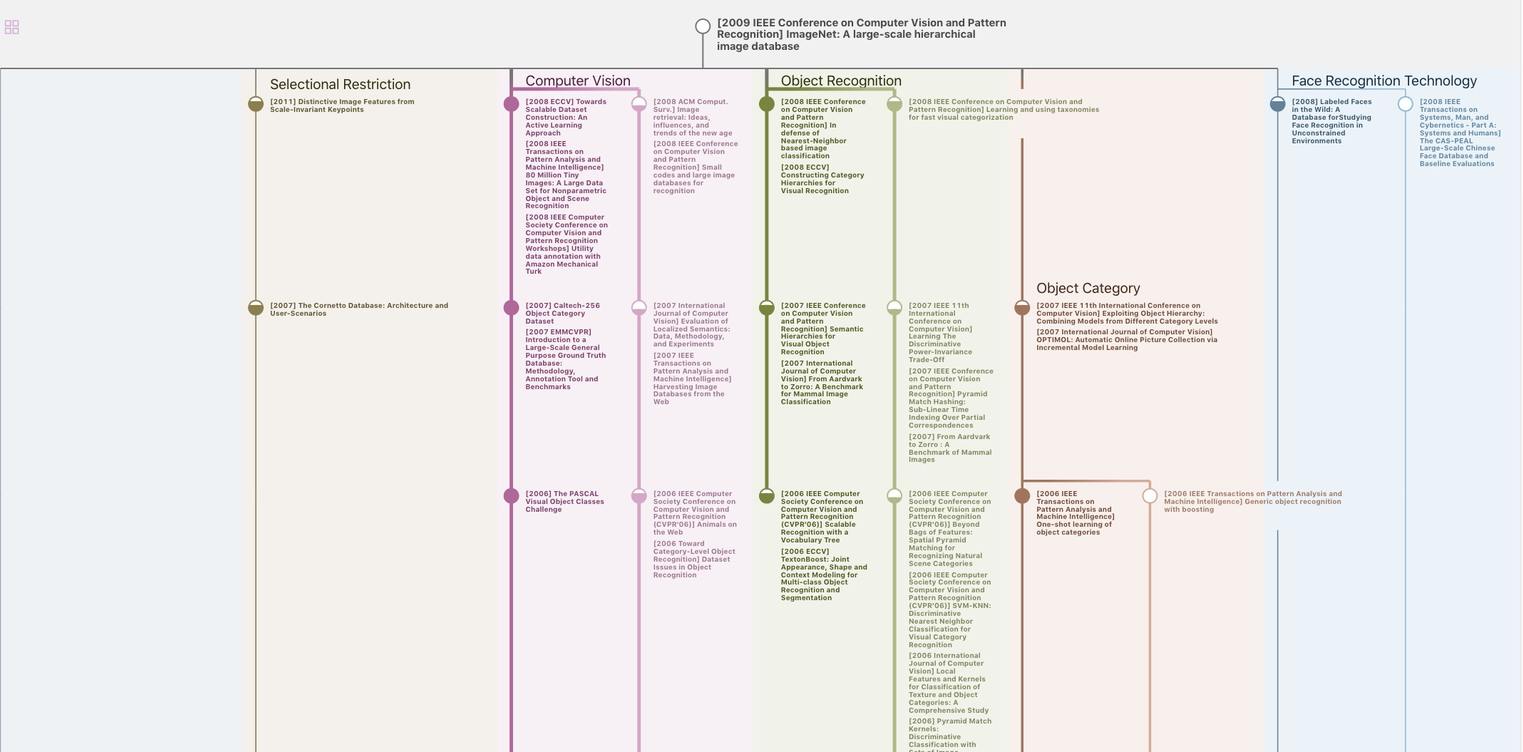
生成溯源树,研究论文发展脉络
Chat Paper
正在生成论文摘要